Online Debiasing for Adaptively Collected High-Dimensional Data With Applications to Time Series Analysis
JOURNAL OF THE AMERICAN STATISTICAL ASSOCIATION(2023)
摘要
Adaptive collection of data is commonplace in applications throughout science and engineering. From the point of view of statistical inference, however, adaptive data collection induces memory and correlation in the samples, and poses significant challenge. We consider the high-dimensional linear regression, where the samples are collected adaptively, and the sample size n can be smaller than p, the number of covariates. In this setting, there are two distinct sources of bias: the first due to regularization imposed for consistent estimation, for example, using the LASSO, and the second due to adaptivity in collecting the samples. We propose "online debiasing," a general procedure for estimators such as the LASSO, which addresses both sources of bias. In two concrete contexts (i) time series analysis and (ii) batched data collection, we demonstrate that online debiasing optimally debiases the LASSO estimate when the underlying parameter theta(0) has sparsity of order o(root n/log p). In this regime, the debiased estimator can be used to compute p-values and confidence intervals of optimal size.
更多查看译文
关键词
Bias,Confidence intervals,Hypothesis testing,Lasso
AI 理解论文
溯源树
样例
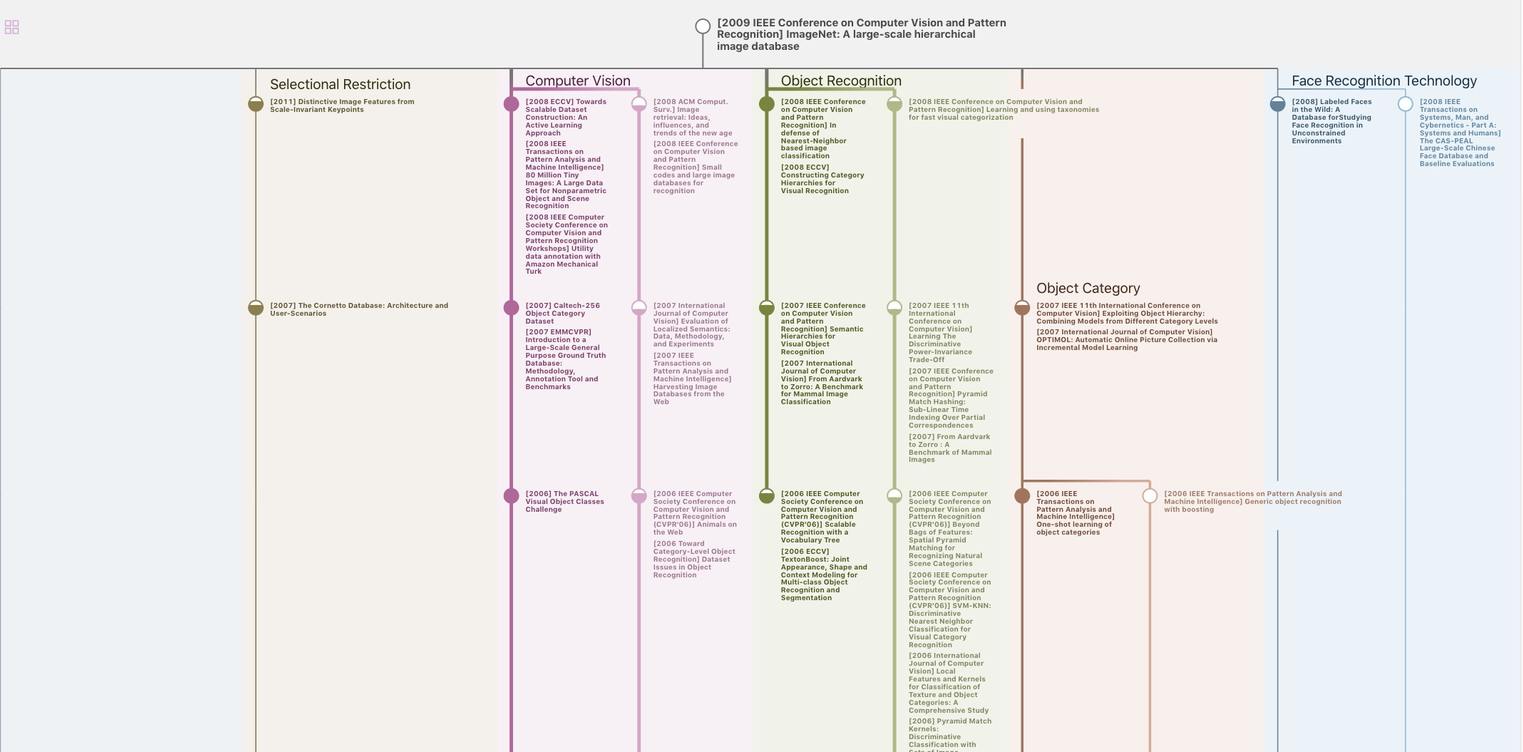
生成溯源树,研究论文发展脉络
Chat Paper
正在生成论文摘要