Autonomous Detection of Spodoptera frugiperda by Feeding Symptoms Directly from UAV RGB Imagery
APPLIED SCIENCES-BASEL(2022)
摘要
The use of digital technologies to detect, position, and quantify pests quickly and accurately is very important in precision agriculture. Imagery acquisition using air-borne drones in combination with the deep learning technique is a new and viable solution to replace human labor such as visual interpretation, which consumes a lot of time and effort. In this study, we developed a method for automatic detecting an important maize pest-Spodoptera frugiperda-by its gnawing holes on maize leaves based on convolution neural network. We validated the split-attention mechanism in the classical network structure ResNet50, which improves the accuracy and robustness, and verified the feasibility of two kinds of gnawing holes as the identification features of Spodoptera frugiperda invasion and the degree. In order to verify the robustness of this detection method against plant morphological changes, images at the jointing stage and heading stage were used for training and testing, respectively. The performance of the models trained with the jointing stage images has been achieved the validation accuracy of ResNeSt50, ResNet50, EfficientNet, and RegNet at 98.77%, 97.59%, 97.89%, and 98.07%, with a heading stage test accuracy of 89.39%, 81.88%, 86.21%, and 84.21%.
更多查看译文
关键词
Spodoptera frugiperda, deep learning, convolutional neural network, corn insect
AI 理解论文
溯源树
样例
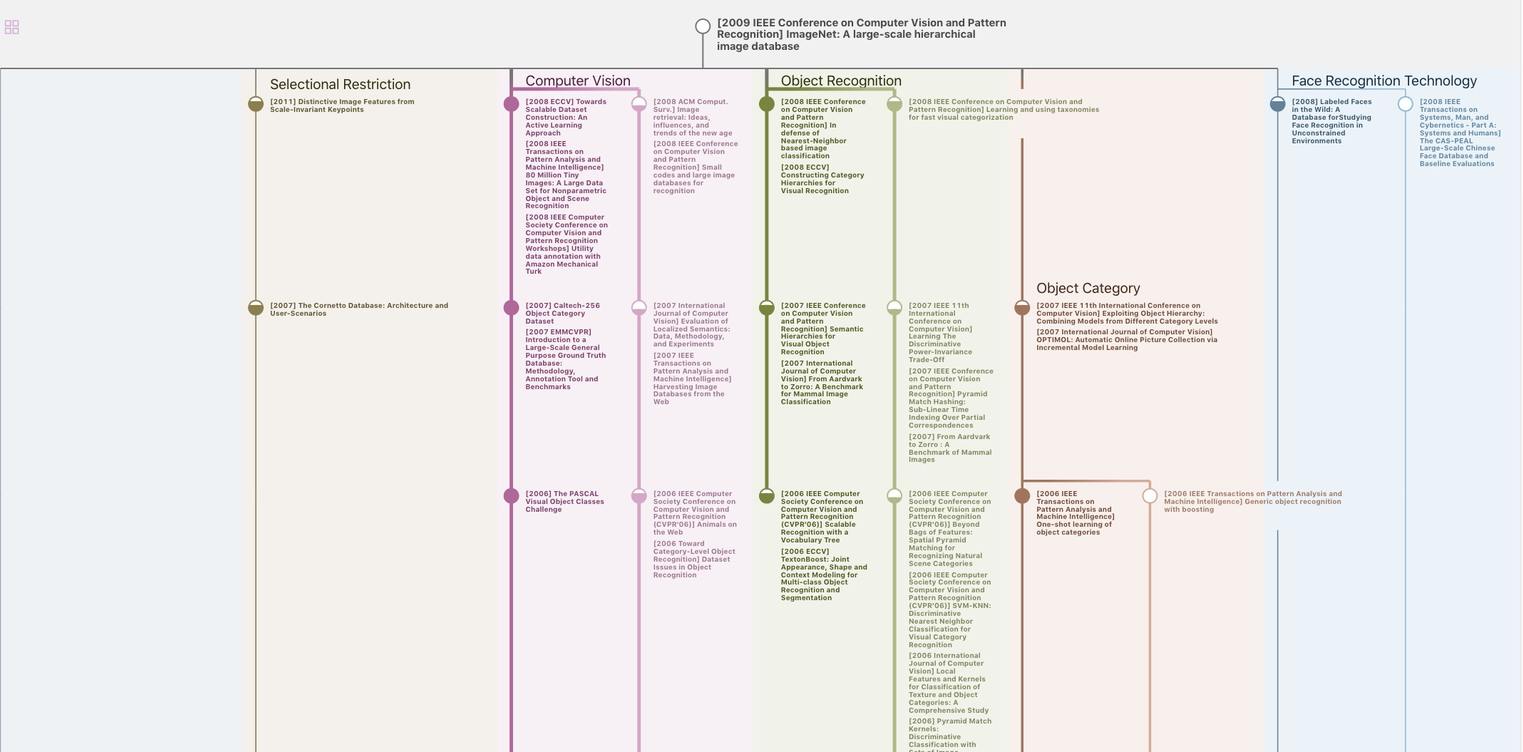
生成溯源树,研究论文发展脉络
Chat Paper
正在生成论文摘要