People detection with omnidirectional cameras using a spatial grid of deep learning foveatic classifiers
DIGITAL SIGNAL PROCESSING(2022)
摘要
A novel deep-learning people detection algorithm using omnidirectional cameras is presented, which only requires point-based annotations, unlike most of the prominent works that require bounding box annotations. Thus, the effort of manually annotating the needed training databases is significantly reduced, allowing a faster system deployment. The algorithm is based on a novel deep neural network architecture that implements the concept of Grid of Spatial-Aware Classifiers, but allowing end-to-end training that improves the performance of the whole system. The designed algorithm satisfactorily handles the severe geometric distortions of the omnidirectional images, which typically degrades the performance of state-of-the-art detectors, without requiring any camera calibration. The algorithm has been evaluated in well-known omnidirectional image databases (PIROPO, BOMNI, and MW-18Mar) and compared with several works of the state of the art.(c) 2022 The Author(s). Published by Elsevier Inc.& nbsp;
更多查看译文
关键词
Spatial grid, Deep learning, Omnidirectional cameras, People detection, Point based annotations
AI 理解论文
溯源树
样例
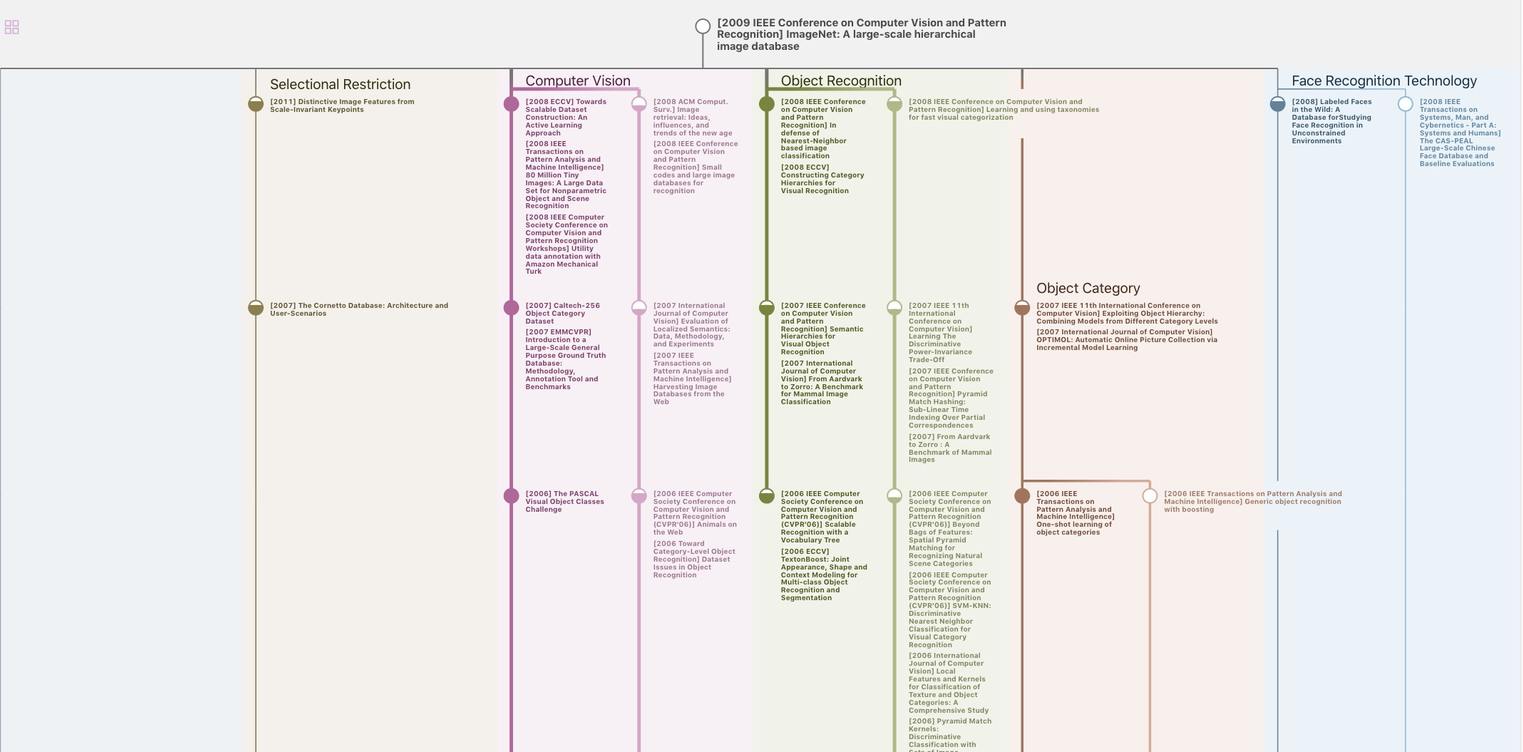
生成溯源树,研究论文发展脉络
Chat Paper
正在生成论文摘要