A Structure-Aware Adversarial Framework with the Keypoint Biorientation Field for Multiperson Pose Estimation
WIRELESS COMMUNICATIONS & MOBILE COMPUTING(2022)
摘要
Human pose estimation is aimed at locating the anatomical parts or keypoints of the human body and is regarded as a core component in obtaining detailed human understanding in images or videos. However, the occlusion and overlap upon human bodies and complex backgrounds often result in implausible pose predictions. To address the problem, we propose a structure-aware adversarial framework, which combines cues of local joint interconnectivity and priors about the holistic structure of human bodies, achieving high-quality results for multiperson human pose estimation. Effective learning of such cues and priors is typically a challenge. The presented framework uses a nonparametric representation, which is referred to as the Keypoint Biorientation Field (KBOF), to learn orientation cues of joint interinteractivity in the image, just as human vision can explore geometric constraints of joint interconnectivity. Additionally, a module using multiscale feature representation with inflated convolution for joint heatmap detection and Keypoint Biorientation Field detection is applied in our framework to fully explore the local features of joint points and the bidirectional connectivity between them at the microscopic level. Finally, we employ improving generative adversarial networks which use KBOF and multiscale feature extraction that implicitly leverages the cues and priors about the structure of human bodies for global structural inference. The adversarial network enables our framework to combine information about the connections between local body joints at the microscopic level and the structural priors of the human body at the global level, thus enhancing the performance of our framework. The effectiveness and robustness of the network are evaluated on the task of human pose prediction in two widely used benchmark datasets, i.e., MPII and COCO datasets. Our approach outperforms the state-of-the-art methods, especially in the case of complex scenes. Our method achieves an improvement of 2.6% and 1.7% compared to the latest method on the MPII test set and COCO validation set, respectively.
更多查看译文
AI 理解论文
溯源树
样例
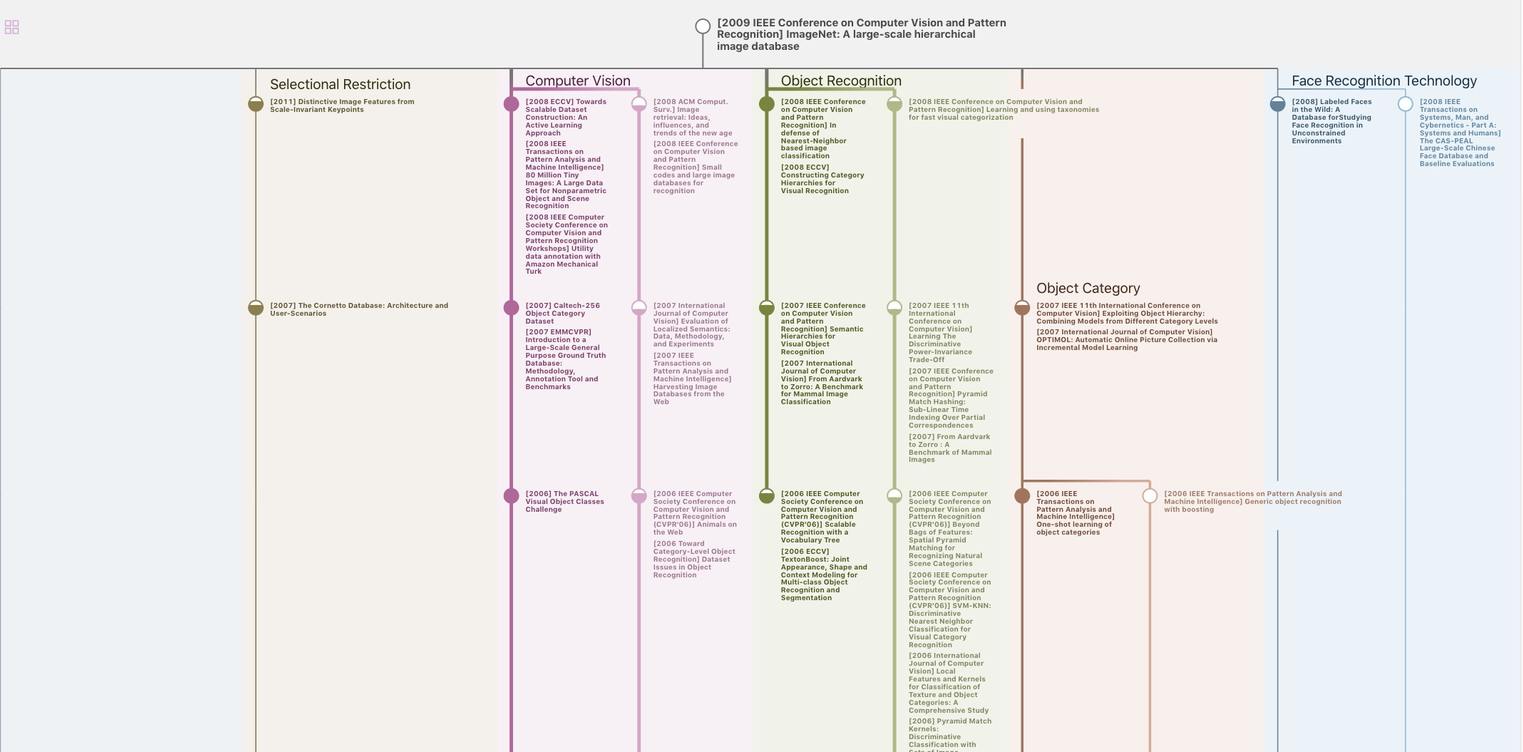
生成溯源树,研究论文发展脉络
Chat Paper
正在生成论文摘要