CrimeForecaster: Crime Prediction by Exploiting the Geographical Neighborhoods' Spatiotemporal Dependencies
MACHINE LEARNING AND KNOWLEDGE DISCOVERY IN DATABASES: APPLIED DATA SCIENCE AND DEMO TRACK, ECML PKDD 2020, PT V(2021)
摘要
Crime prediction in urban areas can improve the allocation of resources (e.g., police patrols) towards a safer society. Recently, researchers have been using deep learning frameworks for urban crime forecasting with better accuracies as compared to previous work. However, these studies typically partition a metropolitan area into synthetic regions, e.g., grids, which neglects the geographical semantics of a region, nor captures the spatial correlation across the regions, e.g., precincts, neighborhoods, blocks, and postal division. In this paper, we design and implement an end-to-end spatiotemporal deep learning framework, dubbed CrimeForecaster, which captures both the temporal recurrence and the spatial dependency simultaneously within and across regions. We model temporal dependencies by using the Gated Recurrent Network with Diffusion Convolution modules to capture the cross-region dependencies at the same time. Empirical experiments on two real-world datasets showcase the effectiveness of CrimeForecaster, where CrimeForecaster outperforms the current state-of-the-art algorithm by up to 21%. We also collect and publish a ten-year crime dataset in Los Angeles for future use by the research community.
更多查看译文
关键词
Social good, Crime precition, Spatiotemporal learning
AI 理解论文
溯源树
样例
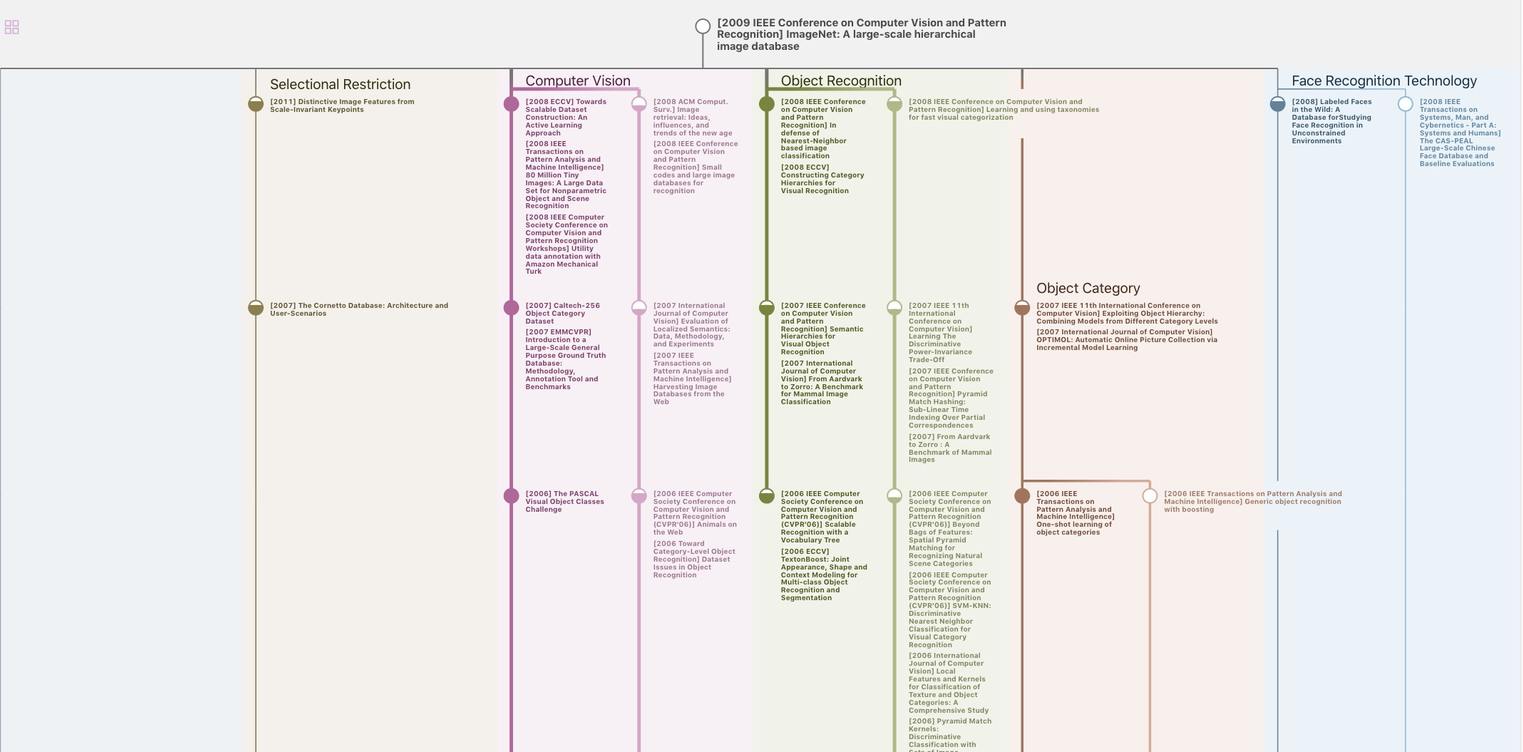
生成溯源树,研究论文发展脉络
Chat Paper
正在生成论文摘要