Evaluating Association Between Two Event Time Observations Subject to informative Censoring
JOURNAL OF THE AMERICAN STATISTICAL ASSOCIATION(2023)
摘要
This article is concerned with evaluating the association between two event times without specifying the joint distribution parametrically. This is particularly challenging when the observations on the event times are subject to informative censoring due to a terminating event such as death. There are few methods suitable for assessing covariate effects on association in this context. We link the joint distribution of the two event times and the informative censoring time using a nested copula function. We use flexible functional forms to specify the covariate effects on both the marginal and joint distributions. In a semiparametric model for the bivariate event time, we estimate simultaneously the association parameters, the marginal survival functions, and the covariate effects. A byproduct of the approach is a consistent estimator for the induced marginal survival function of each event time conditional on the covariates. We develop an easy-to-implement pseudolikelihood-based inference procedure, derive the asymptotic properties of the estimators, and conduct simulation studies to examine the finite-sample performance of the proposed approach. For illustration, we apply our method to analyze data from the breast cancer survivorship study that motivated this research. Supplementary materials for this article are available online.
更多查看译文
关键词
Association parameter, Copula model, Marginal distribution, Pseudolikelihood estimation, Semiparametric modeling
AI 理解论文
溯源树
样例
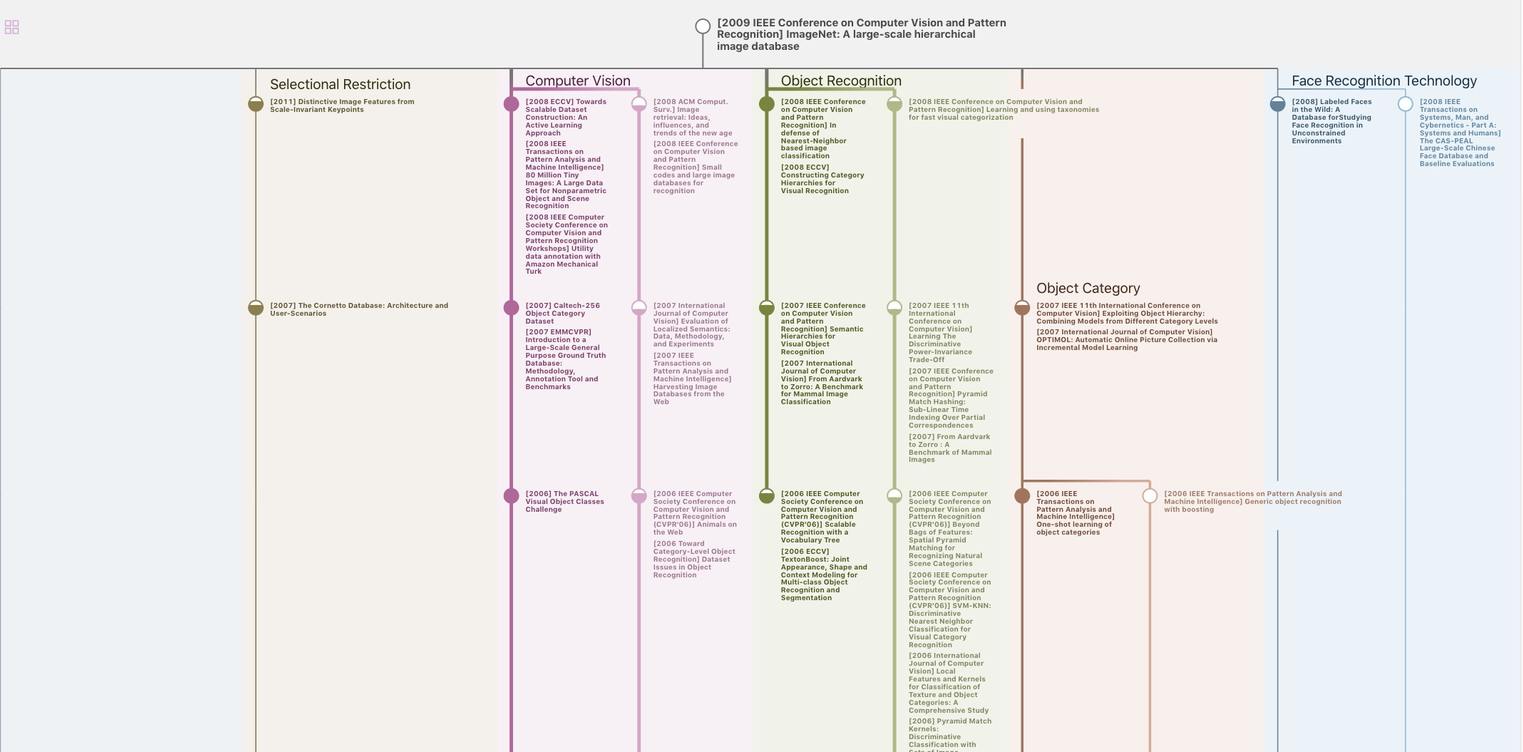
生成溯源树,研究论文发展脉络
Chat Paper
正在生成论文摘要