Non-parametric Learning of Embeddings for Relational Data Using Gaifman Locality Theorem
INDUCTIVE LOGIC PROGRAMMING (ILP 2021)(2021)
摘要
We consider the problem of full model learning from relational data. To this effect, we construct embeddings using symbolic trees learned in a non-parametric manner. The trees are treated as a decisionlist of first order rules that are then partially grounded and counted over local neighborhoods of a Gaifman graph to obtain the feature representations. We propose the first method for learning these relational features using a Gaifman graph by using relational tree distances. Our empirical evaluation on real data sets demonstrates the superiority of our approach over handcrafted rules, classical rule-learning approaches, the state-of-the-art relational learning methods and embedding methods.
更多查看译文
关键词
Statistical relational learning, Gaifman locality theorem, Embeddings, Relational density estimation
AI 理解论文
溯源树
样例
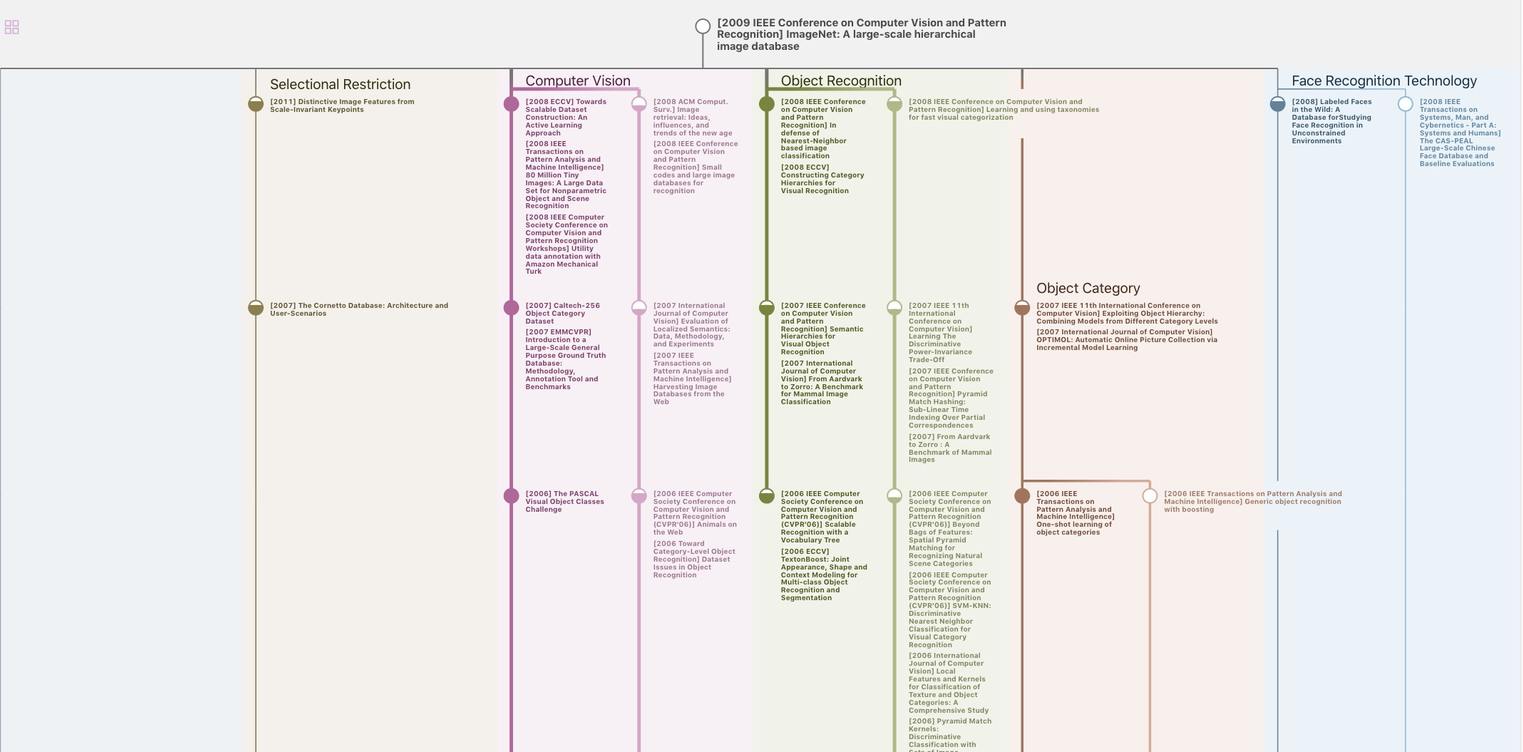
生成溯源树,研究论文发展脉络
Chat Paper
正在生成论文摘要