An Improved Action Recognition Network With Temporal Extraction and Feature Enhancement
IEEE ACCESS(2022)
摘要
Image classification and action recognition are both active research topics in the field of computer vision. However, the development of action recognition is rather slow compared with image classification, due to the difficulties in spatial-temporal information modeling. In this paper, we present TEFE, a deep structure combining temporal extraction with feature enhancement to explore the spatial coherence across temporal dimension. The temporal extraction (TE) module is used to capture the short-term and long-term temporal features. Considering the spatial and temporal cues are fine-grained, we believe such cues (if encoded by well-designed bilinear models) could enhance the representation of actions in videos. The feature enhancement (FE) module approximates bilinear pooling operations, which greatly reduce the amount of parameters exist in original bilinear pooling. Extensive experiments have been conducted on mainstream datasets of human action (Something - Something V1 & V2, and Jester). Experimental results show that our model achieves competitive performances than some existing methods.
更多查看译文
关键词
Feature extraction, Licenses, Convolution, Streaming media, Spatiotemporal phenomena, Optical flow, Kernel, Action recognition, spatial-temporal information modelling, feature enhancement
AI 理解论文
溯源树
样例
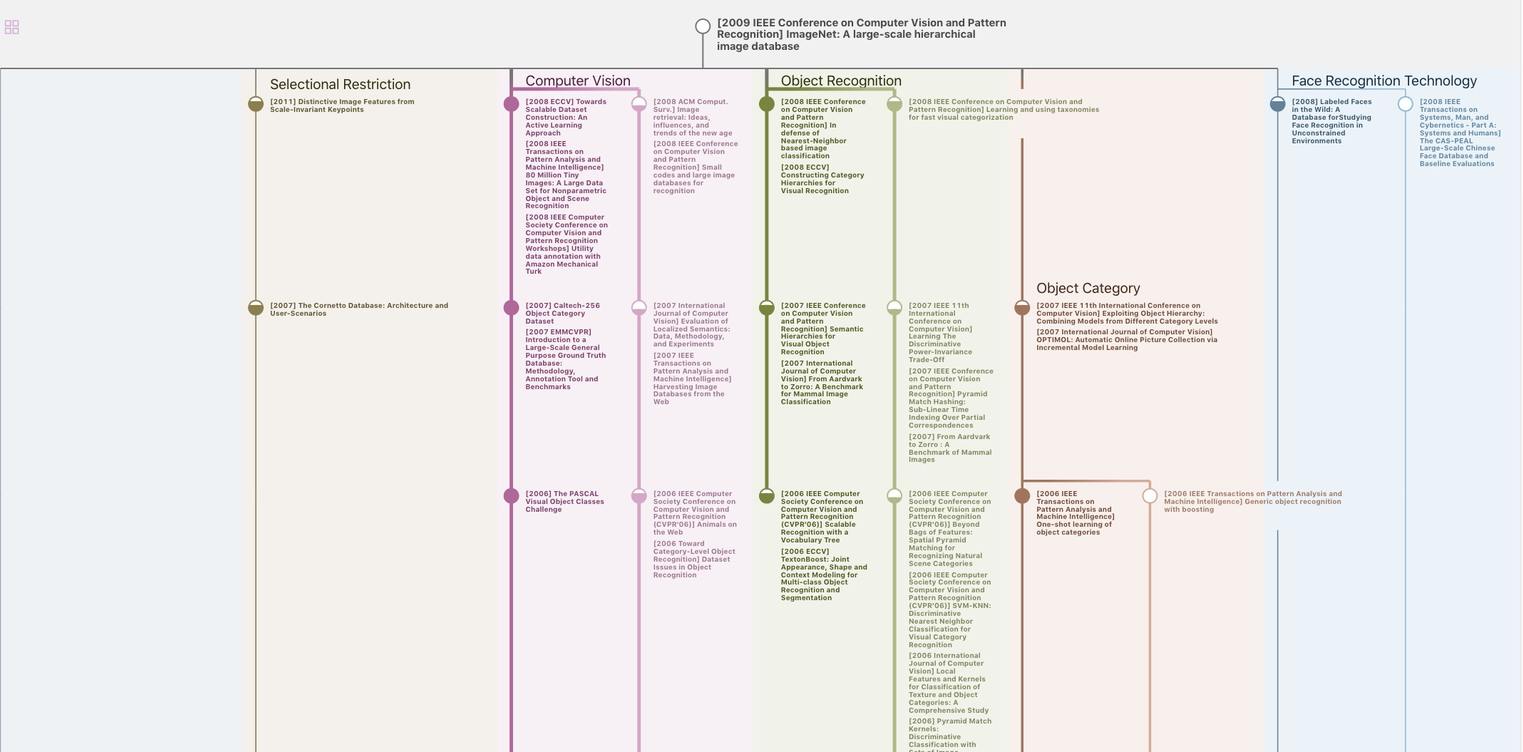
生成溯源树,研究论文发展脉络
Chat Paper
正在生成论文摘要