A SYSTEMATIC REVIEW ON THE DEVELOPMENT OF MACHINE LEARNING MODELS FOR DIFFERENTIATING PCNSL FROM GLIOMAS
NEURO-ONCOLOGY(2021)
摘要
Abstract PURPOSE Differentiating gliomas and Primary CNS Lymphomas (PCNSL) represents a diagnostic challenge with important therapeutic ramifications. MR imaging combined with Machine Learning (ML) has shown promising results in differentiating tumors non-invasively. The purpose of this systematic review is to evaluate and synthesize the findings on the application of ML in differentiating PCNSL and gliomas. MATERIALS AND METHODS A systematic search of literature was performed in October 2020 and February 2021 on Ovid Embase, Ovid MEDLINE, Cochrane trials, and Web of Science – Core Collection. The search strategy included keywords and controlled vocabulary including the terms: gliomas, artificial intelligence, machine learning, and related terms. Publications were reviewed and screened by four different reviewers in accordance with TRIPOD. RESULTS The literature search yielded 11,727 studies and 1,135 underwent full-text review. Data was extracted from 16 publications showing that 10 ML and 3 deep learning (DL) algorithms were tested. The analyzed databases had an average size of 118 patients per study. 50% of the publications validated the algorithm in an independent test cohort. The most commonly tested ML and DL algorithms were support vector machines and Convolutional Neural Networks, respectively. In internal (external) datasets, ML algorithms reached an average AUC of 89% (83%); and DL 74% (77%). Preliminary TRIPOD bias analysis yielded an average score of 0.5 (range 0.31-0.62), with most papers showing deficiencies in reporting model specifications, and funding details among other items. CONCLUSIONS AI-based methods for differentiating gliomas and PCNSL have been reported and show that ML methods result in accuracy = > 85%.With few studies using DL algorithms, further research into novel DL-based approaches is recommended. Additionally, most studies lack large datasets and external validation, thus increasing the risk of overfitting. Bias analysis of the published studies using TRIPOD identified reporting deficiencies, and close adherence to reporting criteria is recommended.
更多查看译文
AI 理解论文
溯源树
样例
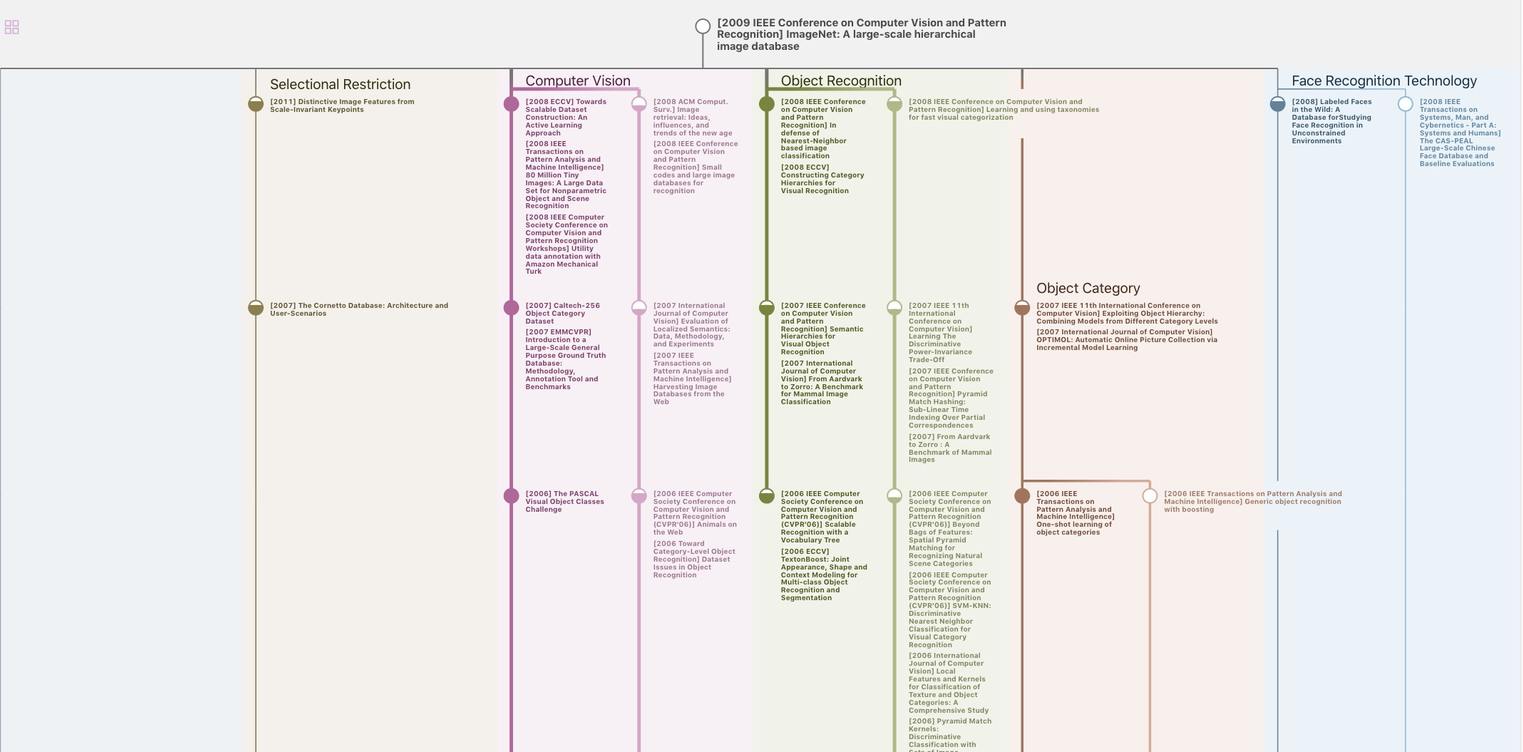
生成溯源树,研究论文发展脉络
Chat Paper
正在生成论文摘要