Reinforcement Learning-Based Flow Management Techniques for Urban Air Mobility and Dense Low-Altitude Air Traffic Operations
2021 IEEE/AIAA 40TH DIGITAL AVIONICS SYSTEMS CONFERENCE (DASC)(2021)
摘要
As Unmanned Aircraft Systems (UAS) technology matures, and the demand for UAS commercial operations is gradually increasing, a widespread proliferation of UAS operations may lead saturation of the airspace resources. Such congestion instances would increase the time-criticality of UAS Traffic Management (UTM) interventions and likely reduce operational efficiency and safety. Therefore, innovative tools and services are needed to deliver Demand and Capacity Balancing (DCB) services in a range of airspace regions, thus increasing operational efficiency and safety while also reducing the time-criticality of UTM operator's duties. The research presented in this paper aims to develop an efficient and uncertainty-resilient DCB process and solution framework based on hybrid learning algorithms, which allows UTM systems to satisfy the operational requirements of UAS in dense metropolitan regions. The focus of this particular paper is on the analysis of uncertainty factors affecting UAS trajectory conformance in the urban and suburban low-altitude airspace and on the requirements which these factors pose on the determination of recommended DCB processes and techniques. Capitalising on these findings, this research will try to improve the safety, efficiency and uncertainty-resilience of UAS traffic in low-altitude urban airspace operations.
更多查看译文
关键词
Reinforcement Learning, Genetic Algorithm, Traffic Flow Management, Low-Altitude Airspace Operations, UAS Air Mobility, Demand Capacity Balancing
AI 理解论文
溯源树
样例
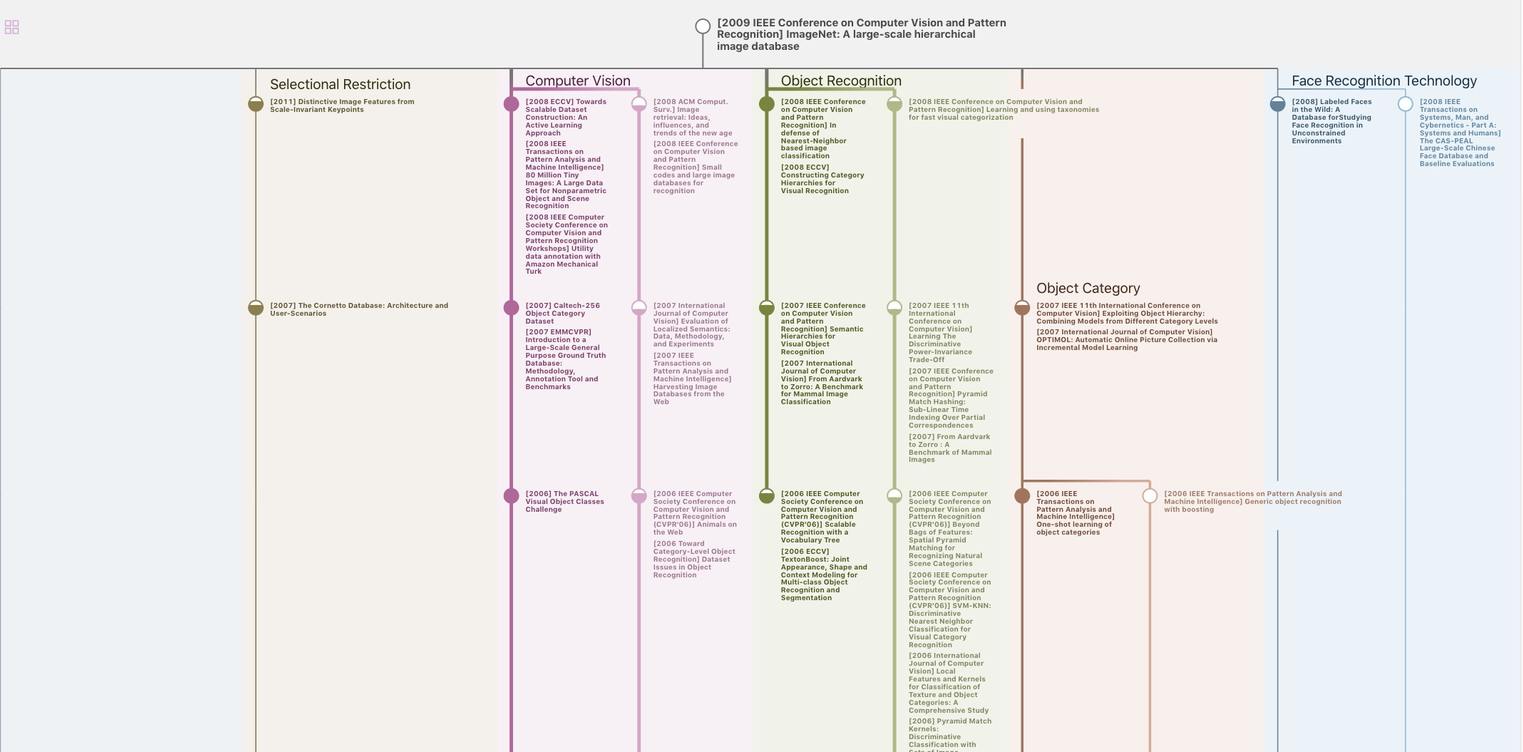
生成溯源树,研究论文发展脉络
Chat Paper
正在生成论文摘要