Benchmarking the performance of homogenization algorithms on synthetic daily temperature data
INTERNATIONAL JOURNAL OF CLIMATOLOGY(2022)
摘要
This paper describes a new homogenization algorithm validation methodology, and its use to assess the skill of eight different algorithms, when applied to synthetic daily temperature time series. These algorithms were ACMANT, Climatol (in both daily and monthly configurations), DAP, HOM, MAC-D, MASH and SpliDHOM. Algorithms were tested on benchmark data replicating daily temperature variability in four regions in North America: Wyoming, the South East, the North East and the South West. These benchmarks contained plausible spatial and temporal correlation, differing station densities and both abrupt and gradual inhomogeneities. Algorithm ability was assessed both according to detection of inhomogeneities and correction of their effects, investigating bias, root-mean-square error (RMSE), linear trend recovery, station extremes and variability recovery. Inhomogeneities with a magnitude greater than 1 degrees C were those most commonly detected with smaller inhomogeneities, and those that were not constant over time, proving harder to identify. Regional RMSE was always reduced by all algorithms and regional bias was reduced in over half of the region/scenario pairs. Trend recovery was variable, but the correct sign of regional trends was retained by all algorithms. Areas for future algorithm improvement include working with autocorrelated data and correcting moments higher than the mean. The data are available from and the validation code from allowing the extension of this work and its application to new algorithms.
更多查看译文
关键词
benchmarking, daily temperature, homogenization, synthetic data
AI 理解论文
溯源树
样例
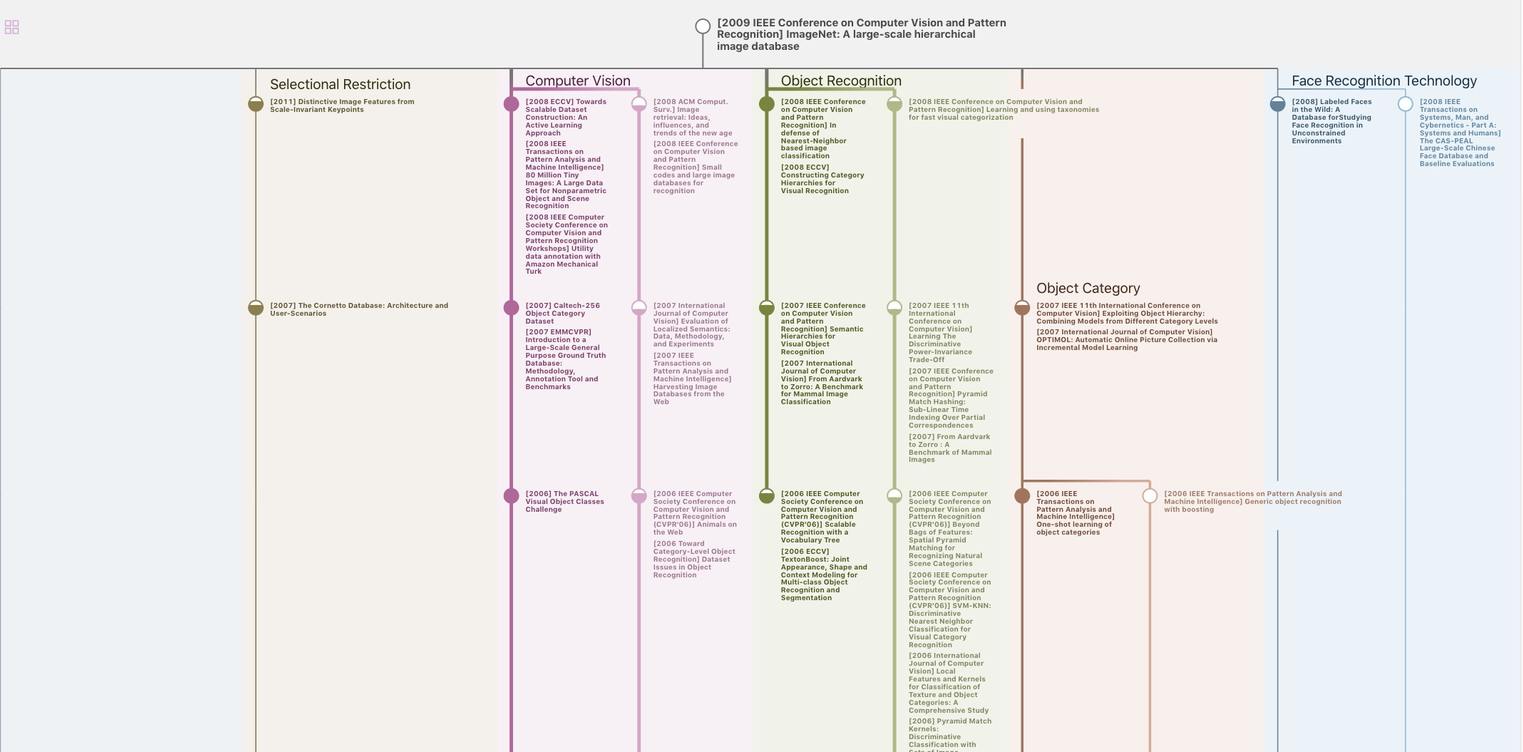
生成溯源树,研究论文发展脉络
Chat Paper
正在生成论文摘要