Fast Multiview Clustering With Spectral Embedding
IEEE TRANSACTIONS ON IMAGE PROCESSING(2022)
摘要
Spectral clustering has been a hot topic in unsupervised learning owing to its remarkable clustering effectiveness and well-defined framework. Despite this, due to its high computation complexity, it is unable of handling large-scale or high-dimensional data, particularly multi-view large-scale data. To address this issue, in this paper, we propose a fast multi-view clustering algorithm with spectral embedding (FMCSE), which speeds up both the spectral embedding and spectral analysis stages of multi-view spectral clustering. Furthermore, unlike conventional spectral clustering, FMCSE can acquire all sample categories directly after optimization without extra k-means, which can significantly enhance efficiency. Moreover, we also provide a fast optimization strategy for solving the FMCSE model, which divides the optimization problem into three decoupled small-scale sub-problems that can be solved in a few iteration steps. Finally, extensive experiments on a variety of real-world datasets (including large-scale and high-dimensional datasets) show that, when compared to other state-of-the-art fast multi-view clustering baselines, FMCSE can maintain comparable or even better clustering effectiveness while significantly improving clustering efficiency.
更多查看译文
关键词
Spectral analysis, Optimization, Complexity theory, Clustering methods, Clustering algorithms, Linear programming, Task analysis, Multi-view clustering, spectral embedding, anchor graph, orthogonality
AI 理解论文
溯源树
样例
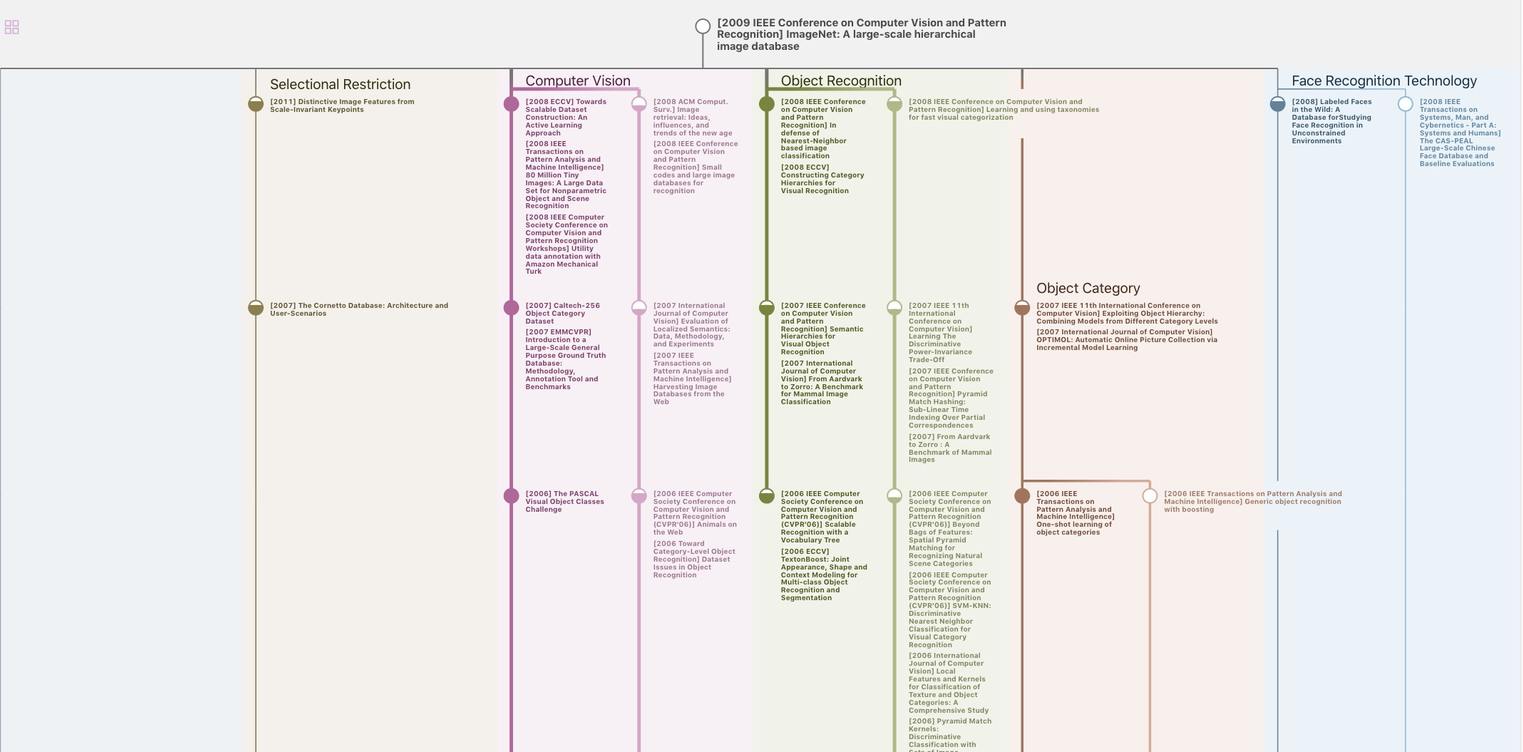
生成溯源树,研究论文发展脉络
Chat Paper
正在生成论文摘要