Multivariate skew Laplace normal distribution for modeling skewness and heavy-tailedness in multivariate data sets
STATISTICS AND ITS INTERFACE(2022)
摘要
Modeling both skewness and heavy-tailedness in multivariate data sets is a challenging problem. The main goal of this paper is to introduce a multivariate skew Laplace normal (MSLN) distribution to deal with the issue by providing a flexible model for modeling skewness and heavy-tailedness simultaneously. This distribution will be an alternative to some multivariate skew distributions including the multivariate skew-t-normal (MSTN) distribution introduced by [28]. This is due to the fact that the MSLN distribution has fewer parameters than most of these distributions, which causes computationally advantageous for the MSLN distribution over these distributions. The definition, some distributional properties of this distribution are studied. The maximum likelihood (ML) estimators for the parameters of the MSLN distribution are obtained via the expectation-maximization (EM) algorithm. A simulation study and a real data example are also provided to illustrate the capability of the MSLN distribution for modeling data sets in multivariate settings.
更多查看译文
关键词
EM algorithm, ML estimation, MSGLN, MSLN, MSTN
AI 理解论文
溯源树
样例
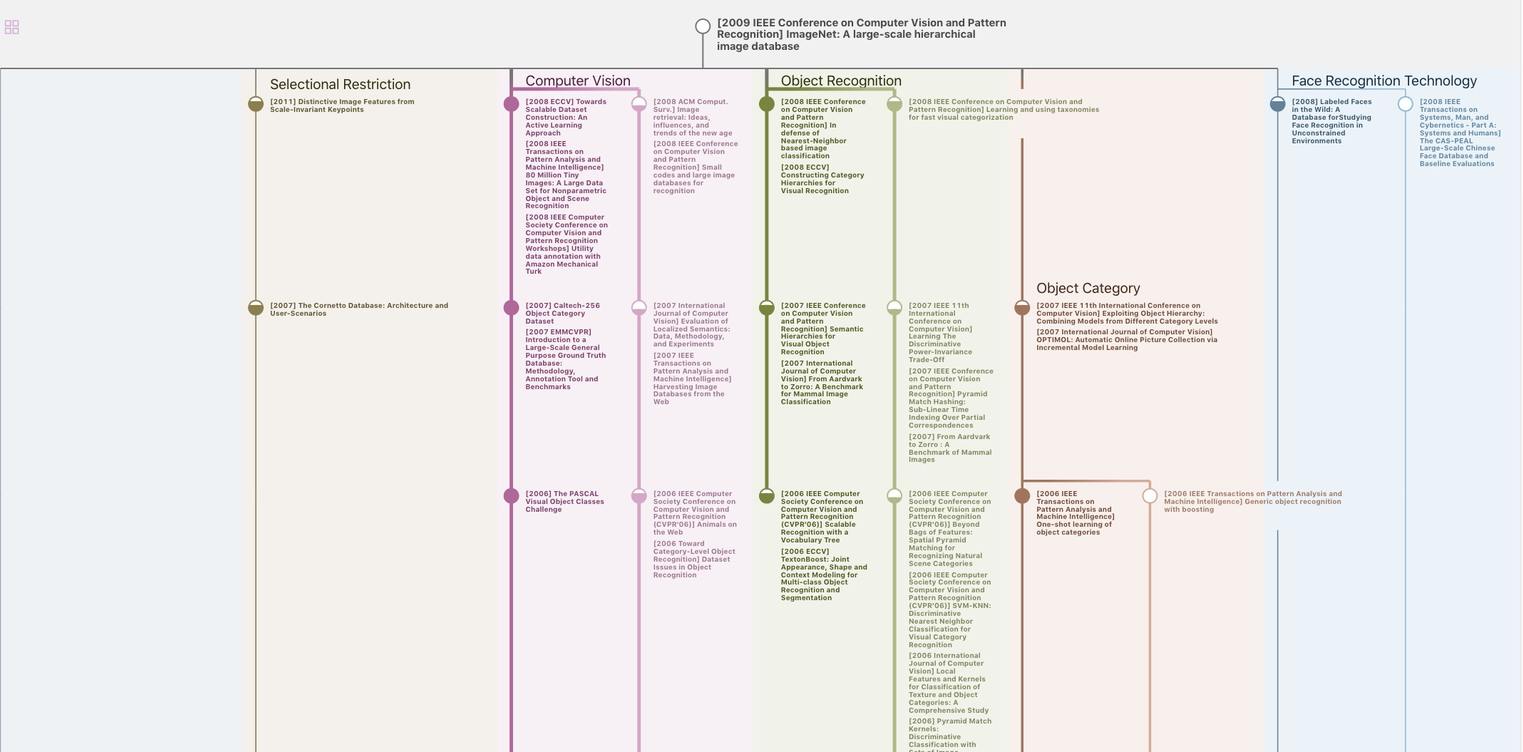
生成溯源树,研究论文发展脉络
Chat Paper
正在生成论文摘要