POST-PROCESSING AND DIMENSIONALITY REDUCTION FOR EXTREME LEARNING MACHINE IN TEXT CLASSIFICATION
ECONOMIC COMPUTATION AND ECONOMIC CYBERNETICS STUDIES AND RESEARCH(2021)
摘要
Text classification is one of the core technologies of textual analysis, with interesting applications varying from sentiment classification, language identification to online abuse detection and many more. Many approaches have been taken to the machine learning classifiers employed in text categorization, with a focus on boosting model performance and efficiency. This paper proposes a new effective framework for the input processing of the Extreme Learning Machine (EML) algorithm, illustrated on the Reuters-21578 test collection of documents. We employ Glove word embeddings to provide a compact and semantic meaningful representation for each word of the input documents. Given the bi-dimensionality constraint of ELM inputs, we reduce the dimensionality of word embeddings using Latent Semantic Analysis and Principal Components Analysis (PCA). Our results reveal that PCA together with the post-processing operation led to more accurate results with lower computational costs.
更多查看译文
关键词
Extreme Learning Machine, Glove Word Embeddings, Post-processing Algorithm, Principal Component Analysis, Latent Semantic Analysis
AI 理解论文
溯源树
样例
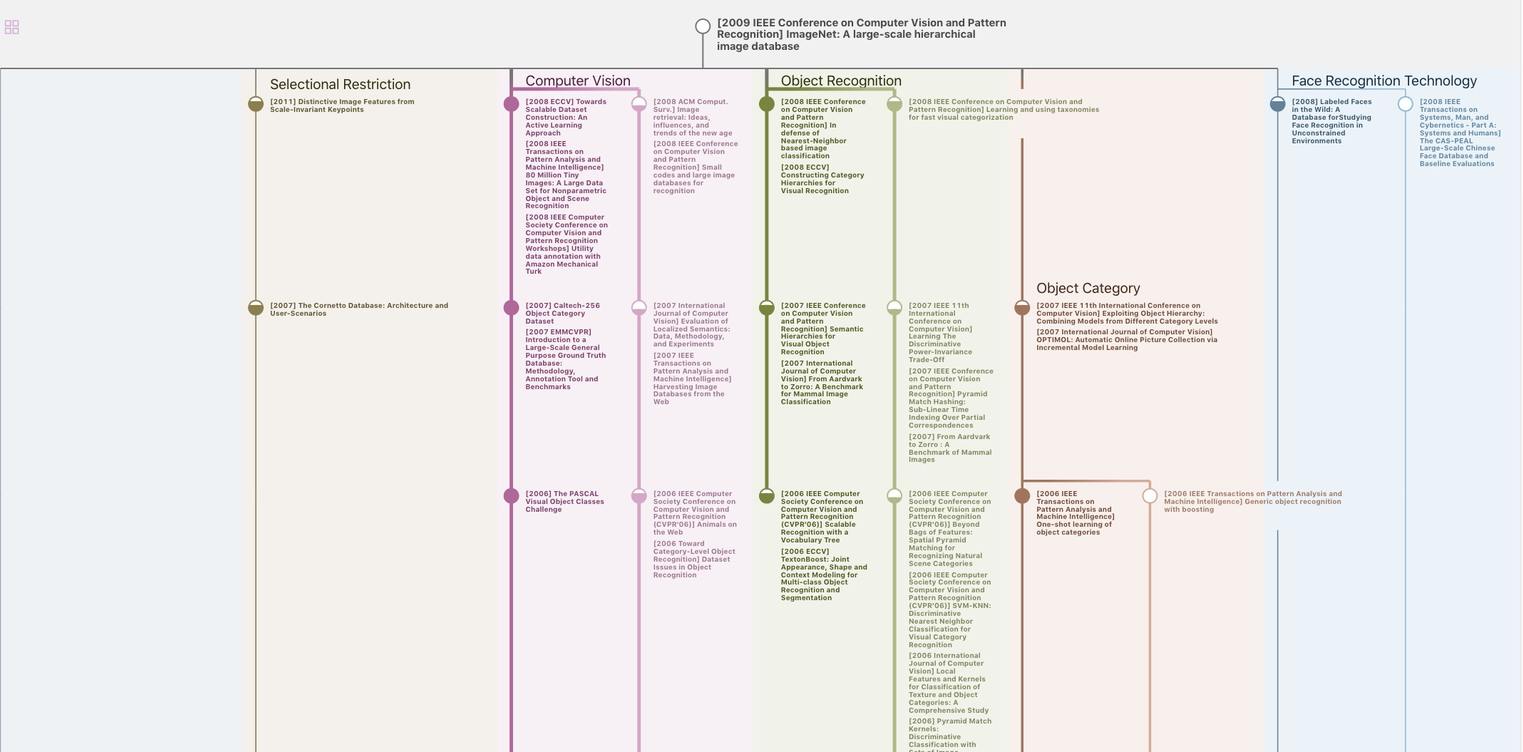
生成溯源树,研究论文发展脉络
Chat Paper
正在生成论文摘要