MuSDRI: Multi-Seasonal Decomposition Based Recurrent Imputation for Time Series
IEEE SENSORS JOURNAL(2021)
摘要
Missing values are a common problem of time series such as sensor-generated data due to issues of data collection, transmission, and storage. To deal with this problem, various data imputation methods have been proposed. Among these methods, variants of recurrent neural networks (RNNs) have attracted a lot of attention as they provide a natural representation of temporal dynamics. Though such methods have been shown to achieve higher imputation accuracy, they mostly focus on the modeling of temporal dynamics from a short-term perspective while ignoring the long-term features such as trend and seasonal information embedded in time series data. In this paper, we investigate different paradigms of time series imputation methods to capture temporal dynamics from both long-term and short-term perspectives. In particular, we propose a new learning paradigm called MuSDRI which combines the time series decomposition method Seasonal Trend decomposition using Loess (STL) with RNNs. Specifically, STL is used to decompose long sequences of time series into trend, seasonal, and remainder components such that long-term dynamics can be revealed; RNNs are used to capture local patterns from the remainder component extracted by STL such that short-term dynamics can be refined. Moreover, MuSDRI is able to handle time series with multiple seasonal patterns by learning their contributions with respect to the imputation task. We evaluate the proposed imputation methods on three real-world datasets. Experimental results show that the imputation methods considering both the long-term and short-term dynamics achieve higher imputation accuracy, and among them MuSDRI in general achieves the best performance.
更多查看译文
关键词
Time series analysis, Market research, Data models, Task analysis, Sensors, Predictive models, Feature extraction, Data imputation, multi-seasonal decomposition, recurrent neural networks, time series
AI 理解论文
溯源树
样例
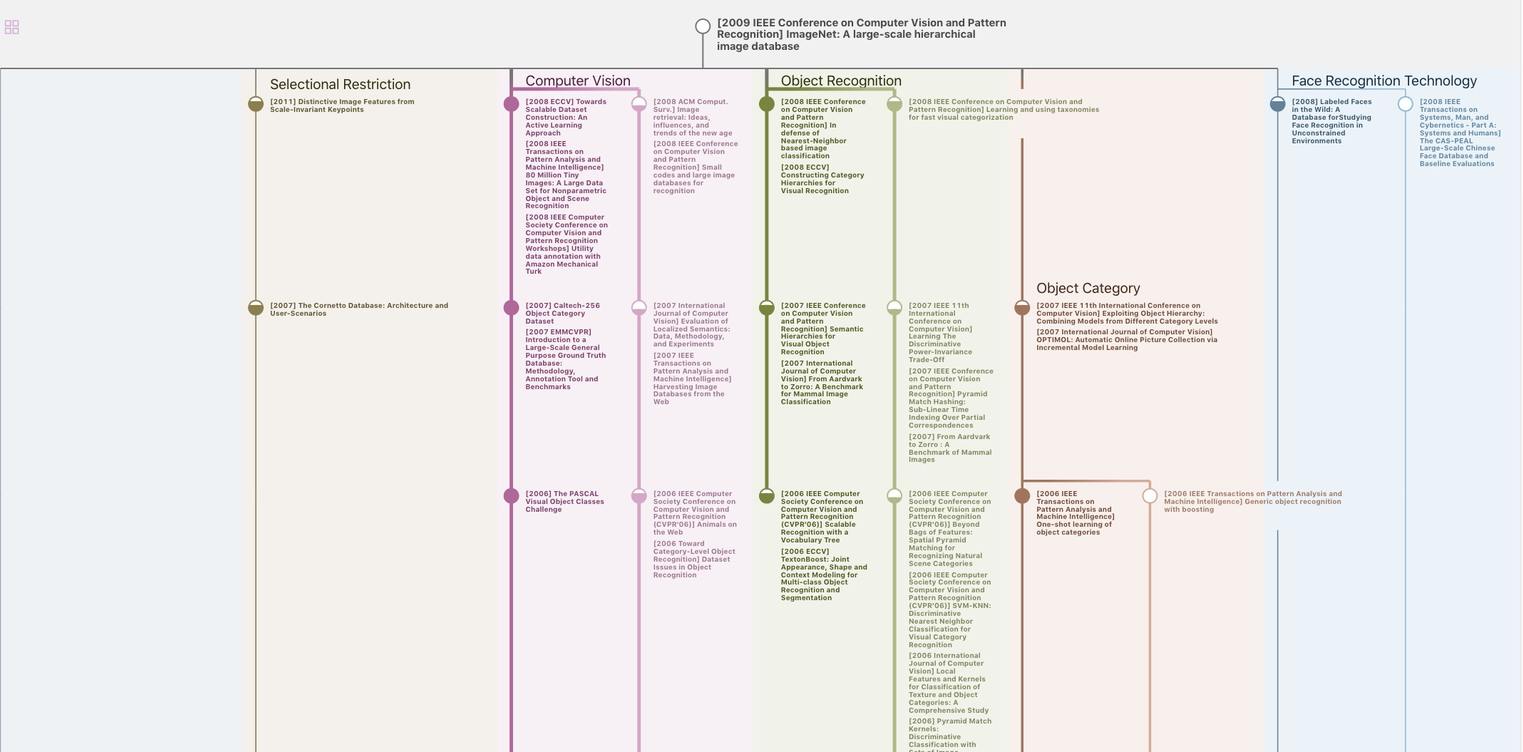
生成溯源树,研究论文发展脉络
Chat Paper
正在生成论文摘要