Smart fault detection and optimal variables identification using Kernel Mahalanobis Distance for industrial manufacturing processes
INTERNATIONAL JOURNAL OF COMPUTER INTEGRATED MANUFACTURING(2022)
摘要
The development of a new methodology for the accurate fault detection considering only those really important features of practical impact is the main motivation of this work. Therefore, the Kernel-based Mahalanobis-Taguchi System as a pre-classification stage followed by a binary particle swarm optimization procedure is introduced in this work in order to identify variables of impact by means of a feature screening in an optimal manner for industrial manufacturing processes. In order to study the practical efficiency of the presented approach, a computational comparison between the introduced approach and the standard Mahalanobis-Taguchi System is reported. Furthermore to test the relevance of the selected features by the proposed fault diagnosis approach, the sequential feature selection (SFS) and Random Forest (RF) algorithms are implemented and five experiments were carried out training a total of 15 machine learning algorithms. The presented approach is applied to foam injection and welding processes from the manufacturing automotive industry showing that the presented approach can achieve betters accuracy values for fault detection by selecting a small subset of features than the other presented feature selection techniques.
更多查看译文
关键词
Kernel Mahalanobis distance, Fault classification, Variable identification, Manufacturing Processes
AI 理解论文
溯源树
样例
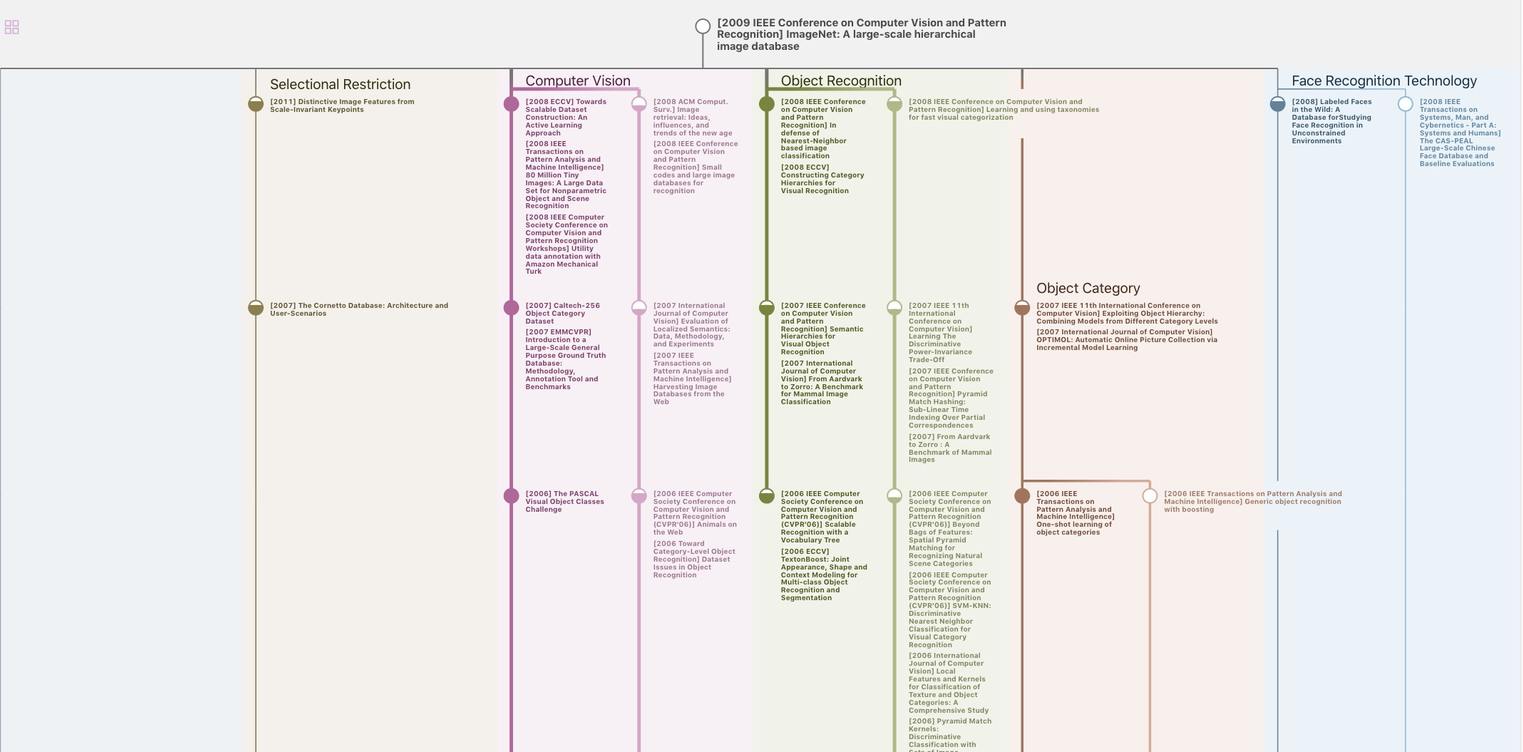
生成溯源树,研究论文发展脉络
Chat Paper
正在生成论文摘要