META-LEARNING OF RBF NETWORKS IN SEQUENTIAL APPROXIMATE OPTIMIZATION
JOURNAL OF NONLINEAR AND CONVEX ANALYSIS(2021)
摘要
It is not desirable to apply traditional optimization techniques for many real-world design problems that the number of objective function evaluations is severely limited because its evaluation requires considerably computational time or cost. For the sake of optimization involving such expensive function, approximate functions are used as surrogate, and are constructed based on sampled points by using machine learning methods such as radial basis function networks and support vector machines. In this research, we suggest meta-learning of RBF networks for building good approximate functions as well as mitigating the burden of function evaluations to obtain a good solution. The performance of RBF networks is closely associated with a basis function and its hyper-parameter. Considering the distance and density of sampled points, we present a meta-learning method for choosing hyper-parameters of the center and the radius of Gaussian basis function in RBF networks. The effectiveness and performance of the proposed method are investigated in terms of the accuracy for obtained solutions and the number of function evaluations through some numerical examples.
更多查看译文
关键词
Meta-learning, Gaussian radial basis function networks, sequential approximate optimization
AI 理解论文
溯源树
样例
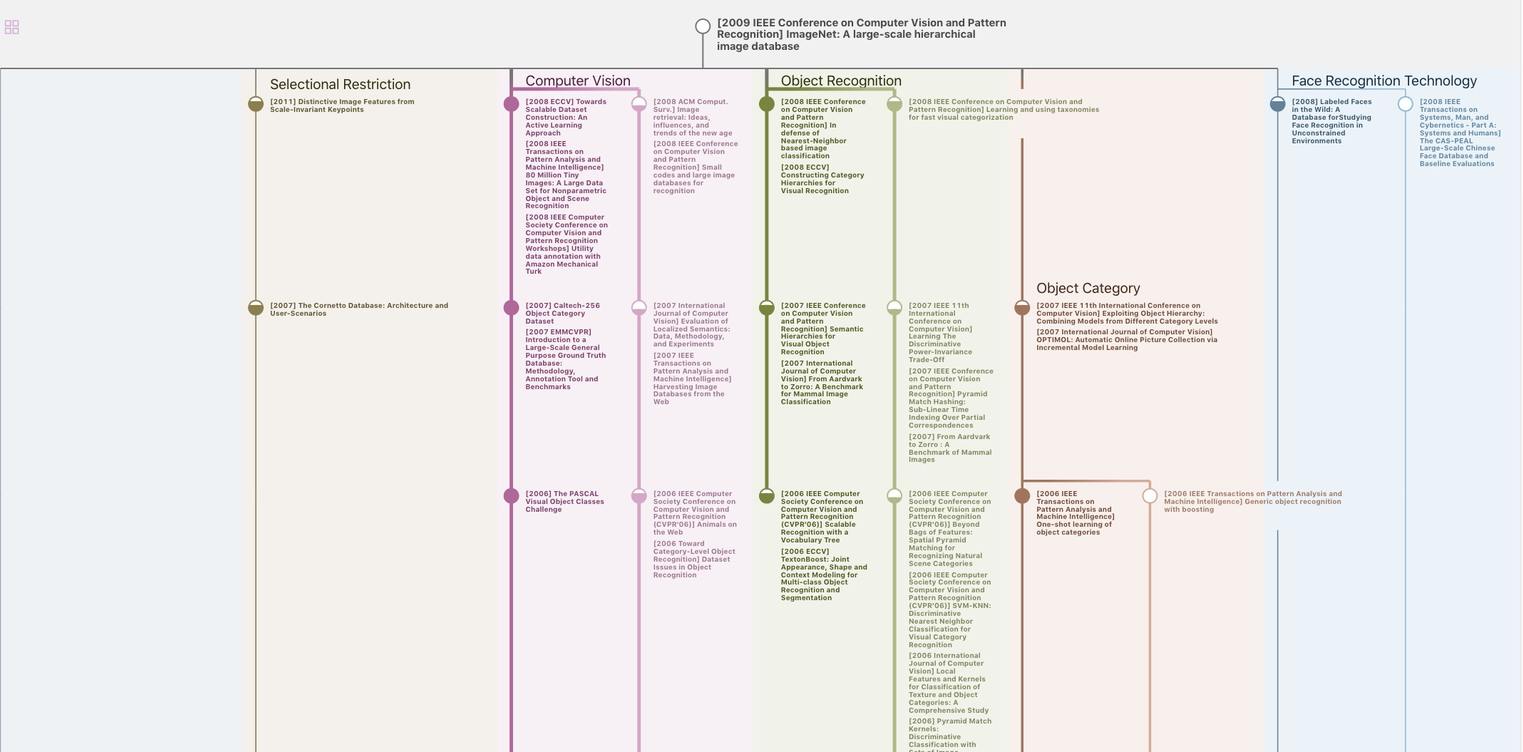
生成溯源树,研究论文发展脉络
Chat Paper
正在生成论文摘要