Multi-Attention DenseNet: A Scattering Medium Imaging Optimization Framework for Visual Data Pre-Processing of Autonomous Driving Systems
IEEE TRANSACTIONS ON INTELLIGENT TRANSPORTATION SYSTEMS(2022)
摘要
The vision system is important for almost all kinds of autonomous driving systems. However, visual data interfered by scattering media, such as smoke, haze, water, and other non-uniform media will be degraded seriously, showing the characteristics of detail loss, poor contrast, low visibility, or color distortion. These characteristics can significantly interfere with the reliability of autonomous driving systems. In real environments the image degradation mechanism is complex, and the estimation of degradation parameters is difficult. This issue remains to be solved. In this study, we employed dense blocks as the framework and introduced the attention mechanism to our model from four dimensions: Multi-scale Attention, Channel Attention, Structure Attention, and ROI (region of interest) Attention. With the help of the training data provided by the weakly supervised model, the proposed method achieved excellent performance in the task of scattering medium imaging optimization in different scenes. Comparative experiments show that the proposed method is robust, and is superior to other state-of-the-art methods in image dehazing, and underwater image enhancement tasks. It is of great significance to improve the reliability of autonomous driving systems in underwater and severe weather environments.
更多查看译文
关键词
Scattering, Imaging, Image restoration, Deep learning, Image color analysis, Degradation, Autonomous vehicles, Attention mechanism, color correction, image dehazing, imaging optimization, image restoration, underwater image enhancement
AI 理解论文
溯源树
样例
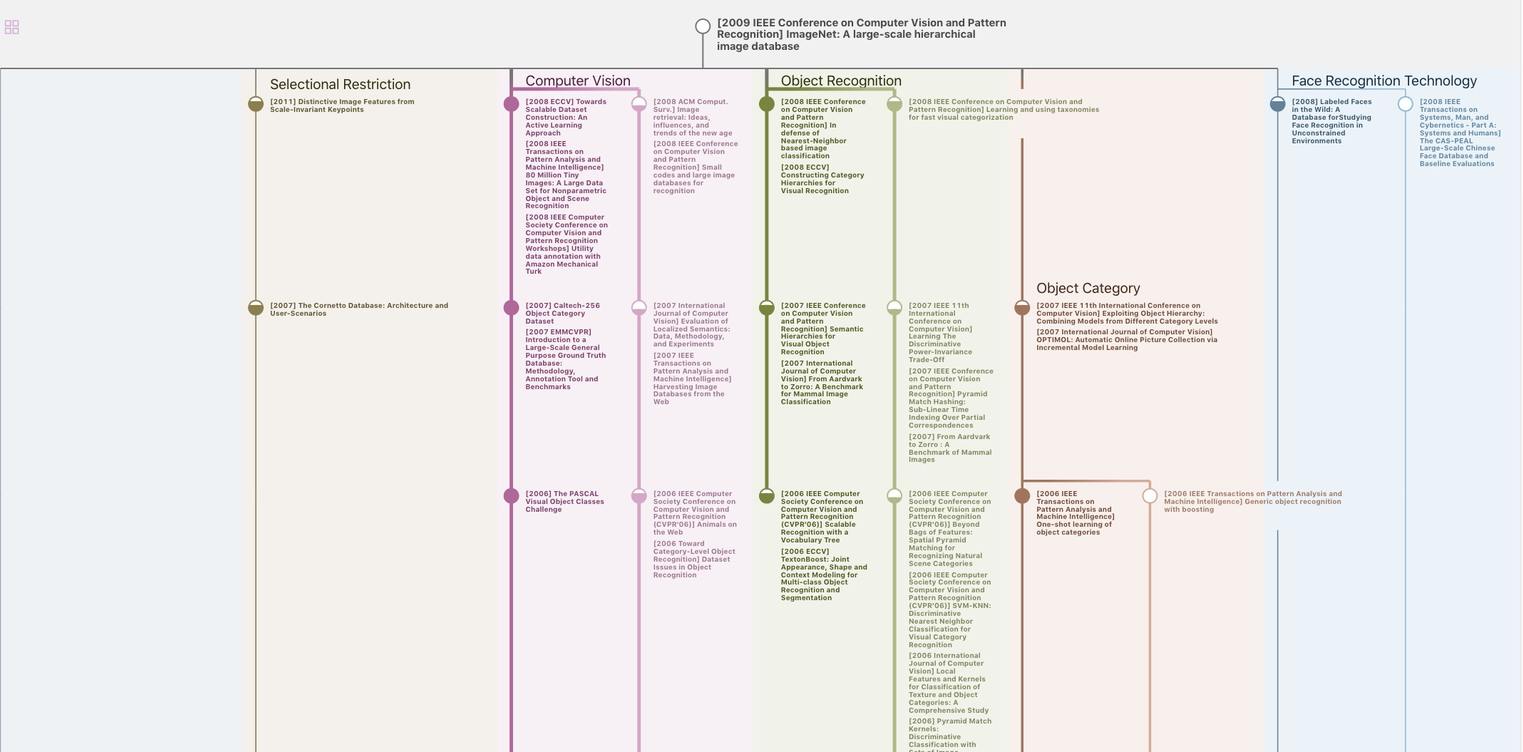
生成溯源树,研究论文发展脉络
Chat Paper
正在生成论文摘要