Multilevel Attention and Multiscale Feature Fusion Network for Author Classification of Chinese Ink-Wash Paintings
DISCRETE DYNAMICS IN NATURE AND SOCIETY(2022)
摘要
How to effectively extract features with high representation ability has always been a research topic and a challenge for classification tasks. Most of the existing methods mainly solve the problem by using deep convolutional neural networks as feature extractors. Although a series of excellent network structures have been successful in the field of Chinese ink-wash painting classification, but most of them adopted the methods of only simple augmentation of the network structures and direct fusion of different scale features, which limit the network to further extract semantically rich and scale-invariant feature information, thus hindering the improvement of classification performance. In this paper, a novel model based on multi-level attention and multi-scale feature fusion is proposed. The model extracts three types of feature maps from the low-level, middle-level and high-level layers of the pretrained deep neural network firstly. Then, the low-level and middle-level feature maps are processed by the spatial attention module, nevertheless the high-level feature maps are processed by the scale invariance module to increase the scale-invariance properties. Moreover, the conditional random field module is adopted to fuse the optimized three-scale feature maps, and the channel attention module is followed to refine the features. Finally, the multi-level deep supervision strategy is utilized to optimize the model for better performance. To verify the effectiveness of the model, extensive experimental results on the Chinese ink-wash painting dataset created in this work show that the classification performance of the model is better than other mainstream research methods.
更多查看译文
AI 理解论文
溯源树
样例
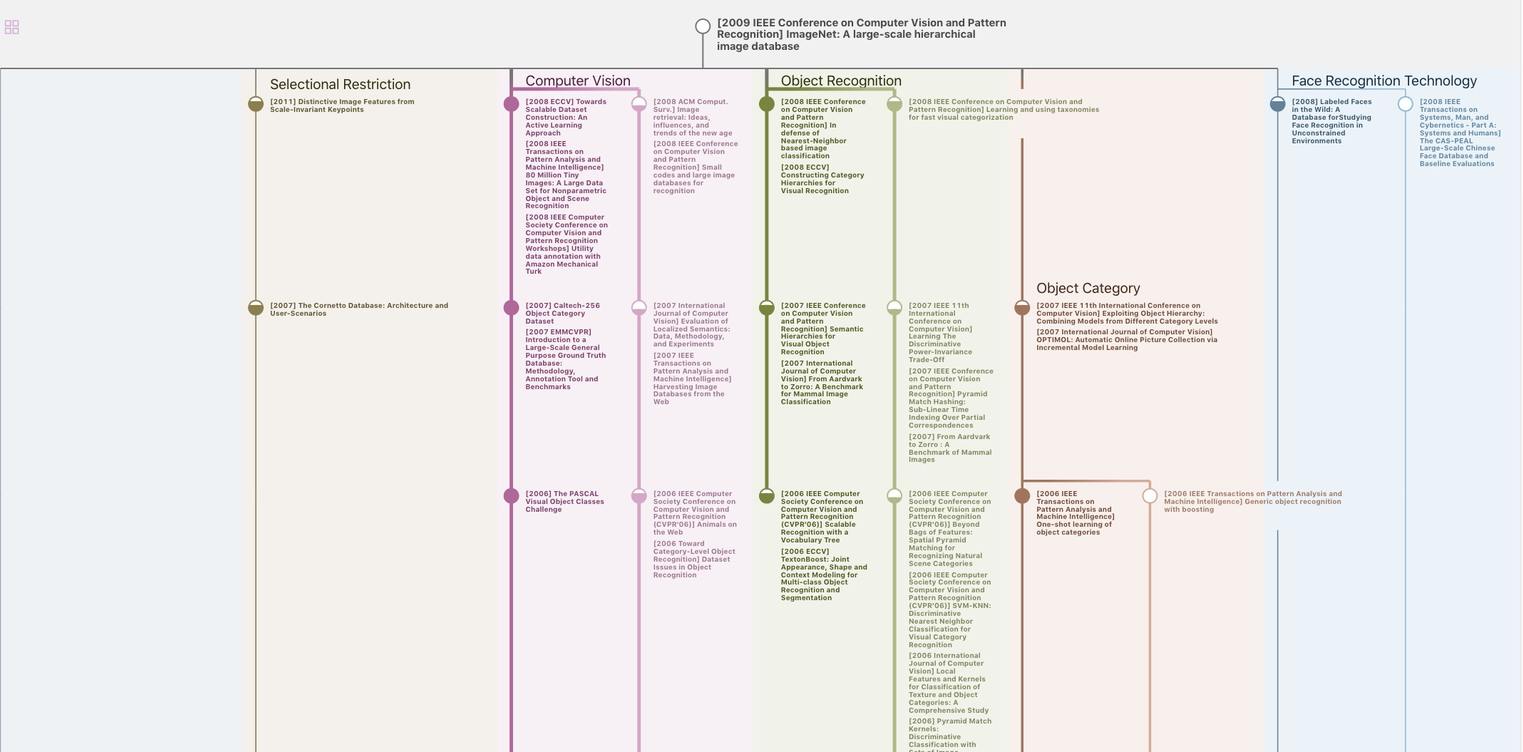
生成溯源树,研究论文发展脉络
Chat Paper
正在生成论文摘要