SPEAKER GENDER IDENTIFICATION IN MATCHED AND MISMATCHED CONDITIONS BASED ON STACKING ENSEMBLE METHOD
JOURNAL OF ENGINEERING SCIENCE AND TECHNOLOGY(2022)
摘要
Identifying the gender of the human voice has been considered one of the challenging tasks because it acts as a pre-processing ingredient for enhancing speech analysis applications. In this work, an automatic system is proposed to identify the speaker's gender without depending on the text in matched and mismatched conditions. Firstly, three groups of features are extracted from each utterance using Fundamental Frequency (F0), Fractal Dimensions, and Mel Frequency Cepstral Coefficient (MFCC) methods. Then, the extracted feature dimensions are reduced using Linear Discriminant Analysis (LDA) method. Finally, the speaker's gender is identified based on proposed stacking ensemble classifier when Logistic Regression (LR), K-Nearest Neighbours (KNN) and Gaussian Naive Bayes (GNB) are used as base classifiers, while Support Vector Machine (SVM) is used as meta classifier. Four experiments are conducted on two datasets: TIMIT, and Common-Voice. In matched conditions (i.e., same language), the proposed system accuracy is 99.74%, 87.28% for the TIMIT, and the Common-Voice dataset, respectively. In mismatched conditions (i.e., cross language), the proposed system shows a high ability to generalize, taking advantage of using the LDA method, where the system accuracy is 81.19%, 97.78% for the (TIMIT\Common-Voice), and (Common-Voice\TIMIT) datasets, respectively. The results also showed a clear superiority for the proposed system in comparison to related works that utilized the TIMIT dataset.
更多查看译文
关键词
Cross-language, Fractal dimensions, Features fusion, LDA, Speaker gender detection
AI 理解论文
溯源树
样例
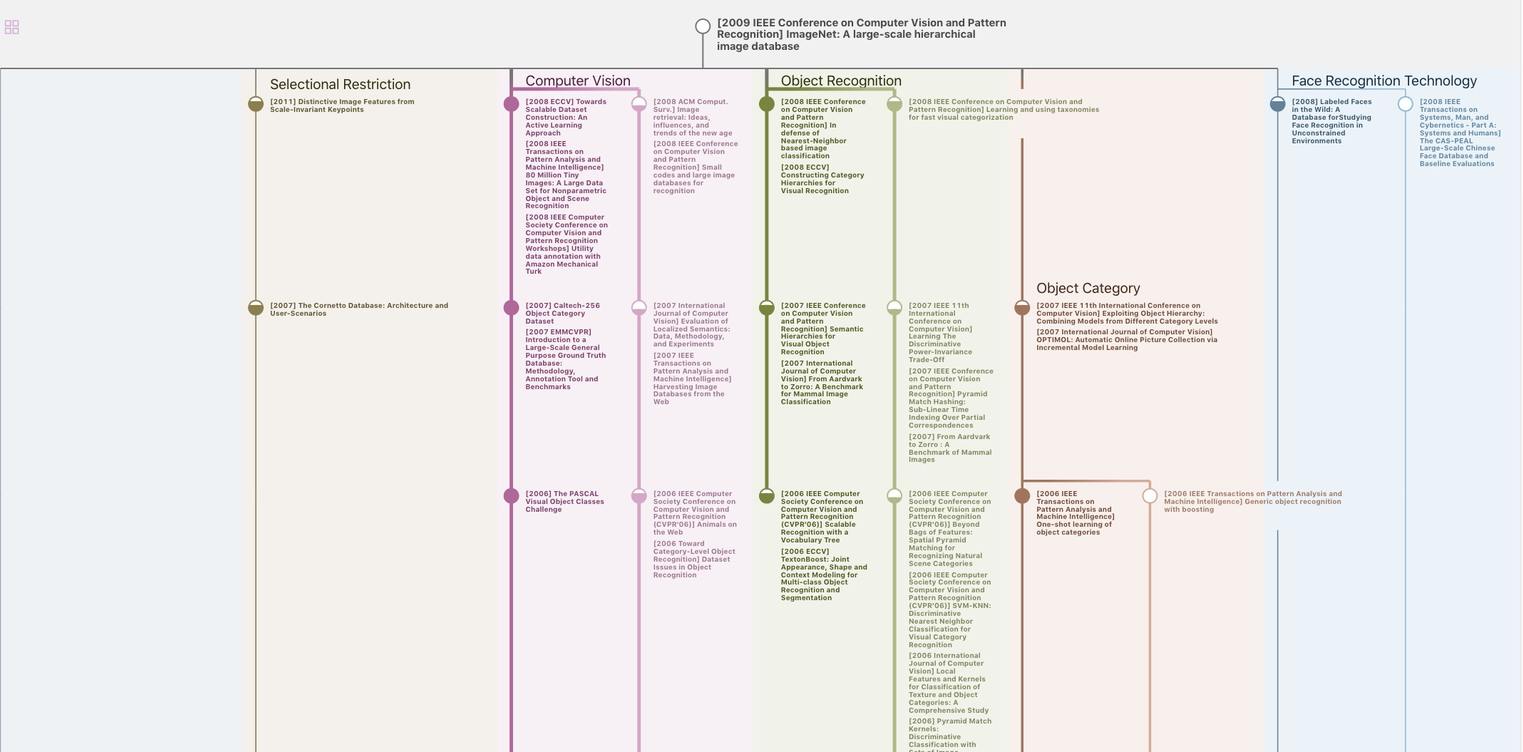
生成溯源树,研究论文发展脉络
Chat Paper
正在生成论文摘要