Prediction of heavy rainfall days over a peninsular Indian station using the machine learning algorithms
JOURNAL OF EARTH SYSTEM SCIENCE(2021)
摘要
dvance prediction of heavy rainfall days over a given location is of paramount importance as heavy rainfall impacts ecosystems, leads to floods, accounts largely for the total rainfall over the region and its prediction is highly desired for the efficient management of weather-dependent activities. Traditionally, Numerical Weather Prediction models serve the purpose of weather predictions, but they have their constraints and limitations. In this regard, artificial intelligence and machine learning tools have gained popularity in recent years. In the present study, we have employed the Gaussian Process Regression (GPR) approach, one of the machine learning methods, on a long time-series rainfall data for the determination of heavy and light rainfall days. Climatological data of daily rainfall for a period of 116 years from 1901 to 2016 over Sriharikota (13.66°N, 80.23°E), a coastal island location in India, is used for training the GPR model for the identification of the heavy and light category of rainy days. The performance of the GPR model is investigated by predicting the heavy and light rainfall days per year over Sriharikota. K-nearest neighbour, random forest, and decision tree models are also used and results are compared. The validation of GPR results shows that the performance of the proposed model is satisfying (root mean square error = 0.161; mean absolute error = 0.126; mean squared error = 0.026), especially for the heavy rainfall days. Furthermore, GPR model is extended to prediction of spatial distribution of monthly rainfall over the Indian region. Results obtained from the present study encourages the utilization of the GPR model as one of the promising machine learning tools for the prediction of heavy rainfall days over a given location.
更多查看译文
关键词
Heavy rainfall, light rainfall, machine learning, Gaussian process regression
AI 理解论文
溯源树
样例
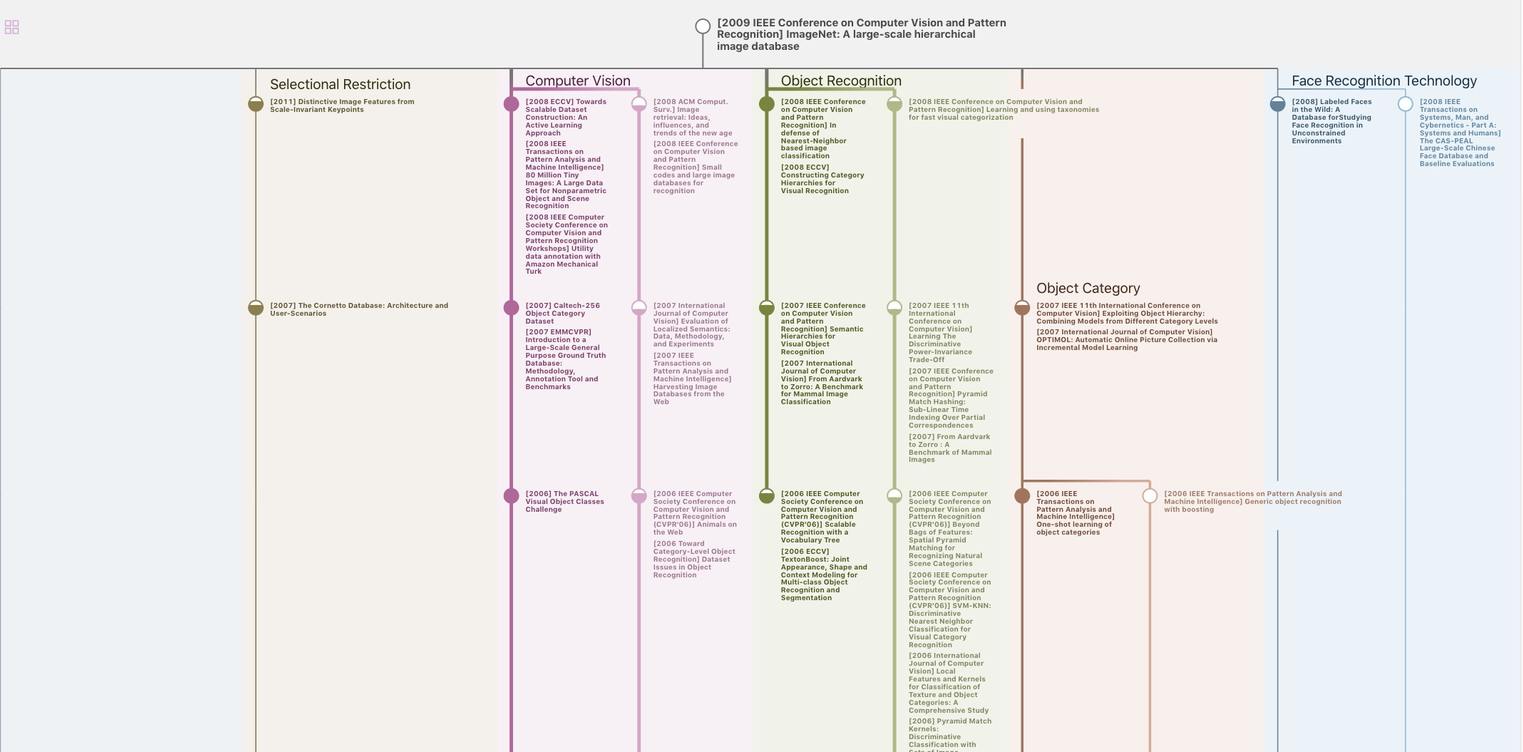
生成溯源树,研究论文发展脉络
Chat Paper
正在生成论文摘要