Multi-scale Attention Guided Recurrent Neural Network for Deformation Map Forecasting
IMAGE AND SIGNAL PROCESSING FOR REMOTE SENSING XXVII(2021)
摘要
Deformation map prediction is a critical tool to foresee signs of abnormal events. Such forecasting facilitates quick countermeasure to avoid undesirable conditions. This work presents a novel recurrent neural network to forecast time-series deformation maps from InSAR data. Our proposed recurrent network employs a multi-scale attention mechanism to identify vital temporal features that influences subsequent deformation maps. We have evaluated our model on volcanic monitoring data using the Micronesia islands (Canary and Cape Verde archipelagos) Sentinel-1 imagery acquired between 2015 and 2018. The proposed method achieves minimal prediction error compared to the observed deformation values, suggesting the high reliability of our approach. The experimental results indicate the superiority of the proposed method in forecasting deformation maps with high accuracy compared to existing state-of-the-art approaches. Various ablation studies were conducted to study and validate the effectiveness of the multi-scale attention mechanism for deformation map forecasting.
更多查看译文
关键词
SAR Image Processing, Remote Sensing, Deformation map forecasting, Convolutional Neural Network, Recurrent network, LSTM
AI 理解论文
溯源树
样例
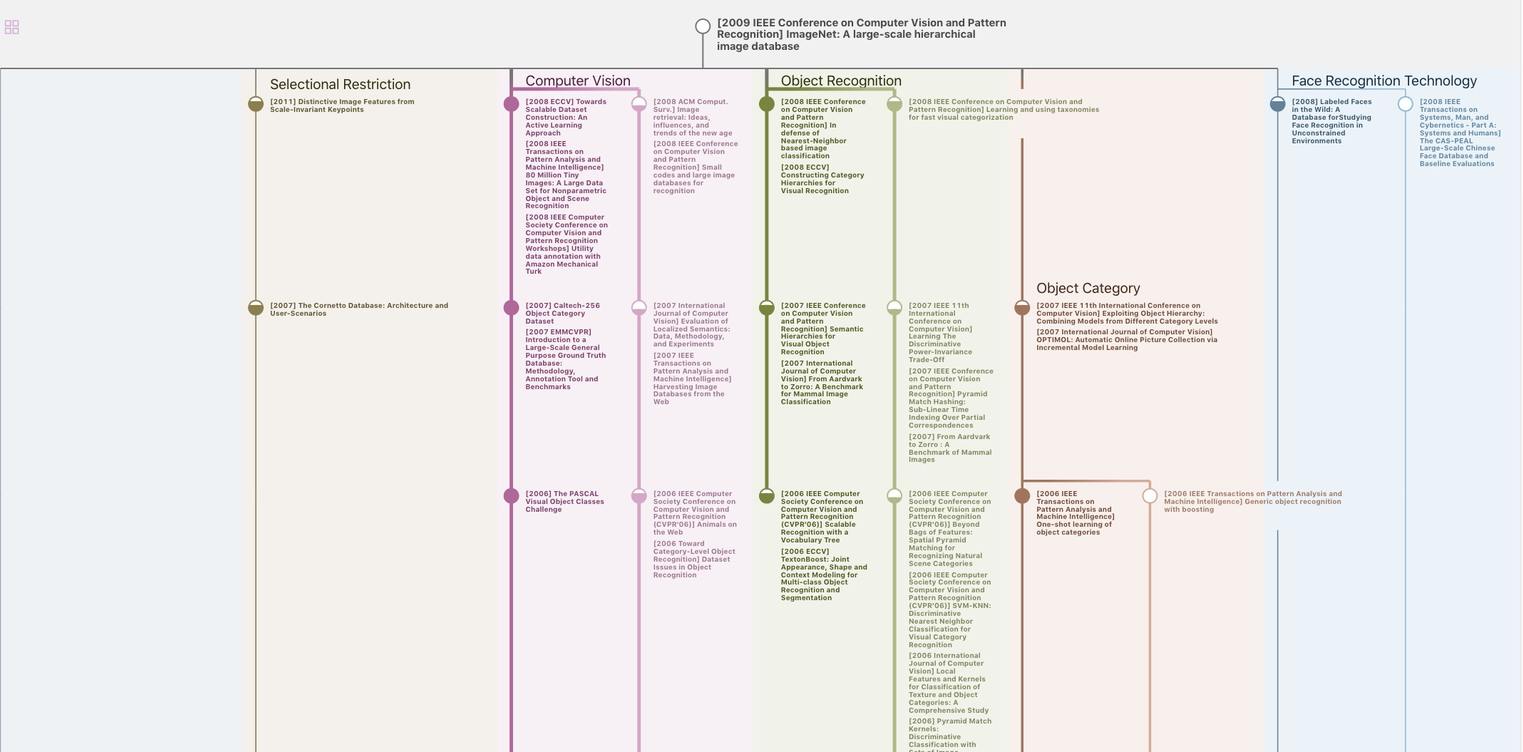
生成溯源树,研究论文发展脉络
Chat Paper
正在生成论文摘要