Variable selection in double/debiased machine learning for causal inference: an outcome-adaptive approach
COMMUNICATIONS IN STATISTICS-SIMULATION AND COMPUTATION(2023)
摘要
Access to high-dimensional data has made the use of machine learning in causal inference more common in recent years. The double/debiased machine learning (DML) estimator for the treatment effect is designed to obtain a valid inference when nuisance functions in the treatment and outcome equations, are estimated using machine learning methods. However, when some covariates in the treatment equation do not appear in the outcome equation, the inclusion of such covariates in the propensity score estimation will result in the increasing bias and variance of the DML estimator. To solve this issue, we introduce an outcome-adaptive DML estimator, which incorporates the outcome-adaptive lasso for the variable selection in the propensity score estimation. We evaluate the performance of the proposed method using Monte Carlo simulation. The results indicate that our proposed method in many cases outperforms other methods.
更多查看译文
关键词
causal inference, Double, debiased machine learning, High-dimensional data, Machine learning, Outcome-adaptive lasso
AI 理解论文
溯源树
样例
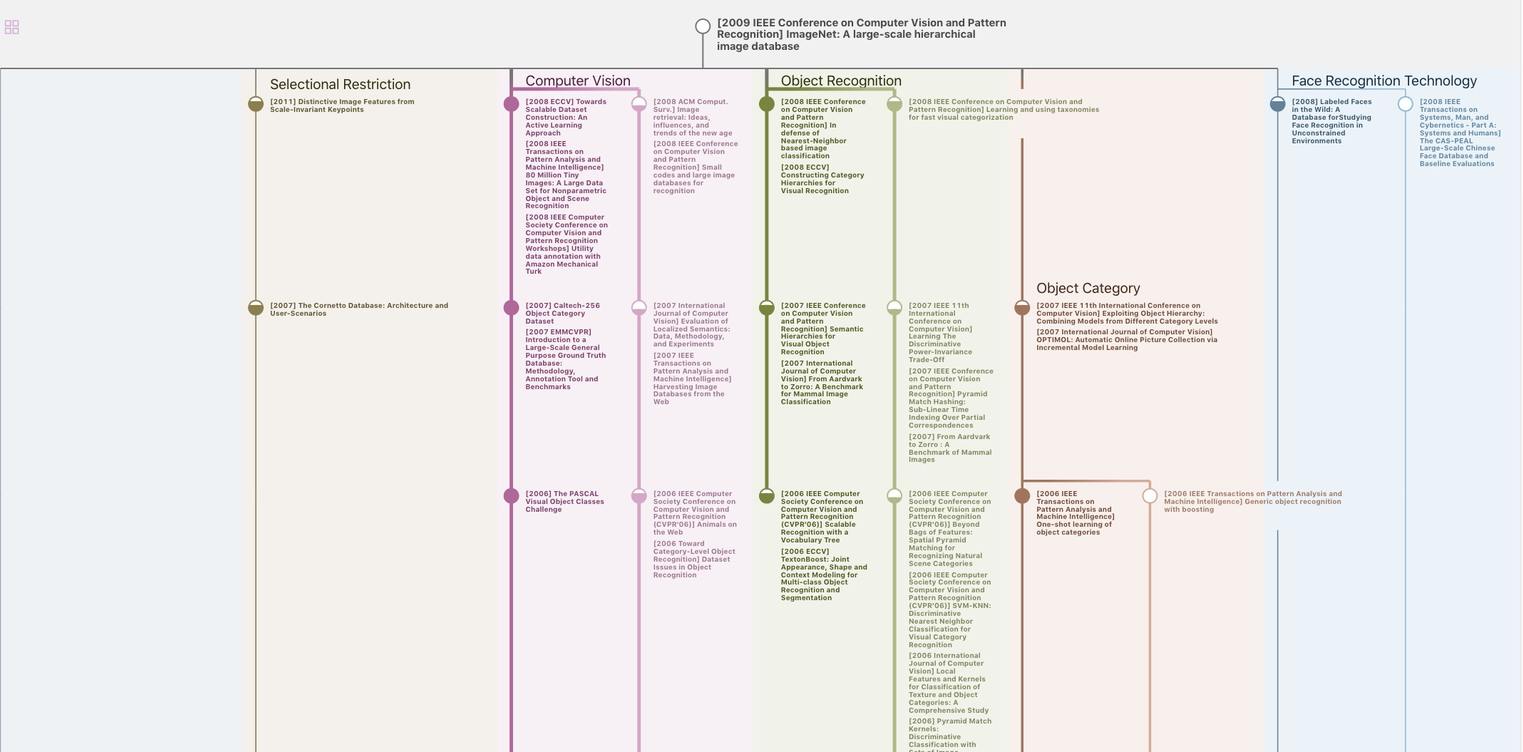
生成溯源树,研究论文发展脉络
Chat Paper
正在生成论文摘要