Neural Contextual Bandits Based Dynamic Sensor Selection for Low-Power Body-Area Networks
International Symposium on Low Power Electronics and Design(2022)
摘要
BSTRACTProviding health monitoring devices with machine intelligence is important for enabling automatic mobile healthcare applications. However, this brings additional challenges due to the resource scarcity of these devices. This work introduces a neural contextual bandits based dynamic sensor selection methodology for high-performance and resource-efficient body-area networks to realize next generation mobile health monitoring devices. The methodology utilizes contextual bandits to select the most informative sensor combinations during runtime and ignore redundant data for decreasing transmission and computing power in a body area network (BAN). The proposed method has been validated using one of the most common health monitoring applications: cardiac activity monitoring. Solutions from our proposed method are compared against those from related works in terms of classification performance and energy while considering the communication energy consumption. Our final solutions could reach 78.8% AU-PRC on the PTB-XL ECG dataset for cardiac abnormality detection while decreasing the overall energy consumption and computational energy by 3.7 × and 4.3 ×, respectively.
更多查看译文
关键词
dynamic sensor selection,neural contextual bandits based,low-power,body-area
AI 理解论文
溯源树
样例
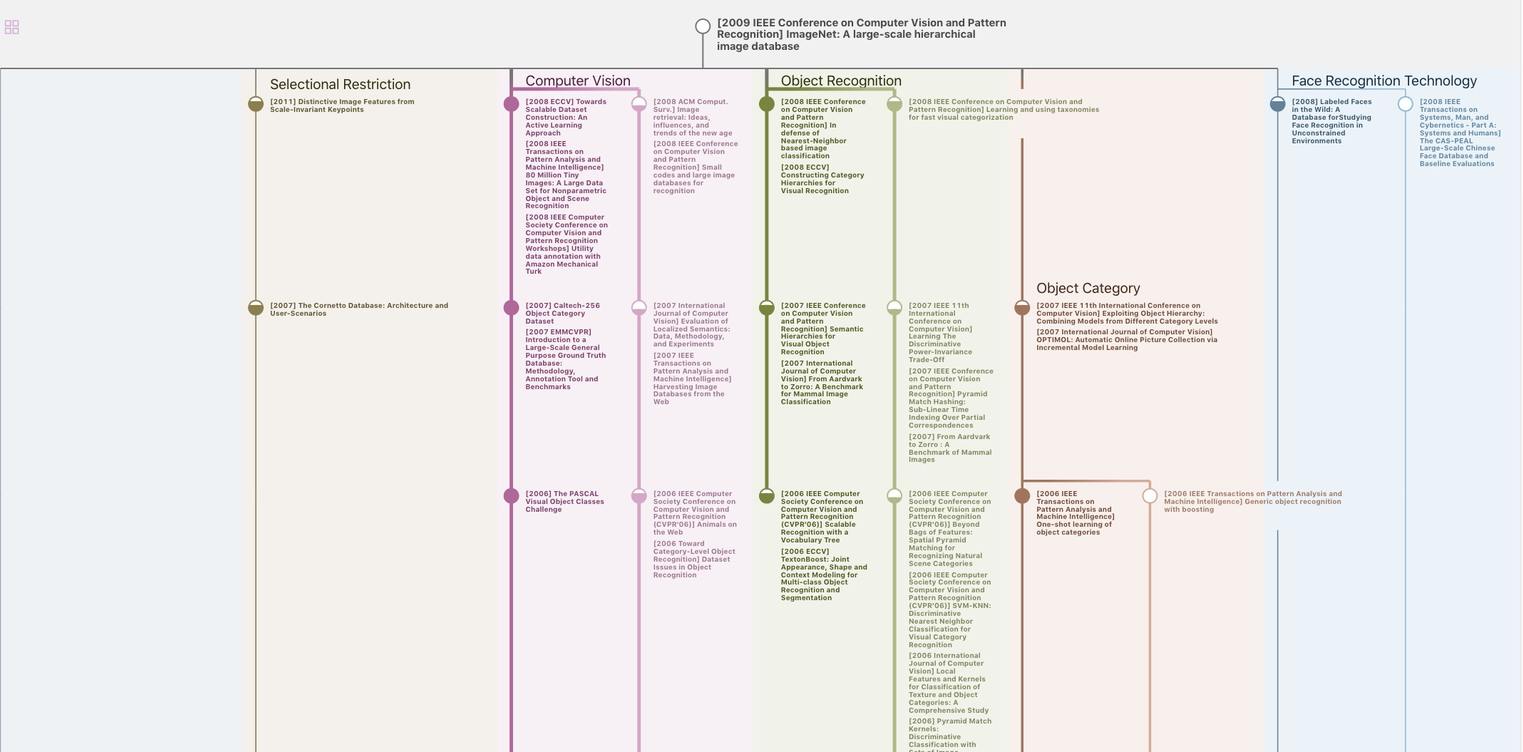
生成溯源树,研究论文发展脉络
Chat Paper
正在生成论文摘要