Enhancing Continual Learning with Global Prototypes: Counteracting Negative Representation Drift
arxiv(2023)
摘要
Continual learning (CL) aims to learn a sequence of tasks over time, with data distributions shifting from one task to another. When training on new task data, data representations from old tasks may drift. Some negative representation drift can result in catastrophic forgetting, by causing the locally learned class prototypes and data representations to correlate poorly across tasks. To mitigate such representation drift, we propose a method that finds global prototypes to guide the learning, and learns data representations with the regularization of the self-supervised information. Specifically, for NLP tasks, we formulate each task in a masked language modeling style, and learn the task via a neighbor attention mechanism over a pre-trained language model. Experimental results show that our proposed method can learn fairly consistent representations with less representation drift, and significantly reduce catastrophic forgetting in CL without resampling data from past tasks.
更多查看译文
关键词
representations,learning,sentence,task-related
AI 理解论文
溯源树
样例
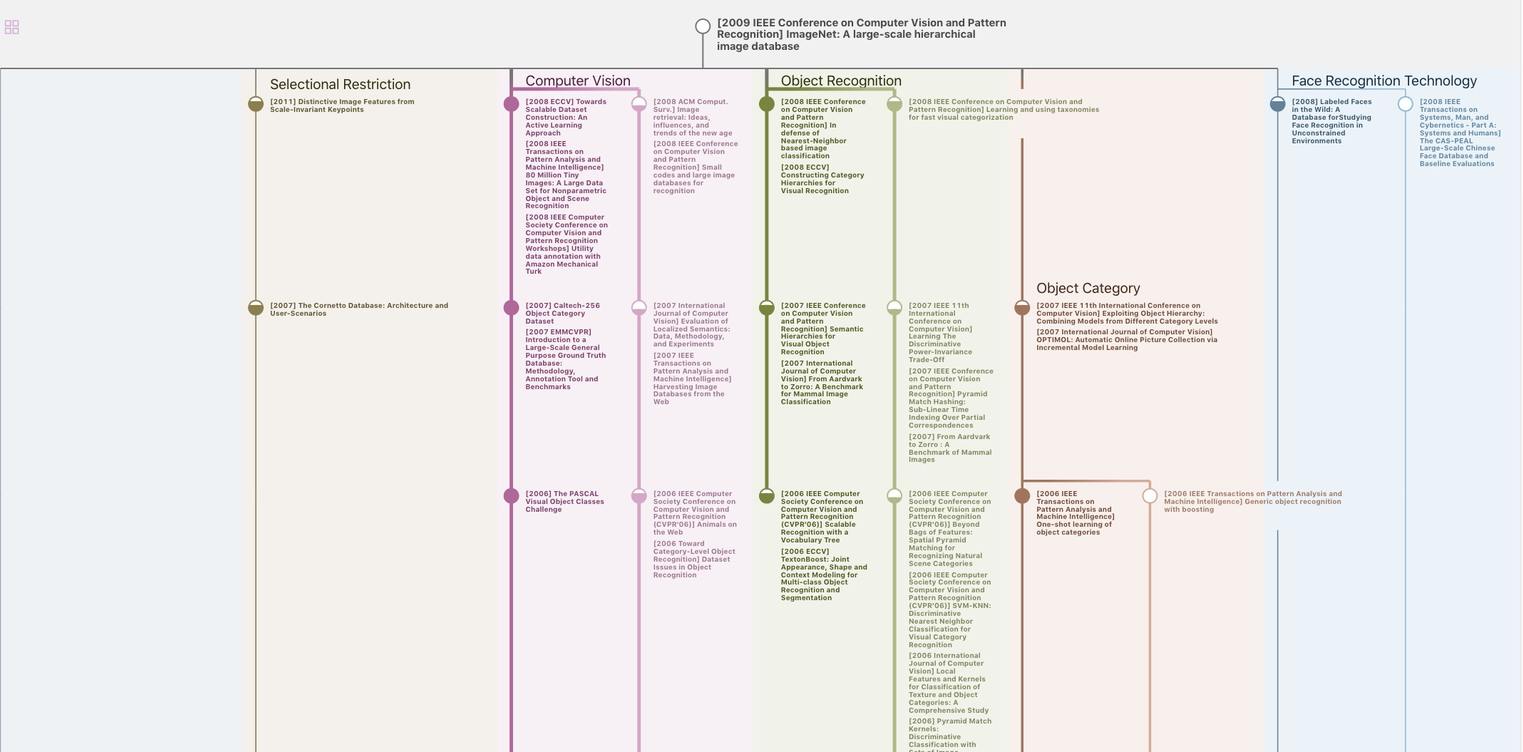
生成溯源树,研究论文发展脉络
Chat Paper
正在生成论文摘要