Spatio-Temporal Model Variance-Covariance Approach to Assimilating Streamflow Observations Into a Distributed Landscape Water Balance Model
WATER RESOURCES RESEARCH(2022)
摘要
Accurate initial conditions play a critical role in improving predictive accuracy of hydrological models for quantities such as streamflow generation. Streamflow observations from in situ gauging sites have been assimilated in a wide range of past research to improve lumped catchment streamflow. However, spatio-temporal state updating in distributed hydrological models through streamflow data assimilation (DA) remains a challenge due to the large dimensional disparity between model state space and observation space. This study explores the use of model spatio-temporal variance-covariance from simulated climatology as a surrogate for model error, as a way of distributing constraint from gauge measurements spatially. In the approach developed here, grid cells within and surrounding gauged catchments were updated simultaneously. The proposed DA method leads to a substantial improvement in performance and reliability compared to open-loop (OL) estimates of streamflow. In particular, for "ungauged" catchments updated using streamflow observations from neighboring catchments, the mean absolute error (MAE) was reduced by 20% on average after the assimilation. In addition, improvements from using the analyzed states as initial conditions were found to persist for more than 1 week, and even longer for catchments with high annual runoff. The improvement in streamflow forecasts using initial conditions from DA can be more than 40% in terms of MAE compared to OL results for 1 day lead time, and more than 10% for 5 days lead time.
更多查看译文
关键词
data assimilation, streamflow, error variance-covariance, water balance modeling
AI 理解论文
溯源树
样例
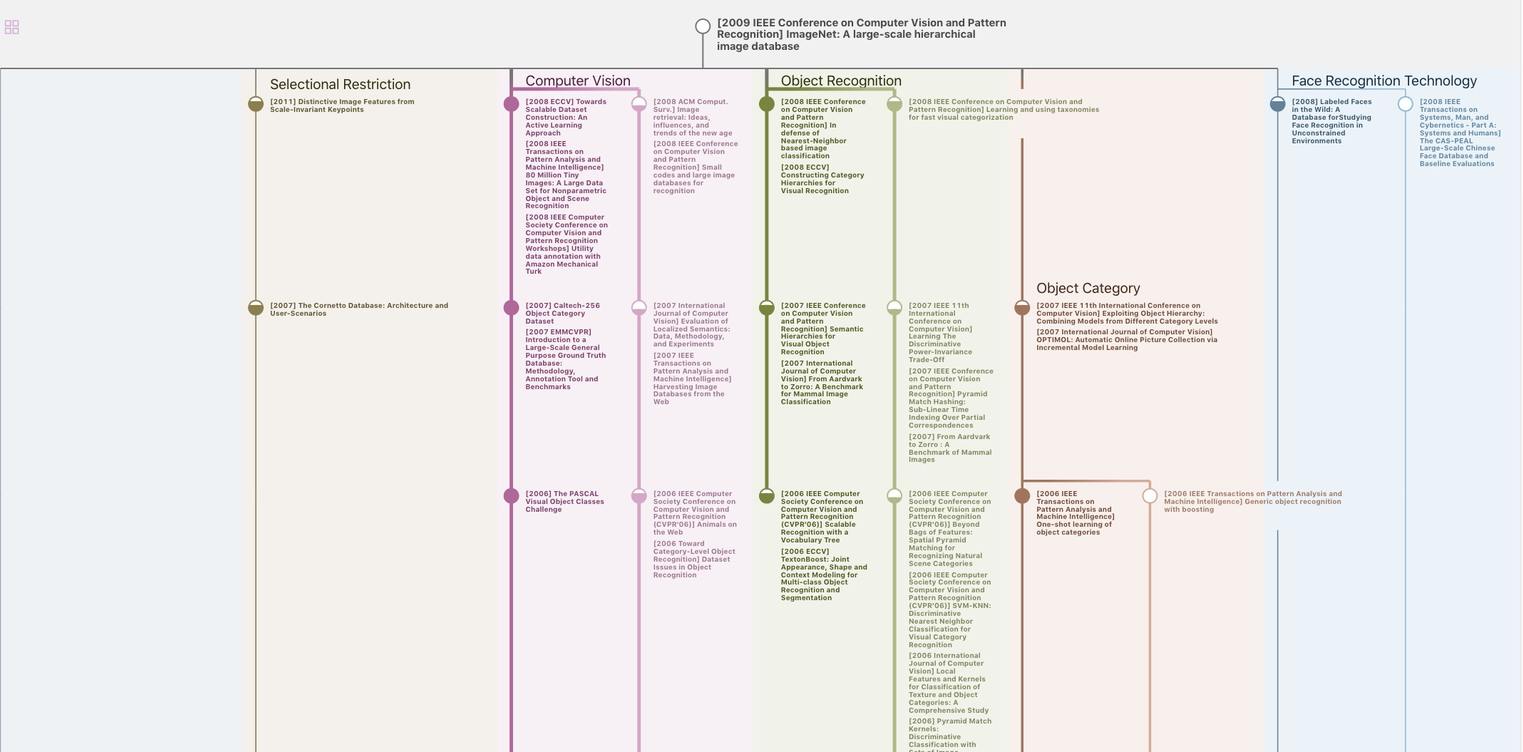
生成溯源树,研究论文发展脉络
Chat Paper
正在生成论文摘要