Global attention network for collaborative saliency detection
INTERNATIONAL JOURNAL OF MACHINE LEARNING AND CYBERNETICS(2022)
摘要
Collaborative saliency (cosaliency) detection aims to identify common and saliency objects or regions in a set of related images. The major challenge to address is how to extract useful information on single images and image groups to express collaborative saliency cues. In this paper, we propose a global attention network for cosaliency detection to extract individual features from the feature enhancement module (FEM). Then to capture useful global information, the global information module (GIM) is applied to all individual features to obtain individual cues, and finally, group collaborative cues are obtained by the collaboration correlation module (CCM). Specifically, the channel attention module and spatial attention module are plugged into the convolution feature network. To increase global context information, we perform global information module (GIM) on the preprocessed features and embed nonlocal modules in the backbone network and adopt global average pooling to extract global semantic representation vector as individual cues. Then, we build a collaborative correlation module (CCM) to extract collaborative and consistent information by calculating the correlation between the individual features of the input image and individual cues in the collaborative correlation module. We evaluate our method on two cosaliency detection benchmark datasets (CoSal2015, iCoSeg). Extensive experiments demonstrate the effectiveness of the proposed model, in most cases our method exceeds the state-of-the-art methods.
更多查看译文
关键词
Co-saliency,Collaborative correlation,Global information,Attention
AI 理解论文
溯源树
样例
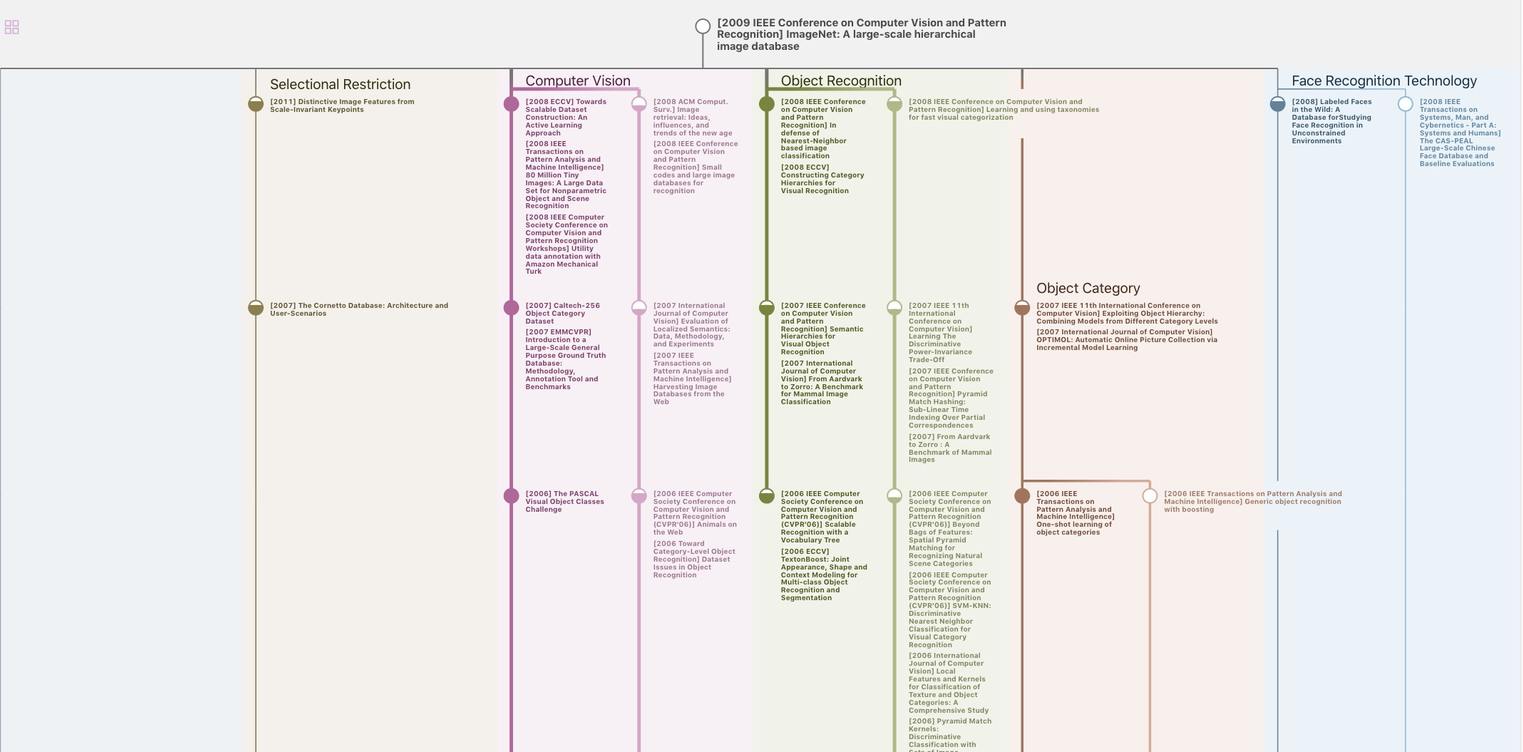
生成溯源树,研究论文发展脉络
Chat Paper
正在生成论文摘要