Multi WGAN-GP loss for pathological stain transformation using GAN
2021 29TH IRANIAN CONFERENCE ON ELECTRICAL ENGINEERING (ICEE)(2021)
摘要
In this paper, we proposed a new loss function to train the conditional generative adversarial network (CGAN). CGANs use a condition to generate images. Adding a class condition to the discriminator helps improve the training process of GANs and has been widely used for CGAN. Therefore, many loss functions have been proposed for the discriminator to add class conditions to it. Most of them have the problem of adjusting weights. This paper presents a simple yet new loss function that uses class labels, but no adjusting is required. This loss function is based on WGAN-GP loss, and the discriminator has outputs of the same order (the reason for no adjusting). More specifically, the discriminator has K (the number of classes) outputs, and each of them is used to compute the distance between fake and real samples of one class. Another loss to enable the discriminator to classify is also proposed by applying SoftMax to the outputs and adding cross-entropy to our first loss. The proposed loss functions are applied to a CGAN for image-to-image translation (here stain transformation for pathological images). The performances of proposed losses with some state-of-the-art losses are compared using Histogram Intersection Score between generated images and target images. The accuracy of a classifier is also computed to measure the effect of stain transformation. Our first loss performs almost similar to the loss that achieved the best results.
更多查看译文
关键词
conditional generative adversarial network, Wasserstien distance, WGAN-GP loss, stain transformation, pathology
AI 理解论文
溯源树
样例
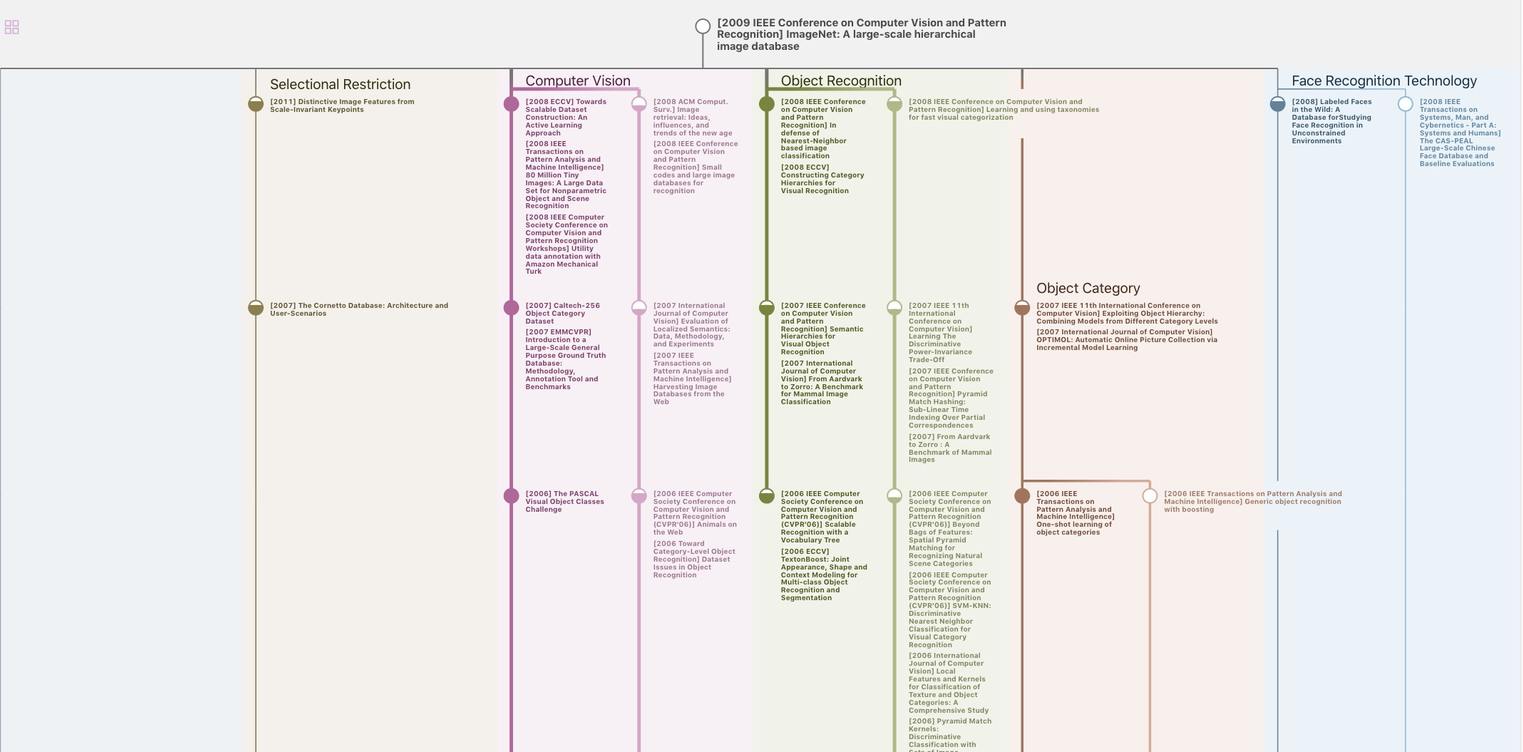
生成溯源树,研究论文发展脉络
Chat Paper
正在生成论文摘要