Unsupervised Learning of Hierarchical Conversation Structure
arXiv (Cornell University)(2022)
摘要
Human conversations can evolve in many different ways, creating challenges for automatic understanding and summarization. Goal-oriented conversations often have meaningful sub-dialogue structure, but it can be highly domain-dependent. This work introduces an unsupervised approach to learning hierarchical conversation structure, including turn and sub-dialogue segment labels, corresponding roughly to dialogue acts and sub-tasks, respectively. The decoded structure is shown to be useful in enhancing neural models of language for three conversation-level understanding tasks. Further, the learned finite-state sub-dialogue network is made interpretable through automatic summarization.
更多查看译文
关键词
hierarchical conversation structure,learning
AI 理解论文
溯源树
样例
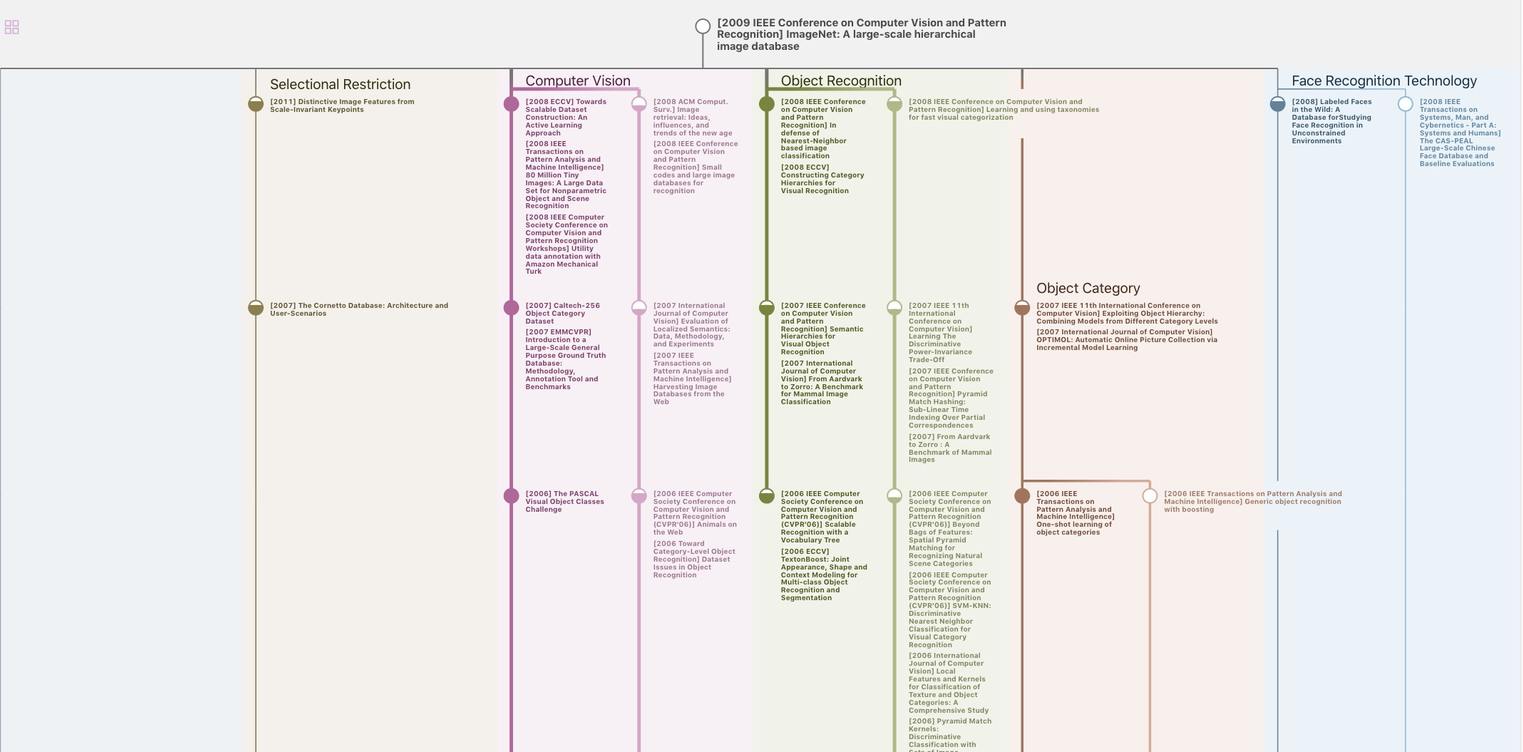
生成溯源树,研究论文发展脉络
Chat Paper
正在生成论文摘要