RFNet: Reverse Fusion Network With Attention Mechanism for RGB-D Indoor Scene Understanding
IEEE TRANSACTIONS ON EMERGING TOPICS IN COMPUTATIONAL INTELLIGENCE(2023)
摘要
RGB-D indoor multiclass scene understanding is a pixelwise task that interprets RGB-D images using depth information to improve the RGB features for higher performance. We propose a novel asymmetric encoder structure for RGB-D indoor scene understanding that uses a reverse fusion network (RFNet) with an attention mechanism and a simplified feature extraction block. Specifically, the pre-trained ResNet34 and VGG16 networks (two asymmetric input streams) are used as the backbone for the information extraction paths as well as additive fusion and attention modules that further enhance network performance. The strong feature extraction ability of classical networks and the advantages of two-way reverse fusion enable this novel semantic segmentation network to narrow the gap between low- and high-level features, such that the features are better merged for segmentation. We achieved segmentation performances (MIoU) of 53.5% and 50.7% on the SUN RGB-D and NYUDv2 datasets, respectively, thereby outperforming other state-of-the-art approaches.
更多查看译文
关键词
Feature extraction, Semantics, Image segmentation, Sun, Computer architecture, Data mining, Computational intelligence, RGB-D, indoor scene understanding, reverse fusion network, attention mechanism
AI 理解论文
溯源树
样例
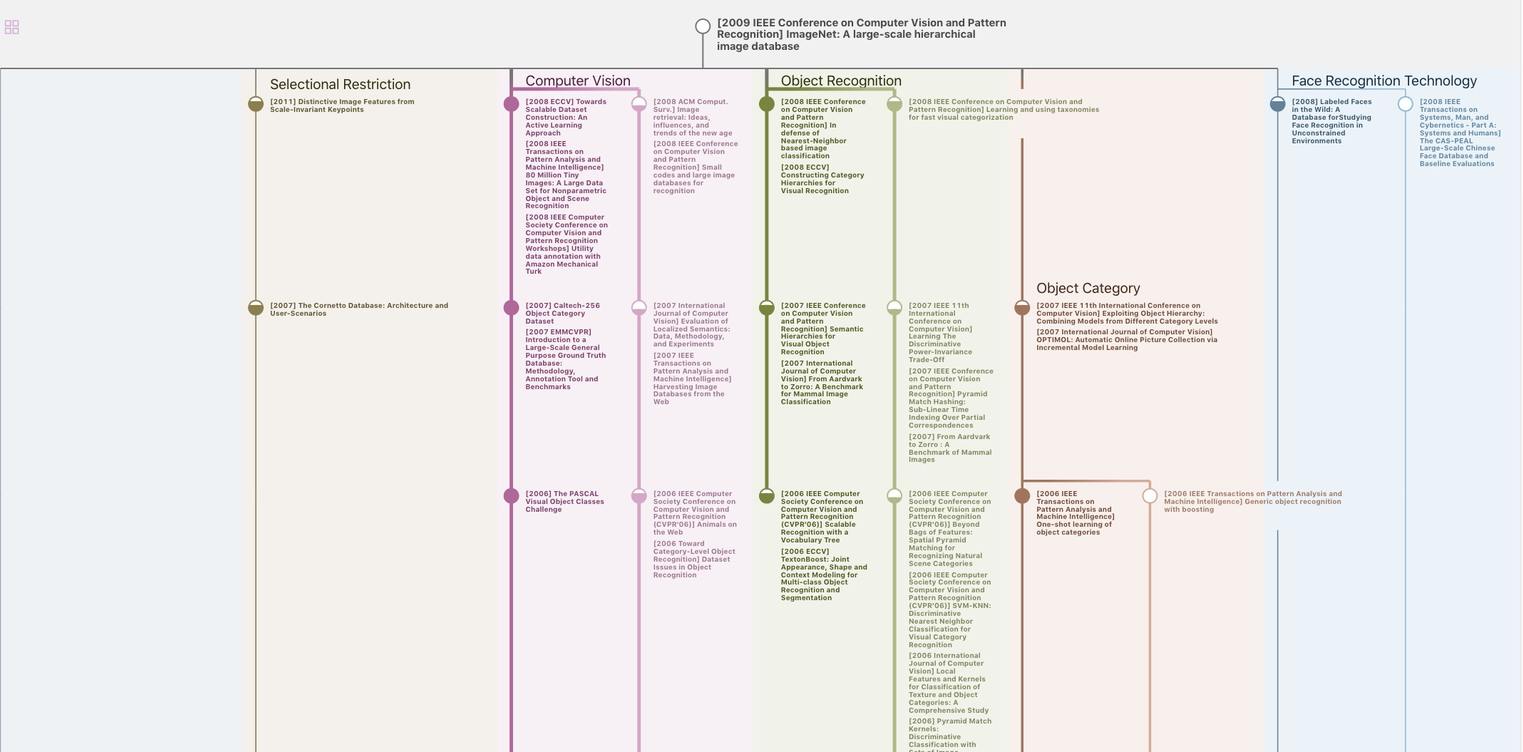
生成溯源树,研究论文发展脉络
Chat Paper
正在生成论文摘要