On-Chip Trainable Spiking Neural Networks Using Time-To-First-Spike Encoding
IEEE ACCESS(2022)
摘要
Artificial Neural Networks (ANNs) have shown remarkable performance in various fields. However, ANN relies on the von-Neumann architecture, which consumes a lot of power. Hardware-based spiking neural networks (SNNs) inspired by a human brain have become an alternative with significantly low power consumption. In this paper, we propose on-chip trainable SNNs using a time-to-first-spike (TTFS) method. We modify the learning rules of conventional SNNs using TTFS to be suitable for on-chip learning. Vertical NAND flash memory cells fabricated by a device manufacturer are used as synaptic devices. The entire learning process considering the hardware implementation is also demonstrated. The performance of the proposed network is evaluated through the MNIST classification in system-level simulation using Python. The proposed SNNs show an accuracy of 96% for a network size of 784 - 400 - 10. We also investigate the effect of non-ideal cell characteristics (such as pulse-to-pulse and device-to-device variations) on inference accuracy. Our networks demonstrate excellent immunity for various device variations compared with the networks using off-chip training.
更多查看译文
关键词
Neurons, Firing, Hardware, Training, System-on-chip, Flash memories, Power demand, Spiking neural networks (SNNs), time-to-first-spike (TTFS), on-chip training, synaptic devices, NAND flash
AI 理解论文
溯源树
样例
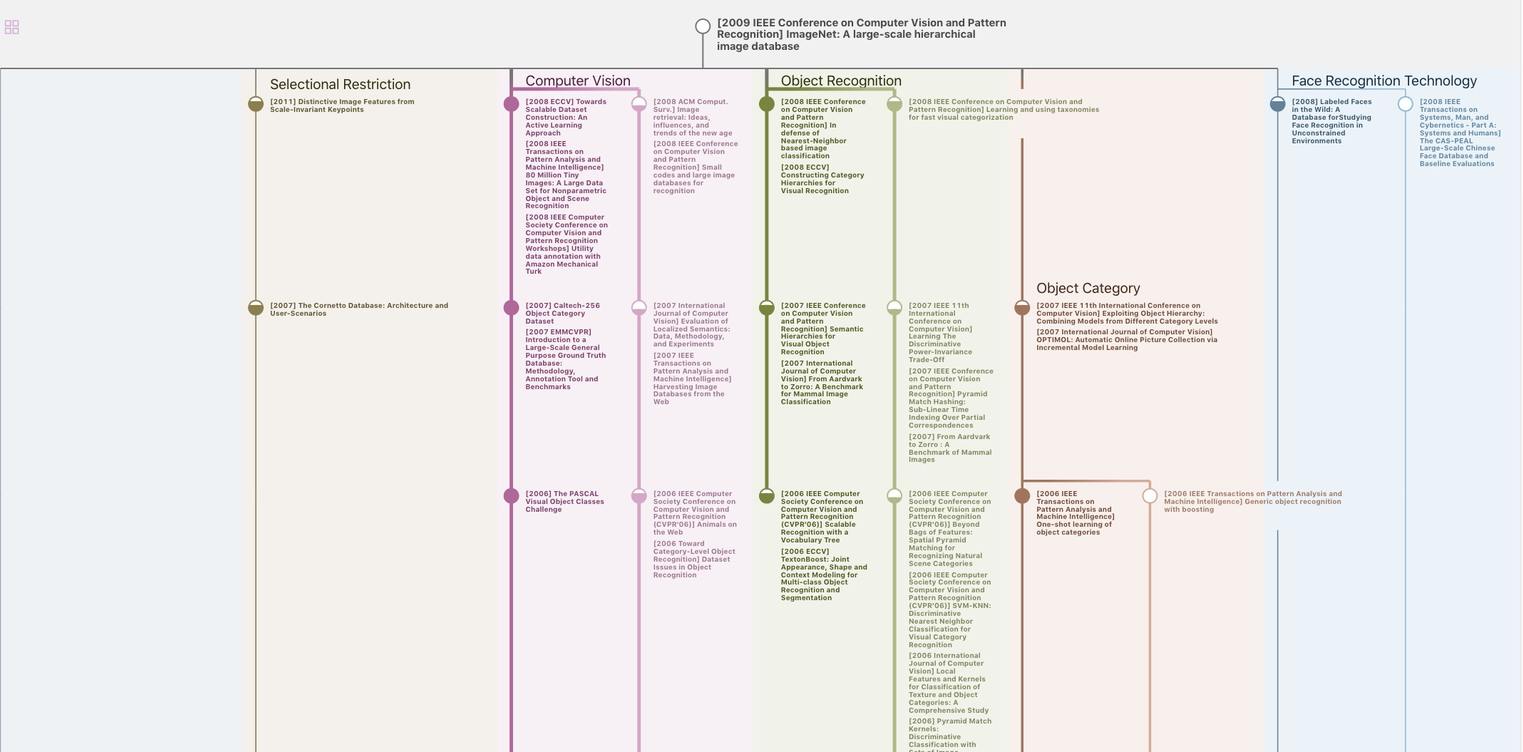
生成溯源树,研究论文发展脉络
Chat Paper
正在生成论文摘要