Sparse and Simple Structure Estimation via Prenet Penalization
Psychometrika(2022)
摘要
We propose a prenet ( pr oduct-based e lastic net ), a novel penalization method for factor analysis models. The penalty is based on the product of a pair of elements in each row of the loading matrix. The prenet not only shrinks some of the factor loadings toward exactly zero but also enhances the simplicity of the loading matrix, which plays an important role in the interpretation of the common factors. In particular, with a large amount of prenet penalization, the estimated loading matrix possesses a perfect simple structure, which is known as a desirable structure in terms of the simplicity of the loading matrix. Furthermore, the perfect simple structure estimation via the proposed penalization turns out to be a generalization of the k -means clustering of variables. On the other hand, a mild amount of the penalization approximates a loading matrix estimated by the quartimin rotation, one of the most commonly used oblique rotation techniques. Simulation studies compare the performance of our proposed penalization with that of existing methods under a variety of settings. The usefulness of the perfect simple structure estimation via our proposed procedure is presented through various real data applications.
更多查看译文
关键词
multivariate analysis,penalized maximum likelihood estimation,perfect simple structure,quartimin rotation,sparse estimation
AI 理解论文
溯源树
样例
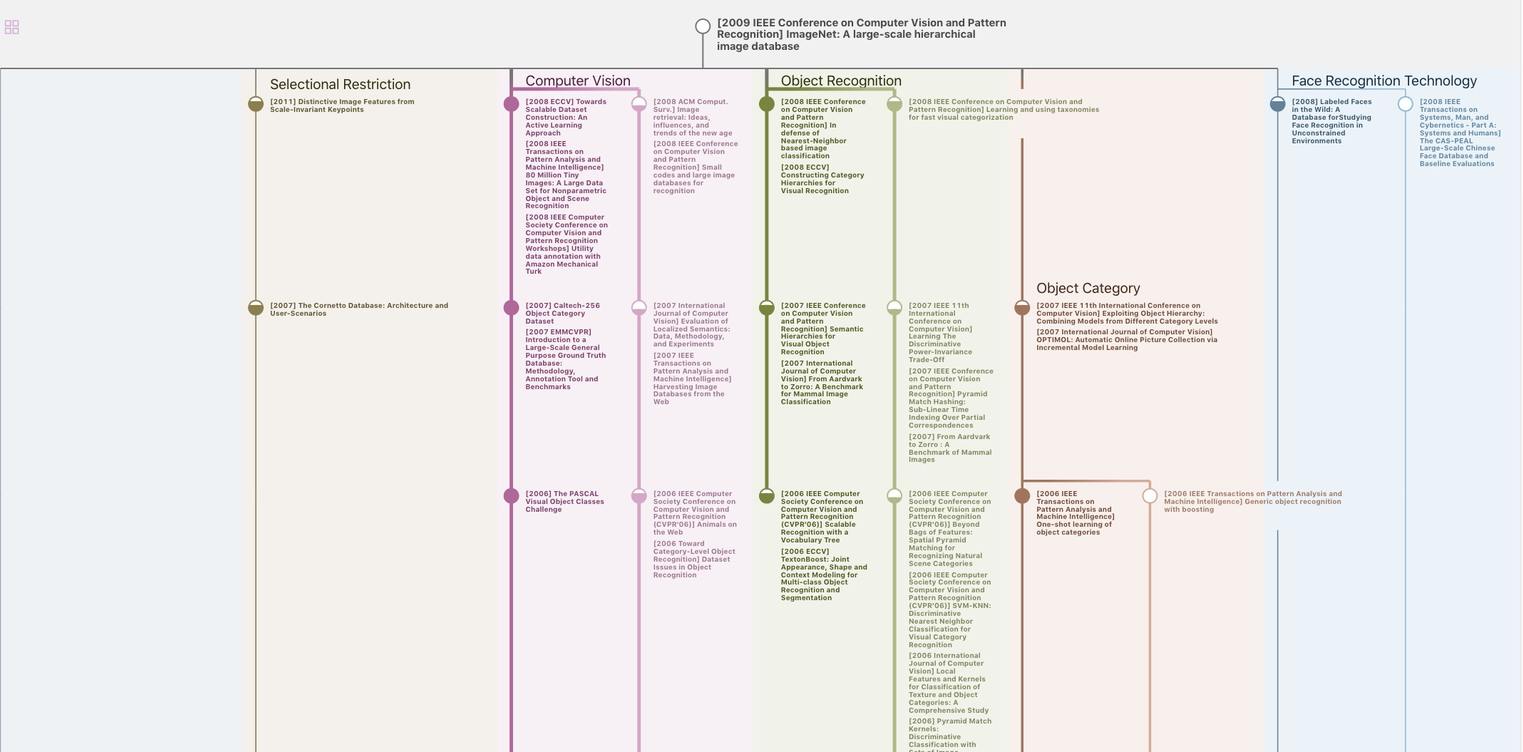
生成溯源树,研究论文发展脉络
Chat Paper
正在生成论文摘要