Functional principal component analysis estimator for non-Gaussian data
JOURNAL OF STATISTICAL COMPUTATION AND SIMULATION(2022)
摘要
Functional principal component analysis (FPCA) could become invalid when data involve non-Gaussian features. Therefore, we aim to develop a general FPCA method that can adapt to such non-Gaussian cases. A Kendall's tau function, which possesses identical eigenfunctions as covariance function, is constructed. The particular formulation of Kendall's tau function makes it less sensitive to data distribution. We further apply it to the estimation of FPCA and study the corresponding asymptotic consistency. Moreover, the effectiveness of the proposed method is demonstrated through a comprehensive simulation study and an application to the physical activity data that collected by a wearable accelerometer monitor.
更多查看译文
关键词
Functional data analysis, functional principal component analysis, non-Gaussian data, Kendall's tau function
AI 理解论文
溯源树
样例
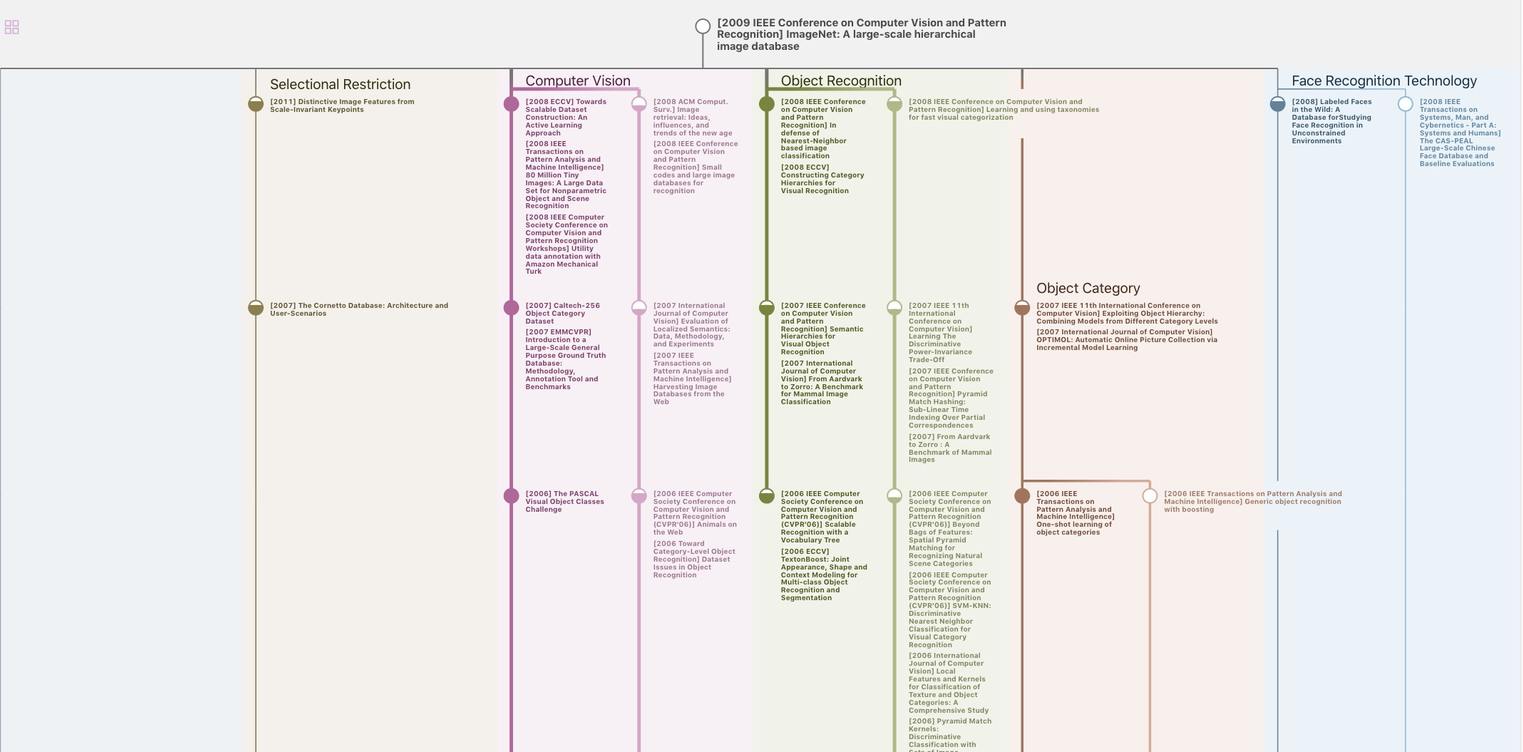
生成溯源树,研究论文发展脉络
Chat Paper
正在生成论文摘要