DOGE-Train: Discrete Optimization on GPU with End-to-End Training
AAAI 2024(2024)
摘要
We present a fast, scalable, data-driven approach for solving relaxations of 0-1 integer linear programs. We use a combination of graph neural networks (GNN) and a Lagrange decomposition based algorithm. We make the latter differentiable for end-to-end training and use GNNs to predict its algorithmic parameters. This allows to retain the algorithm's theoretical properties including dual feasibility and guaranteed non-decrease in the lower bound while improving it via training. We overcome suboptimal fixed points of the basic solver by additional non-parametric GNN update steps maintaining dual feasibility. For training we use an unsupervised loss. We train on smaller problems and test on larger ones showing strong generalization performance with a GNN comprising only around 10k parameters. Our solver achieves significantly faster performance and better dual objectives than its non-learned version, achieving close to optimal objective values of LP relaxations of very large structured prediction problems and on selected combinatorial ones. In particular, we achieve better objective values than specialized approximate solvers for specific problem classes while retaining their efficiency. Our solver has better any-time performance over a large time period compared to a commercial solver.
更多查看译文
关键词
SO: Combinatorial Optimization,CSO: Mixed Discrete/Continuous Optimization,SO: Algorithm Configuration,SO: Learning to Search
AI 理解论文
溯源树
样例
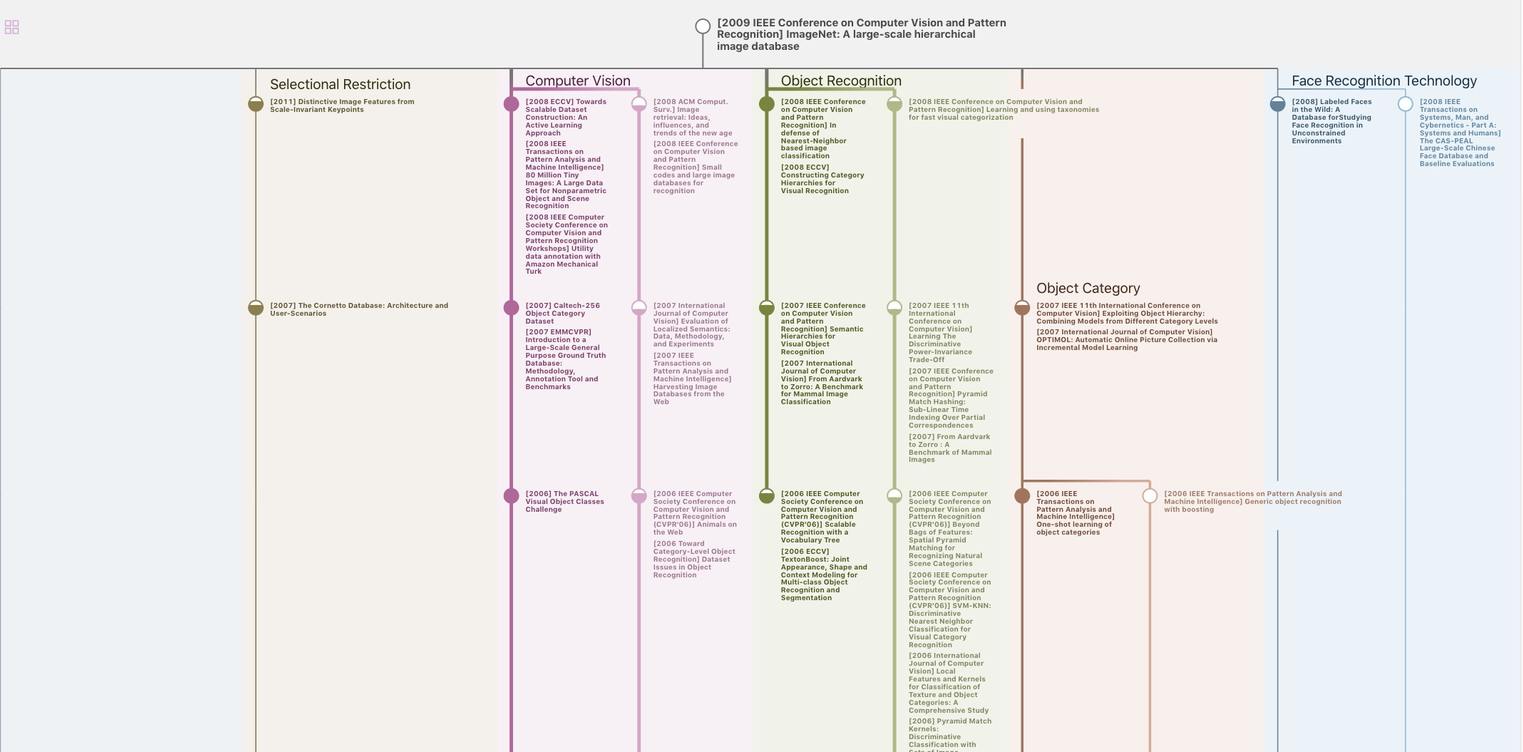
生成溯源树,研究论文发展脉络
Chat Paper
正在生成论文摘要