Optimal control of probabilistic Boolean control networks: A scalable infinite horizon approach
INTERNATIONAL JOURNAL OF ROBUST AND NONLINEAR CONTROL(2023)
摘要
One of the major issues in systems biology is developing control theory for gene regulatory networks (GRNs). Particularly, an important objective is to develop therapeutic intervention strategies to alter regulatory dynamics of GRNs to avoid undesired or diseased states. Several optimal control strategies have been developed to find therapeutic intervention strategies for small (or medium) sized GRNs modeled as probabilistic Boolean control networks (PBCNs). However, due to the humongous nature of GRNs, we require an optimal control strategy that scales to large systems without posing any constraints on the network dynamics. In this article, we formulate the infinite horizon discounted cost optimal control problem by leveraging the Markov decision process (MDP) based PBCN structure to model GRNs. Further, we design a stationary optimal control strategy, thereby avoiding the states associated with a disease. An augmented state space is defined to facilitate the control design method. By exploiting Kullback-Leibler (KL) divergence and the log transformation of the cost function referred to as the desirability function, the optimal control is devised using the path integral (PI) approach. We propose a sampling-based technique for the approximation of the PI and hence the optimal control of PBCNs. The sampling-based strategy is amicable to parallel implementation, thereby addressing the optimal control problem of large-scale PBCNs. Finally, the viability of the results in the article is shown by some illustrative examples.
更多查看译文
关键词
Kullback-Leibler control, Markov decision processes, optimal control, parallel processing, probabilistic Boolean control networks
AI 理解论文
溯源树
样例
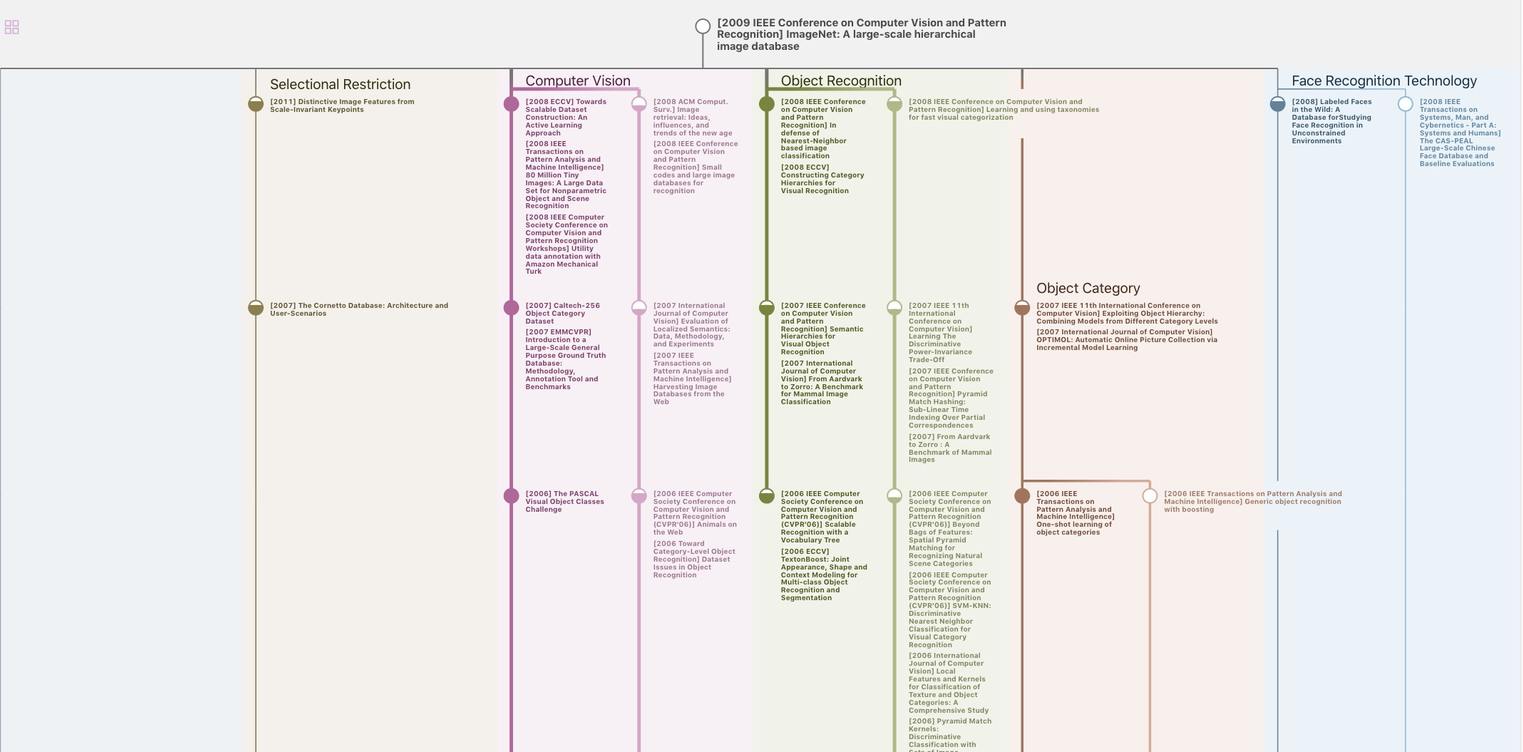
生成溯源树,研究论文发展脉络
Chat Paper
正在生成论文摘要