Data Screening Based on Correlation Energy Fluctuation Coefficient and Deep Learning for Fault Diagnosis of Rolling Bearings
ENERGIES(2022)
摘要
The accuracy of the intelligent diagnosis of rolling bearings depends on the quality of its vibration data and the accuracy of the state identification model constructed accordingly. Aiming at the problem of "poor quality" of data and "difficult to select" structural parameters of the identification model, a method is proposed to integrate data cleaning in order to select effective learning samples and optimize the selection of the structural parameters of the deep belief network (DBN) model. First, by calculating the relative energy fluctuation value of the finite number of intrinsic function components using the variational modal decomposition of the rolling bearing vibration data, the proportion of each component containing the fault component is characterized. Then, high-quality learning samples are obtained through screening and reconstruction to achieve the effective cleaning of vibration data. Second, the improved particle swarm algorithm (IPSO) is used to optimize the number of nodes in each hidden layer of the DBN model in order to obtain the optimal structural parameters of the intelligent diagnosis model. Finally, the high-quality learning samples obtained from data cleaning are used as input to construct an intelligent identification model for rolling bearing faults. The results showed that the proposed method not only screens out the intrinsic mode function components that contain the fault effective components in the rolling bearing vibration data, but also finds the optimal solution for the number of nodes in the DBN hidden layer, which improves bearing state identification accuracy by 3%.
更多查看译文
关键词
learning sample screening, correlation energy fluctuation coefficient, rolling bearing, DBN optimized by IPSO, fault recognition rate
AI 理解论文
溯源树
样例
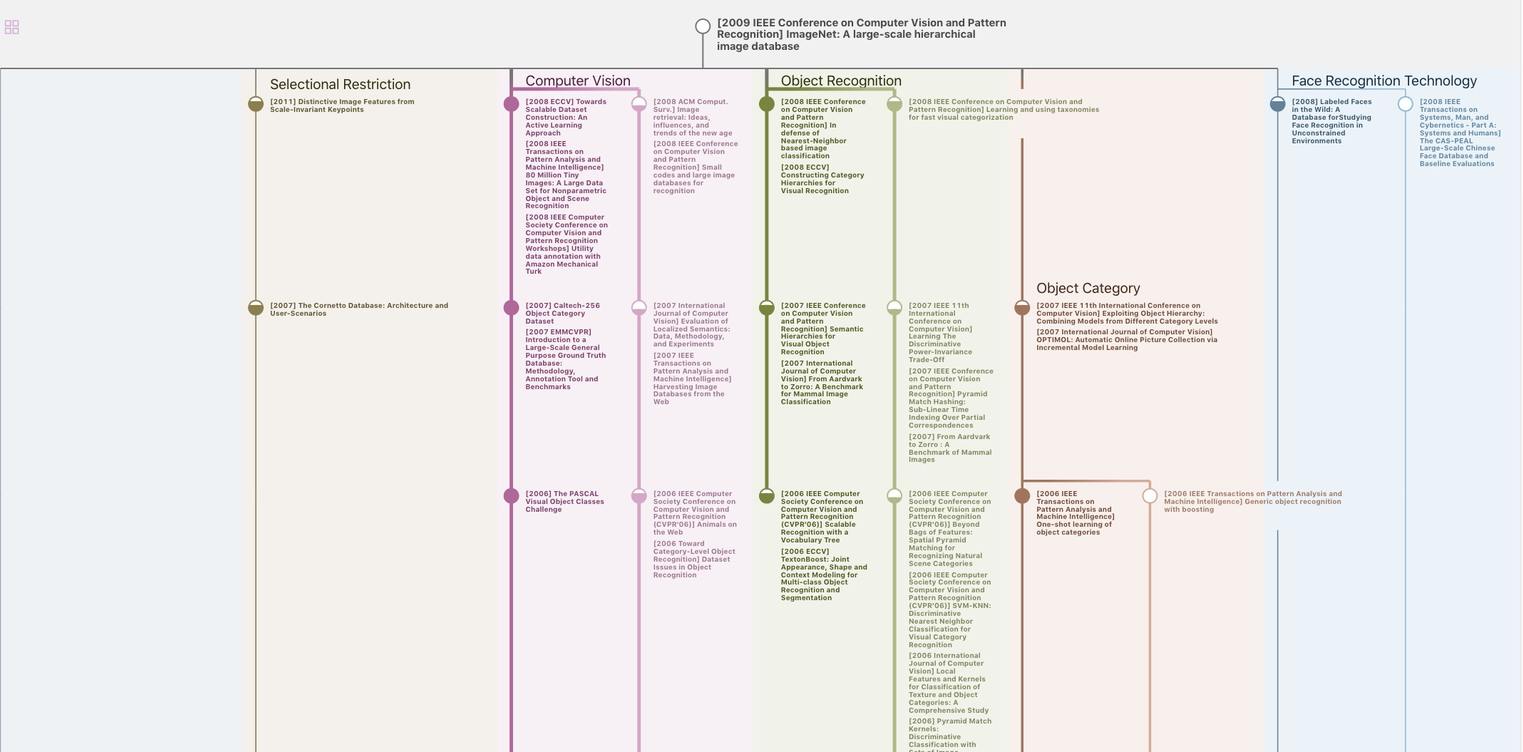
生成溯源树,研究论文发展脉络
Chat Paper
正在生成论文摘要