COVID-19 Face Mask Detection in a Crowd Using Multi-model Based on YOLOv3 and Hand-crafted Features
MULTIMODAL IMAGE EXPLOITATION AND LEARNING 2021(2021)
摘要
Face recognition is one of the most challenging biometric modalities when deployed in unconstrained environments due to the high variability that faces images present in the real world in a crowd, which are affected by complex factors including head poses, aging, illumination conditions, occlusions, and facial expressions. The face recognition system aims to identify and track visual data subjects, such as images and videos. More people are currently carrying masks in public, bringing new challenges to the face detection and identification system. This article focuses on the detection and recognition of masked faces. The presented framework is based on new artificial intelligence tools that use hand-crafted and deep learning (YOLOv3 and CNNs) features and SVM classifiers. Computer simulation on five different face mask datasets (Real-World Masked Face Dataset (RMFD), the Simulated Masked Face Dataset (SMFD), Medical Mask Dataset(MMD), Labeled Faces in the Wild (LFW)) and our proposed Artificially simulated masked face dataset (ASMFD), of which the testing results illustrate that the proposed method is comparable or better in most cases than traditional face mask recognition techniques. The presented system may produce anonymous statistical data that can help the agencies predict potential epidemics of COVID-19.
更多查看译文
关键词
COVID-19, Masked Face, Deep Learning, Machine Learning, Masked Face Detection
AI 理解论文
溯源树
样例
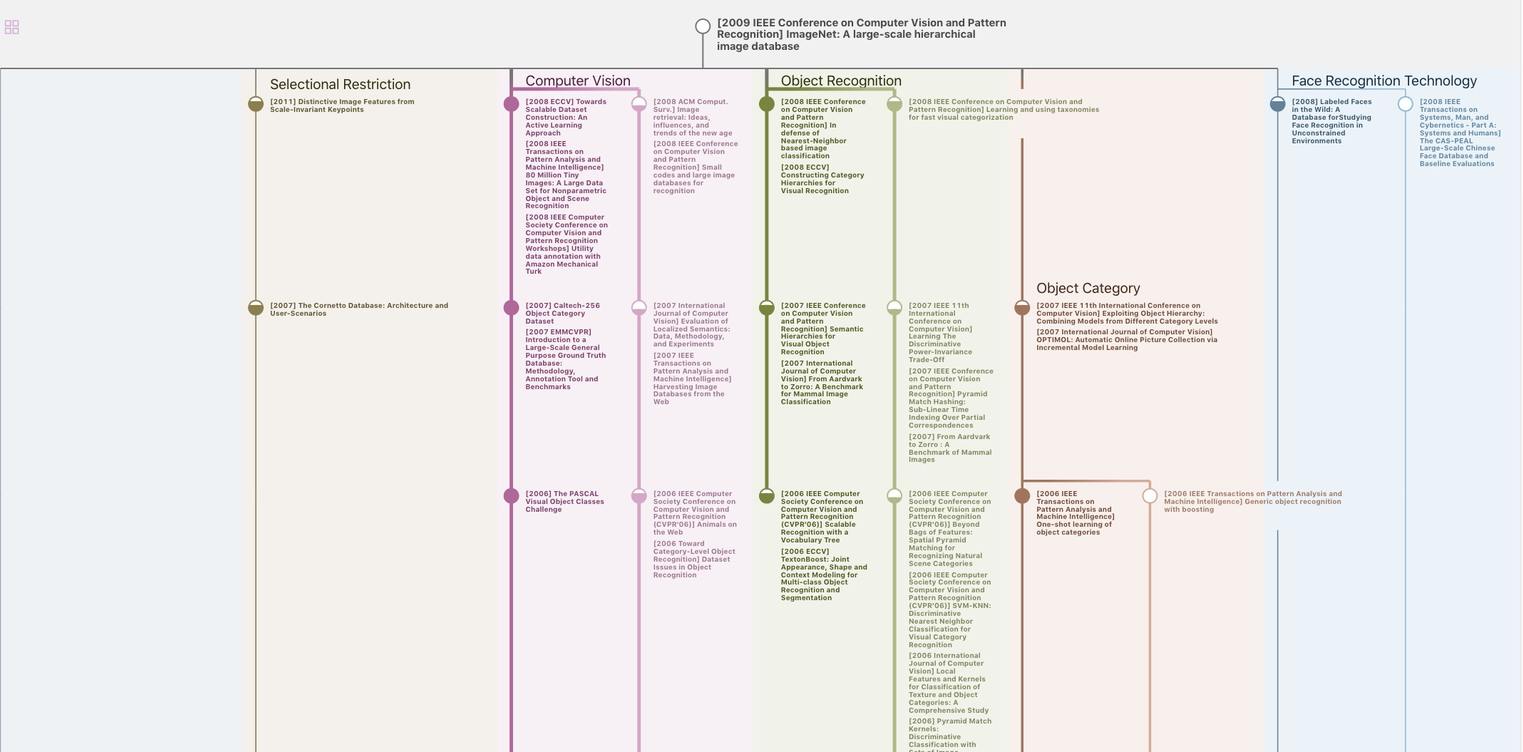
生成溯源树,研究论文发展脉络
Chat Paper
正在生成论文摘要