Dictionary learning for sparse representation of signals with hidden Markov model dependency
DIGITAL SIGNAL PROCESSING(2022)
摘要
The goal of dictionary learning algorithms is factorizing the matrix of training signals Y with K signals, into the dictionary matrix D and the coefficient matrix X which is a sparse matrix. The common approach among the algorithms is minimizing the representation error subject to the sparseness of X using alternation minimization method following 1) the sparsification and 2) the dictionary update steps. In this approach, when D is fixed and X must be estimated (the sparsification step), the minimization problem is divided to K different sparse recovery problems because the training signals are assumed to be independent. However, in some signals this assumption is not correct and the signals are not independent. Therefore, using the current strategy does not lead to the correct estimation of the parameters. The prominence is especially linked to medical signals and images such as electroencephalography (EEG) or diffusion weighted images, where the recordings do not constitute a matrix of independent training signals. In this study, we investigate the dictionary learning problem for sparse representation when there is hidden Markov model (HMM) dependency among the training signals. We propose an approach to improve the performance of the dictionary learning algorithms in the mentioned scenario. The proposed approach is not an independent dictionary learning algorithm. It is a general approach that we can employ for existing dictionary learning algorithms to improve their performance in learning from signals with HMM dependency. We confirm the efficiency of the proposed approach using simulations, and also, present a real application in medical signals for the considered scenario. (C)& nbsp;2022 Elsevier Inc. All rights reserved.
更多查看译文
关键词
Dictionary, Coefficient matrix, Sparse representation, Hidden Markov model, Transition probability matrix
AI 理解论文
溯源树
样例
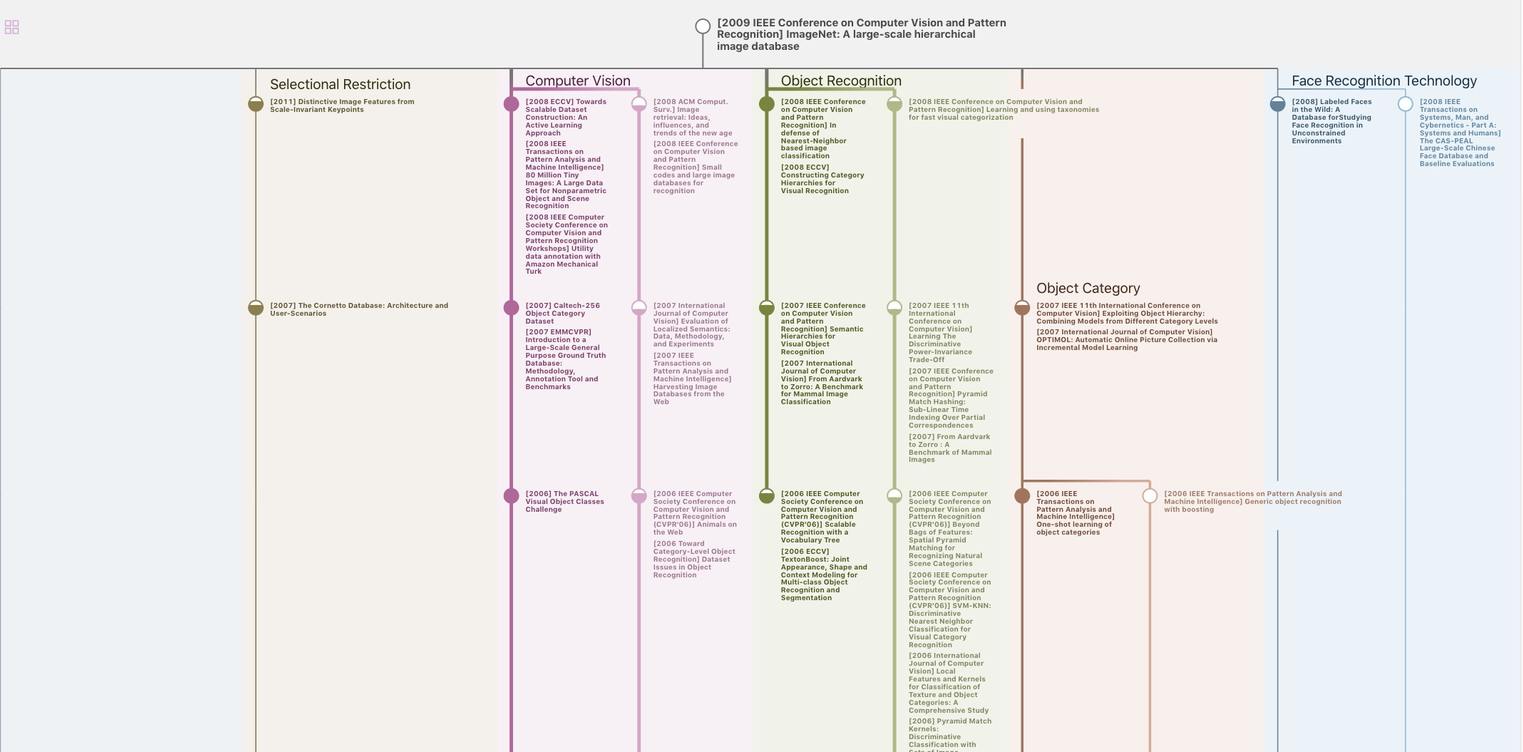
生成溯源树,研究论文发展脉络
Chat Paper
正在生成论文摘要