OPTIMIZED GRADIENT DESCENT AND PRE-FILLED FOR SVD RECOMMENDATION ALGORITHM
JOURNAL OF NONLINEAR AND CONVEX ANALYSIS(2021)
摘要
Matrix Factorization for collaborative filtering approach has important theoretical significance and application value in large-scale systems and practical applications. In this paper, the traditional Singular value decomposition (SVD) collaborative filter recommendation algorithm is sensitive to the sparseness of the data, the accuracy is not high, and the number of iterations is too long. This paper proposes a gradient descent matrix decomposition cooperation based on SVD Filter recommendation algorithm. Firstly,this algorithm proposes the row mean, column mean, and global mean pre-filling method to pre-fill the original scoring matrix, and then performs SVD decomposition to reduce the impact of data sparsity on the recommendation accuracy. Second, the matrix after SVD decomposition the relationship with the original score is used to remodel the prediction score to improve the accuracy of the final prediction score. Finally,an optimized gradient descent(OGD) method is proposed. Specifically,the pre-filled improved SVD decomposition matrix is used as the initial value of gradient descent to perform gradient descent, thereby reducing the number of iterations of gradient descent. Compared with the traditional SVD-based gradient descent matrix factorization collaborative filtering recommendation algorithm, the proposed algorithm can effectively recommend accuracy and speed up the prediction score,data sparsity.
更多查看译文
关键词
Collaborative filtering recommendation algorithm, singular value decomposition, gradient descent, pre-fill
AI 理解论文
溯源树
样例
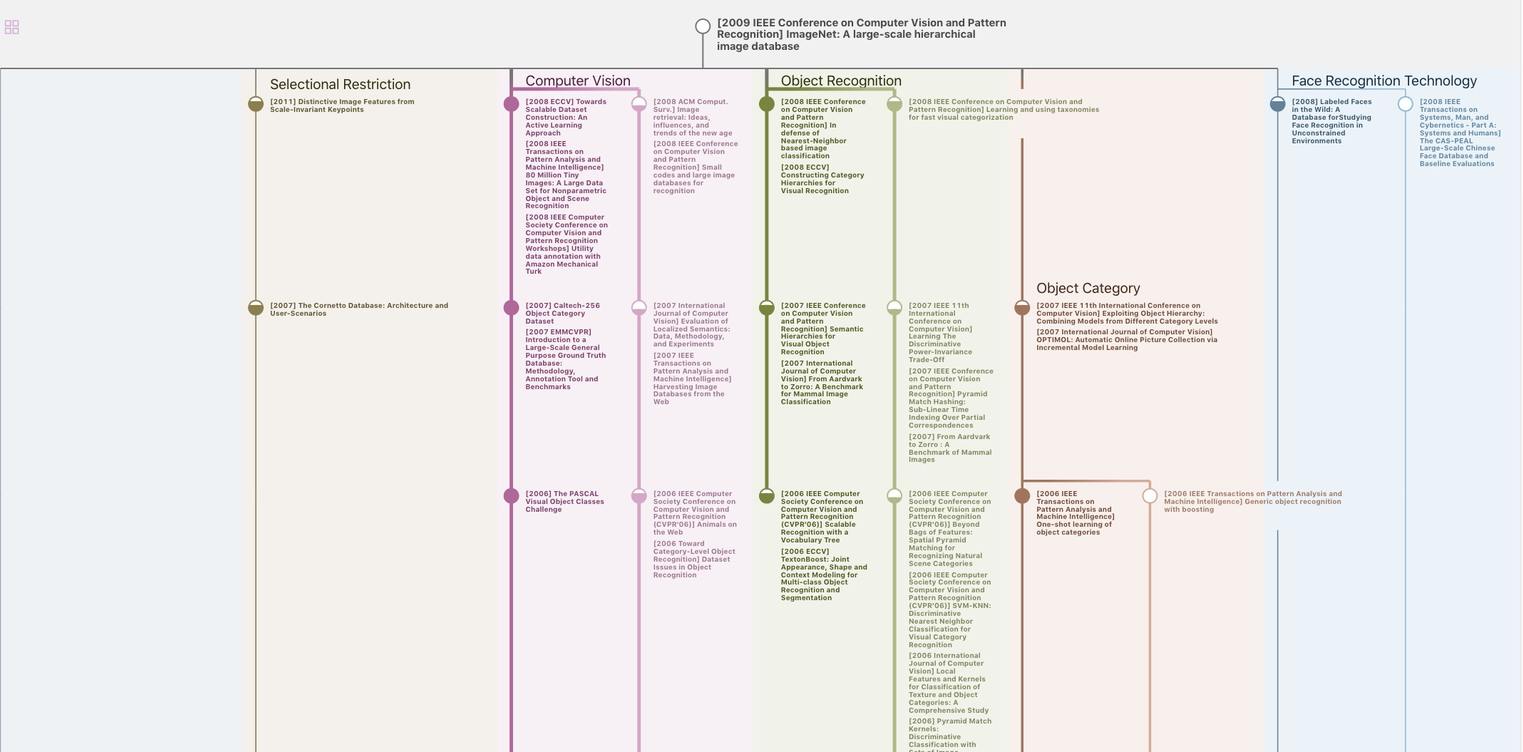
生成溯源树,研究论文发展脉络
Chat Paper
正在生成论文摘要