A Temporary Transformer Network for Guide-Wire Segmentation
2021 14TH INTERNATIONAL CONGRESS ON IMAGE AND SIGNAL PROCESSING, BIOMEDICAL ENGINEERING AND INFORMATICS (CISP-BMEI 2021)(2021)
摘要
In this paper, we solve the task of guide-wire segmentation in X-ray fluoroscopy sequences. Existing deep learning-based guide-wire segmentation models are mainly based on U-Net - a famous convolutional neural network (CNN) in the field of medical image segmentation. Despite its effectiveness, U-Net lacks the ability of modeling long-range dependency. Recently, transformers have attracted increasing attention due to its outstanding performance on modeling long-range dependency. Therefore, we try to adopt transformers to guide-wire segmentation task in this paper. In addition, existing models usually take the single frame as input and ignore the related information between different X-ray fluoroscopy frames in the same sequence. However, the related information can help networks to distinguish the common object through the entire sequence. From this insight, in this paper, we propose a temporary transformer network for guide-wire segmentation. Our network takes the current frame and the previous frames as inputs to obtain temporary information. And the transformers layers utilized in our network help capturing the long-range dependency in X-ray fluoroscopy sequences. Experimental results on the dataset from three hospitals demonstrate the-state-of-the-art performance of our network.
更多查看译文
关键词
temporary transformer network,segmentation,wire
AI 理解论文
溯源树
样例
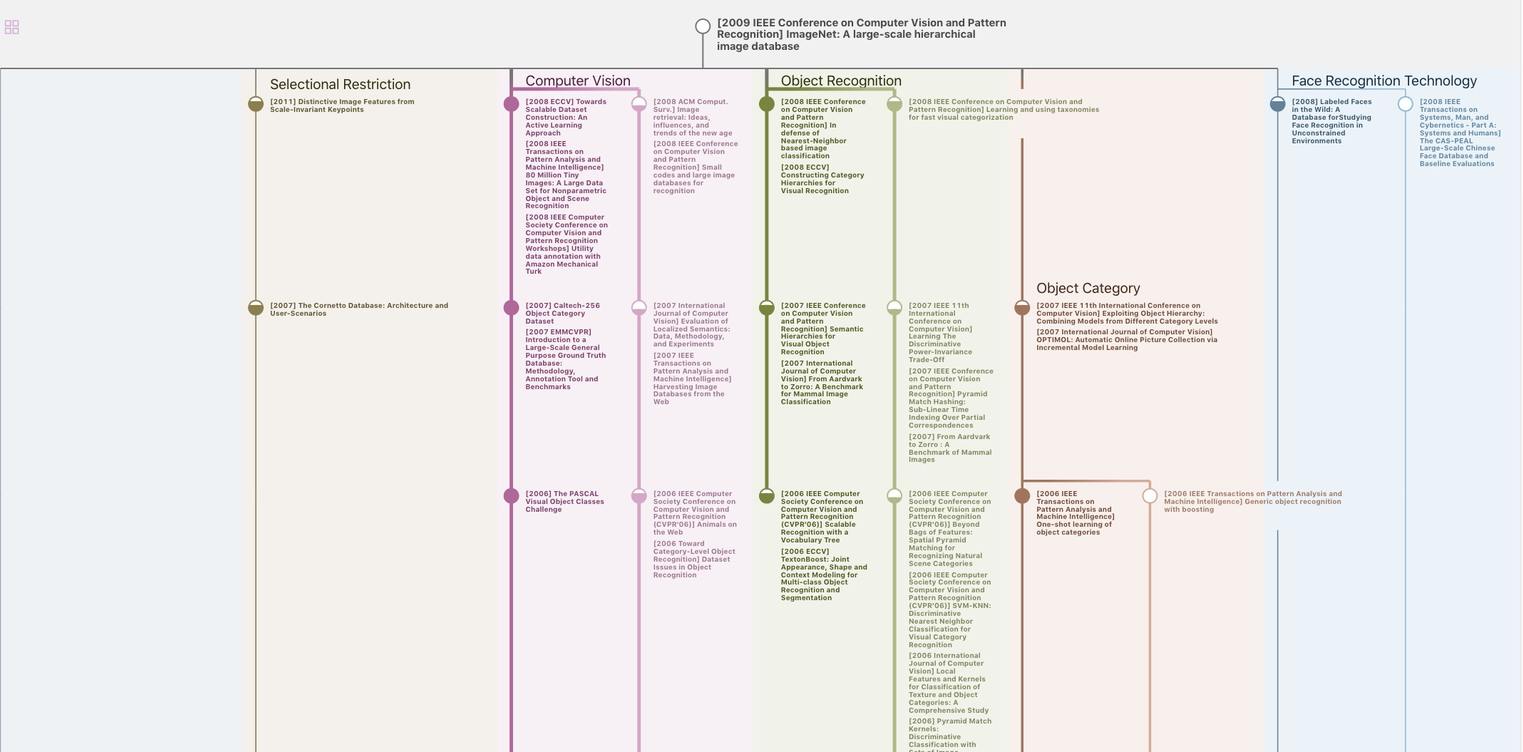
生成溯源树,研究论文发展脉络
Chat Paper
正在生成论文摘要