Structured Uncertainty in the Observation Space of Variational Autoencoders
arxiv(2022)
摘要
Variational autoencoders (VAEs) are a popular class of deep generative models with many variants and a wide range of applications. Improvements upon the standard VAE mostly focus on the modelling of the posterior distribution over the latent space and the properties of the neural network decoder. In contrast, improving the model for the observational distribution is rarely considered and typically defaults to a pixel-wise independent categorical or normal distribution. In image synthesis, sampling from such distributions produces spatially-incoherent results with uncorrelated pixel noise, resulting in only the sample mean being somewhat useful as an output prediction. In this paper, we aim to stay true to VAE theory by improving the samples from the observational distribution. We propose SOS-VAE, an alternative model for the observation space, encoding spatial dependencies via a low-rank parameterisation. We demonstrate that this new observational distribution has the ability to capture relevant covariance between pixels, resulting in spatially-coherent samples. In contrast to pixel-wise independent distributions, our samples seem to contain semantically-meaningful variations from the mean allowing the prediction of multiple plausible outputs with a single forward pass.
更多查看译文
关键词
structured uncertainty,variational
AI 理解论文
溯源树
样例
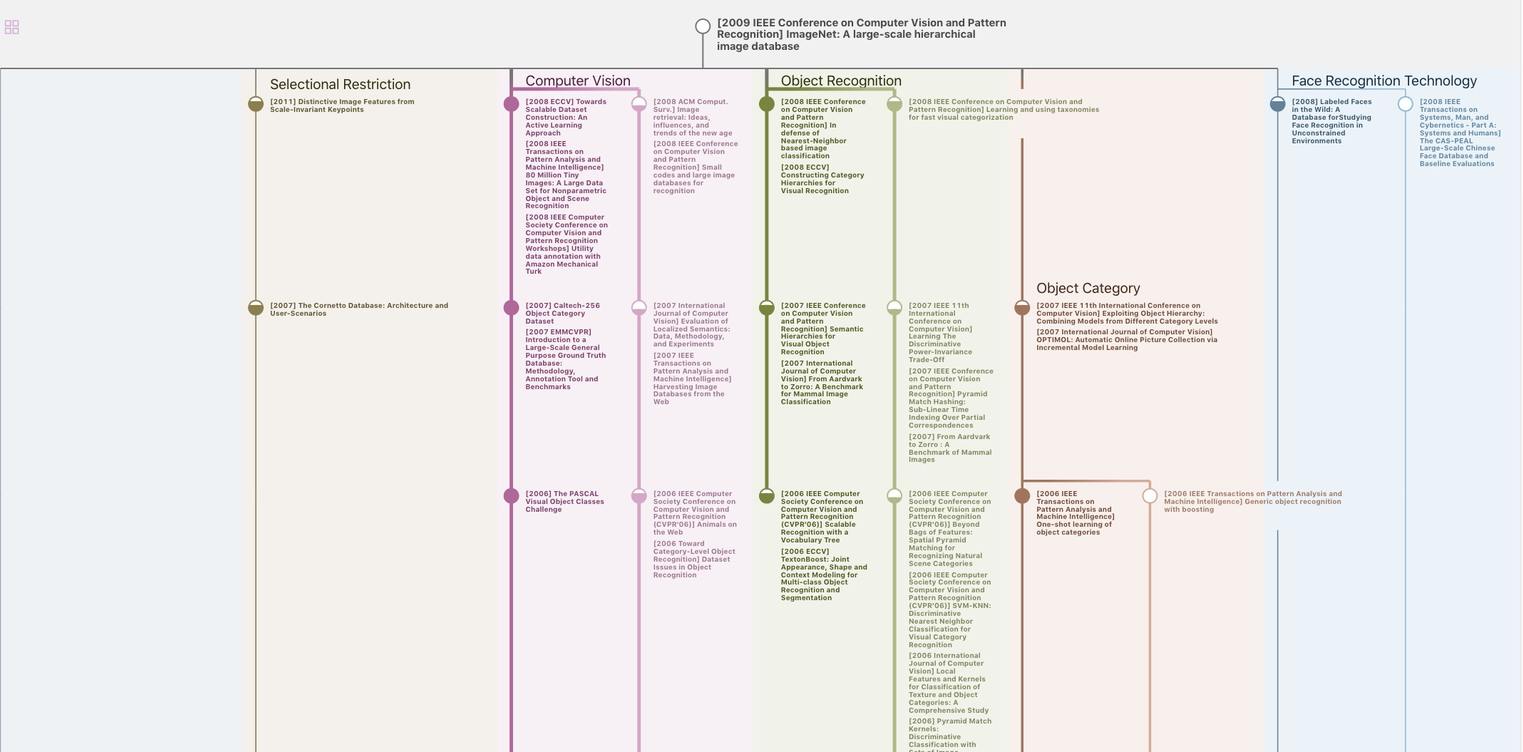
生成溯源树,研究论文发展脉络
Chat Paper
正在生成论文摘要