A Multi-Agent Reinforcement Learning Approach for Conflict Resolution in Dense Traffic Scenarios
2021 IEEE/AIAA 40TH DIGITAL AVIONICS SYSTEMS CONFERENCE (DASC)(2021)
摘要
A multi-agent reinforcement learning (MARL) based conflict resolution method is proposed. The motivation is to reduce the workloads of air traffic controllers (ATCOs) and pilots in operation over the dense airspace. First, a intermediate waypoints generation method is presented to avoid the frequent fine-tuning in the resolution process. This method enables the controllers and pilots to resolve conflicts in one-step decision making. Next, the multi-agent reinforcement learning method is used to search for the optimal intermediate waypoints. Several numerical examples are presented to illustrate the proposed methodology. A detailed discussion of the sample efficiency with respect to various number of agents is given. Both the benchmark and practical examples are used for validation. The proposed method is able to handle the mulit-conflict scenarios without recourse to frequent disturbance of the pilots and controllers.
更多查看译文
关键词
reinforcement learning, conflict detection and resolution, multi-agent deep deterministic policy gradient, air traffic control
AI 理解论文
溯源树
样例
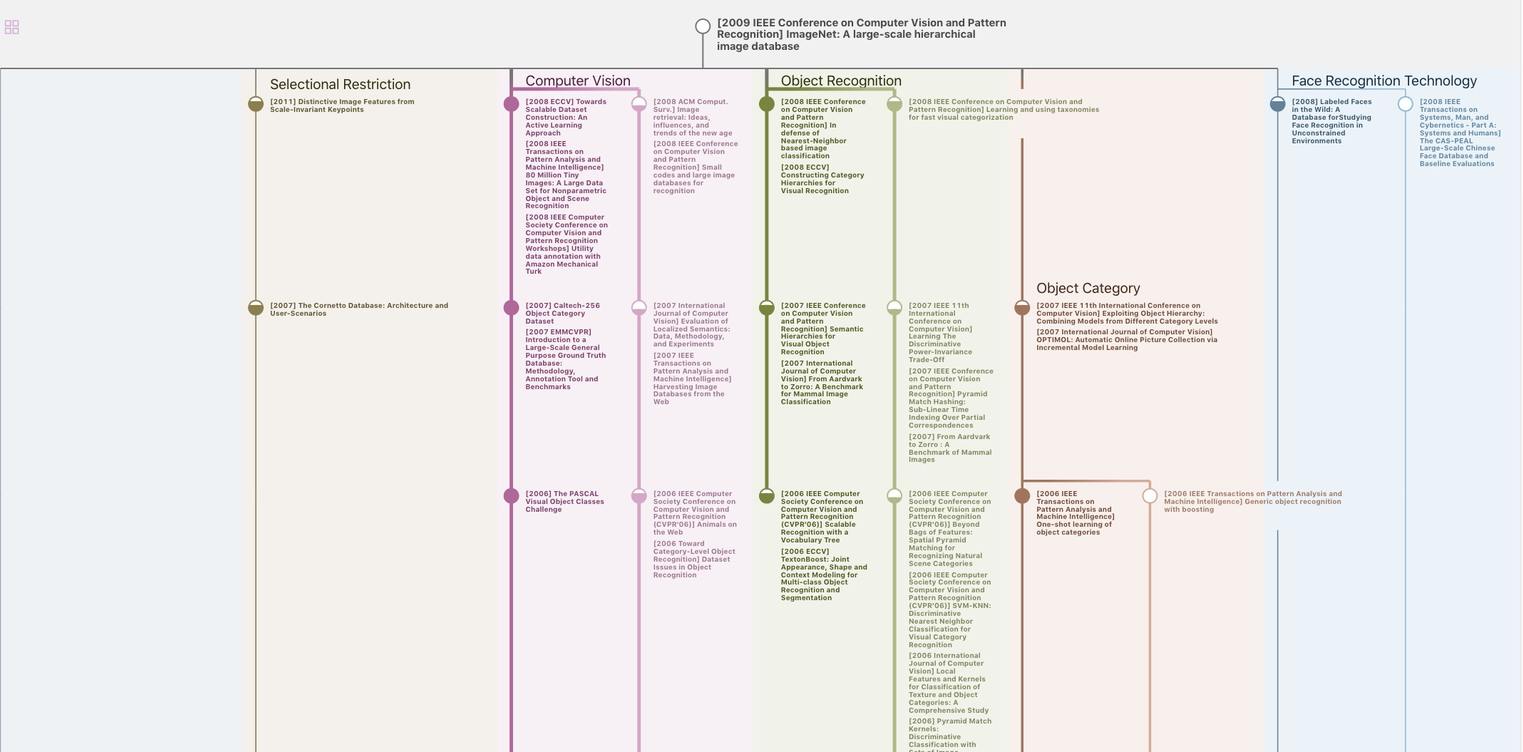
生成溯源树,研究论文发展脉络
Chat Paper
正在生成论文摘要