PS3: Partition-Based Skew-Specialized Sampling for Batch Mode Active Learning in Imbalanced Text Data
MACHINE LEARNING AND KNOWLEDGE DISCOVERY IN DATABASES: APPLIED DATA SCIENCE AND DEMO TRACK, ECML PKDD 2020, PT V(2021)
摘要
While social media has taken a fixed place in our daily life, its steadily growing prominence also exacerbates the problem of hostile contents and hate-speech. These destructive phenomena call for automatic hate-speech detection, which, however, is facing two major challenges, namely i) the dynamic nature of online content causing significant data-drift over time, and ii) a high class-skew, as hate-speech represents a relatively small fraction of the overall online content. The first challenge naturally calls for a batch mode active learning solution, which updates the detection system by querying human domain-experts to annotate meticulously selected batches of data instances. However, little prior work exists on batch mode active learning with high class-skew, and in particular for the problem of hate-speech detection. In this work, we propose a novel partition-based batch mode active learning framework to address this problem. Our framework falls into the so-called screening approach, which pre-selects a subset of most uncertain data items and then selects a representative set from this uncertainty space. To tackle the classs-kew problem, we use a data-driven skew-specialized cluster representation, with a higher potential to "cherry pick" minority classes. In extensive experiments we demonstrate substantial improvements in terms of G-Means, and F1 measure, over several baseline approaches and multiple datasets, for highly imbalanced class ratios.
更多查看译文
关键词
Batch-mode active learning, Imbalance data, Hate-speech recognition
AI 理解论文
溯源树
样例
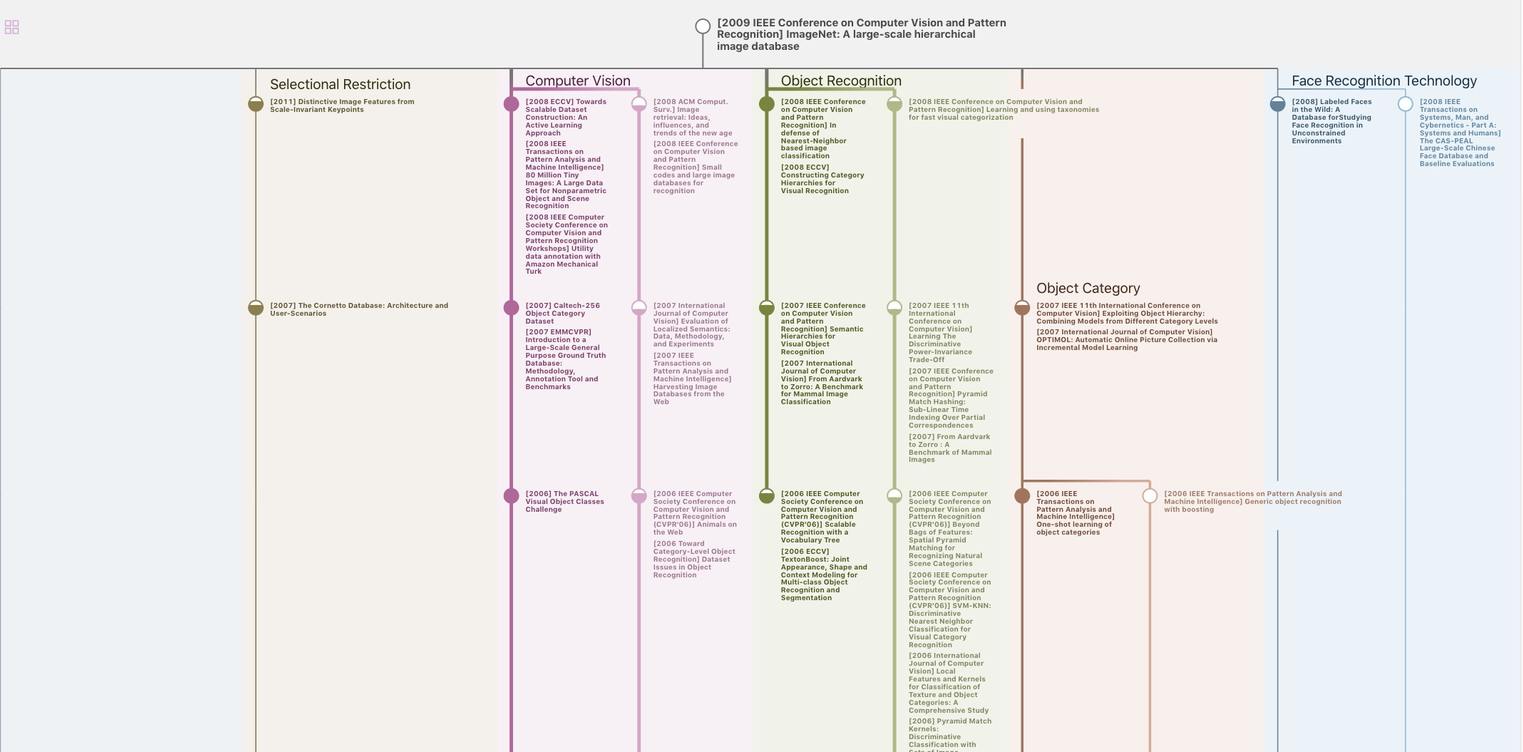
生成溯源树,研究论文发展脉络
Chat Paper
正在生成论文摘要