Multi-Class Classifier in Parkinson's Disease Using an Evolutionary Multi-Objective Optimization Algorithm
APPLIED SCIENCES-BASEL(2022)
摘要
In this contribution, a novel methodology for multi-class classification in the field of Parkinson's disease is proposed. The methodology is structured in two phases. In a first phase, the most relevant volumes of interest (VOI) of the brain are selected by means of an evolutionary multi-objective optimization (MOE) algorithm. Each of these VOIs are subjected to volumetric feature extraction using the Three-Dimensional Discrete Wavelet Transform (3D-DWT). When applying 3D-DWT, a high number of coefficients is obtained, requiring the use of feature selection/reduction algorithms to find the most relevant features. The method used in this contribution is based on Mutual Redundancy (MI) and Minimum Maximum Relevance (mRMR) and PCA. To optimize the VOI selection, a first group of 550 MRI was used for the 5 classes: PD, SWEDD, Prodromal, GeneCohort and Normal. Once the Pareto Front of the solutions is obtained (with varying degrees of complexity, reflected in the number of selected VOIs), these solutions are tested in a second phase. In order to analyze the SVM classifier accuracy, a test set of 367 MRI was used. The methodology obtains relevant results in multi-class classification, presenting several solutions with different levels of complexity and precision (Pareto Front solutions), reaching a result of 97% as the highest precision in the test data.
更多查看译文
关键词
Parkinson's disease (PD), 3D-discrete wavelet transform (3D-DWT), support vector machine (SVM), multi-objective optimization evolutionary algorithm (MOE), minimum redundancy maximum relevance (mRMR)
AI 理解论文
溯源树
样例
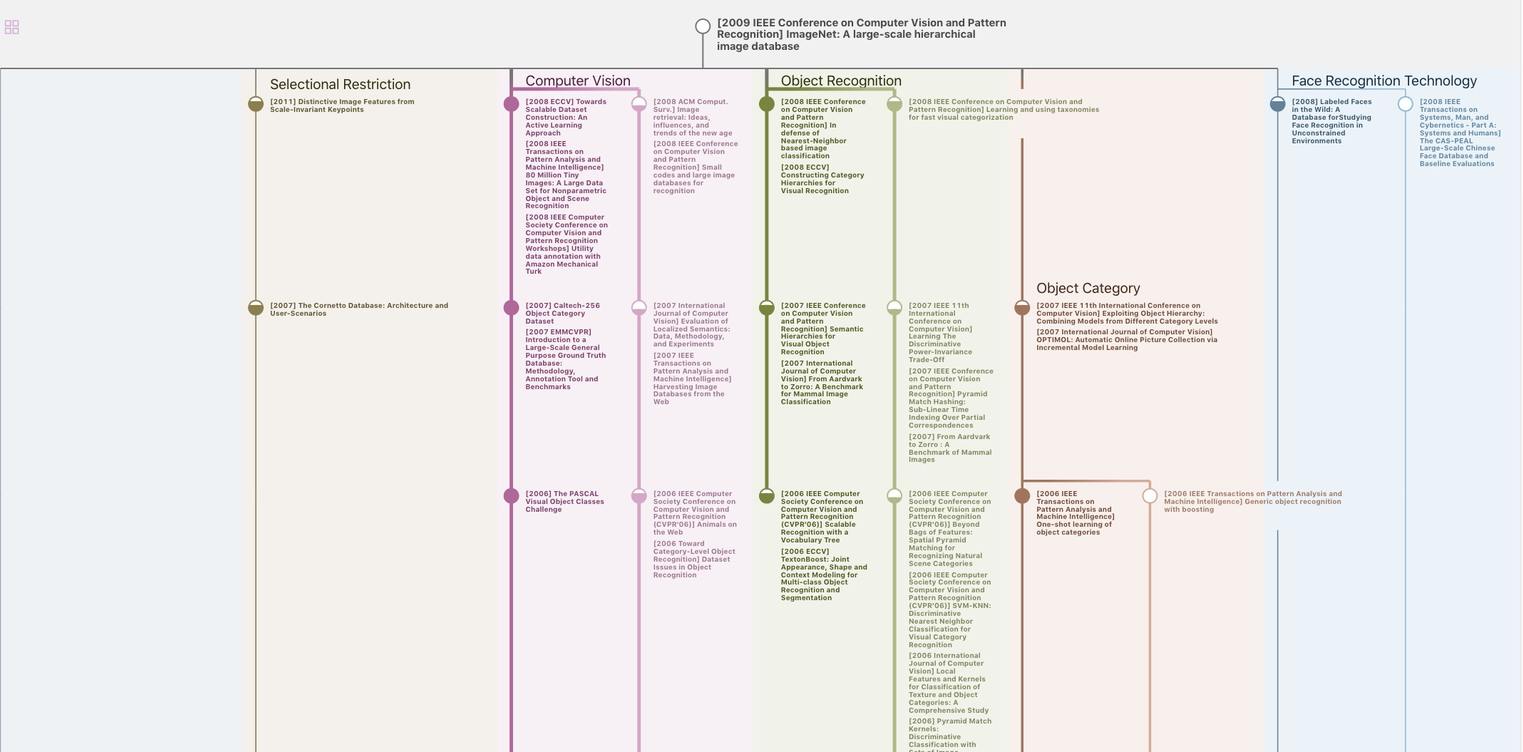
生成溯源树,研究论文发展脉络
Chat Paper
正在生成论文摘要