Sparse*BERT: Sparse Models Generalize To New tasks and Domains
arxiv(2023)
摘要
Large Language Models have become the core architecture upon which most modern natural language processing (NLP) systems build. These models can consistently deliver impressive accuracy and robustness across tasks and domains, but their high computational overhead can make inference difficult and expensive. To make using these models less costly, recent work has explored leveraging structured and unstructured pruning, quantization, and distillation to improve inference speed and decrease size. This paper studies how models pruned using Gradual Unstructured Magnitude Pruning can transfer between domains and tasks. Our experimentation shows that models that are pruned during pretraining using general domain masked language models can transfer to novel domains and tasks without extensive hyperparameter exploration or specialized approaches. We demonstrate that our general sparse model Sparse*BERT can become SparseBioBERT simply by pretraining the compressed architecture on unstructured biomedical text. Moreover, we show that SparseBioBERT can match the quality of BioBERT with only 10\% of the parameters.
更多查看译文
关键词
sparse*bert models generalize,new tasks
AI 理解论文
溯源树
样例
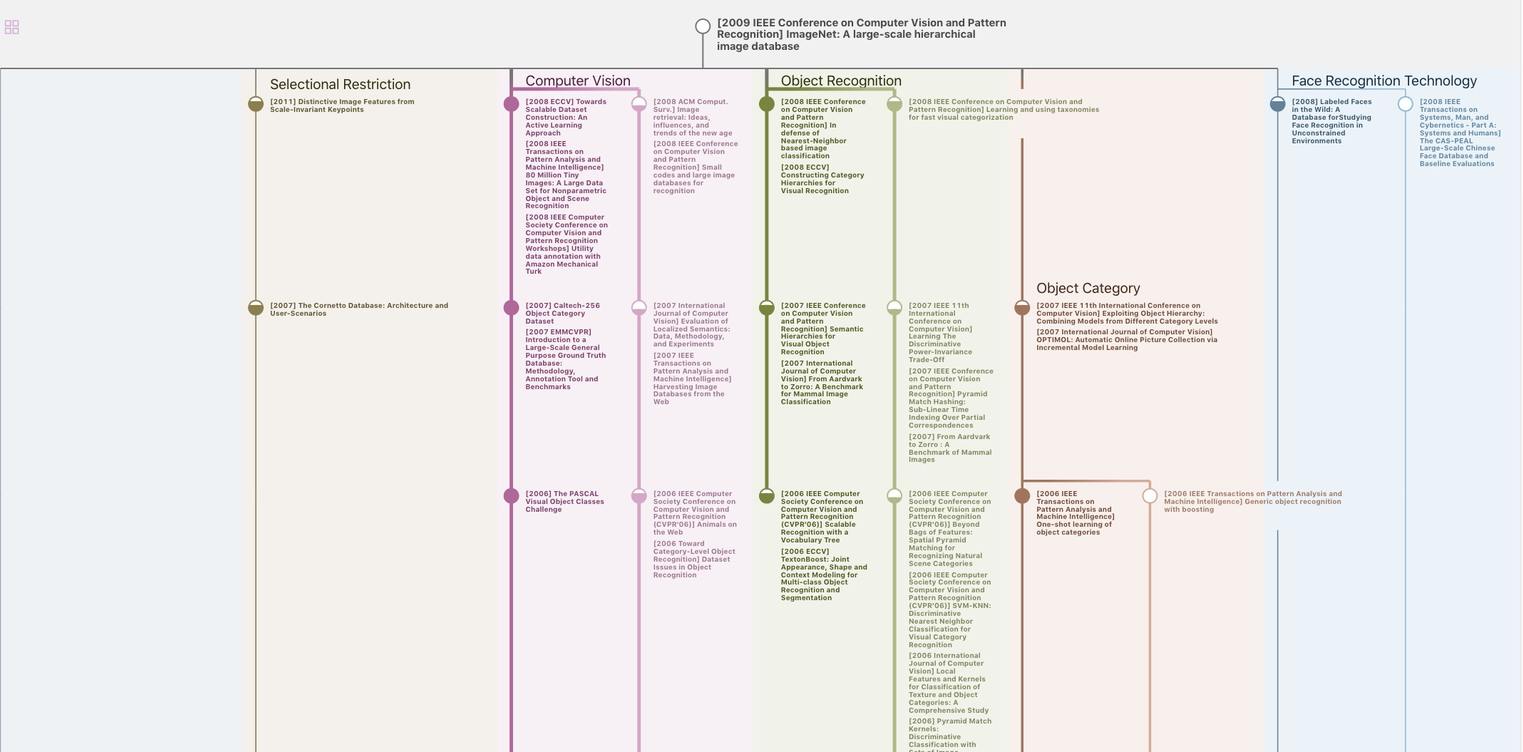
生成溯源树,研究论文发展脉络
Chat Paper
正在生成论文摘要