A combined traffic flow forecasting model based on graph convolutional network and attention mechanism
INTERNATIONAL JOURNAL OF MODERN PHYSICS C(2021)
摘要
Accurate traffic flow forecasting is a prerequisite guarantee for the realization of intelligent transportation, but due to the complex spatiotemporal characteristics of traffic flow, its forecasting has always been difficult. Deep learning can learn the deep spatiotemporal characteristics of traffic flow from a large amount of data. Deep learning can learn the deep spatiotemporal characteristics of traffic flow from a large amount of data. This paper establishes a novel combination forecasting model GGCN-SA based on deep learning for traffic flow to effectively capture the spatiotemporal characteristics of traffic flow and improve forecasting accuracy. The model captures the spatial correlation of the road traffic network through the graph convolutional network (GCN), captures the time dependence of the traffic flow through the gated recursive unit (GRU), and further introduces the soft attention mechanism (Soft Attention) to aggregate different neighborhoods Spatio-temporal information within the range to enhance the model's ability to characterize the temporal and spatial characteristics of traffic flow. A large number of experiments have been conducted on the METR-LA and SZ-taxi data sets. The experimental results show that the GGCN-SA model proposed in this paper has better forecasting performance compared with the baseline methods.
更多查看译文
关键词
Traffic flow forecasting, deep learning, attention mechanism, graph convolutional network, spatiotemporal characteristics
AI 理解论文
溯源树
样例
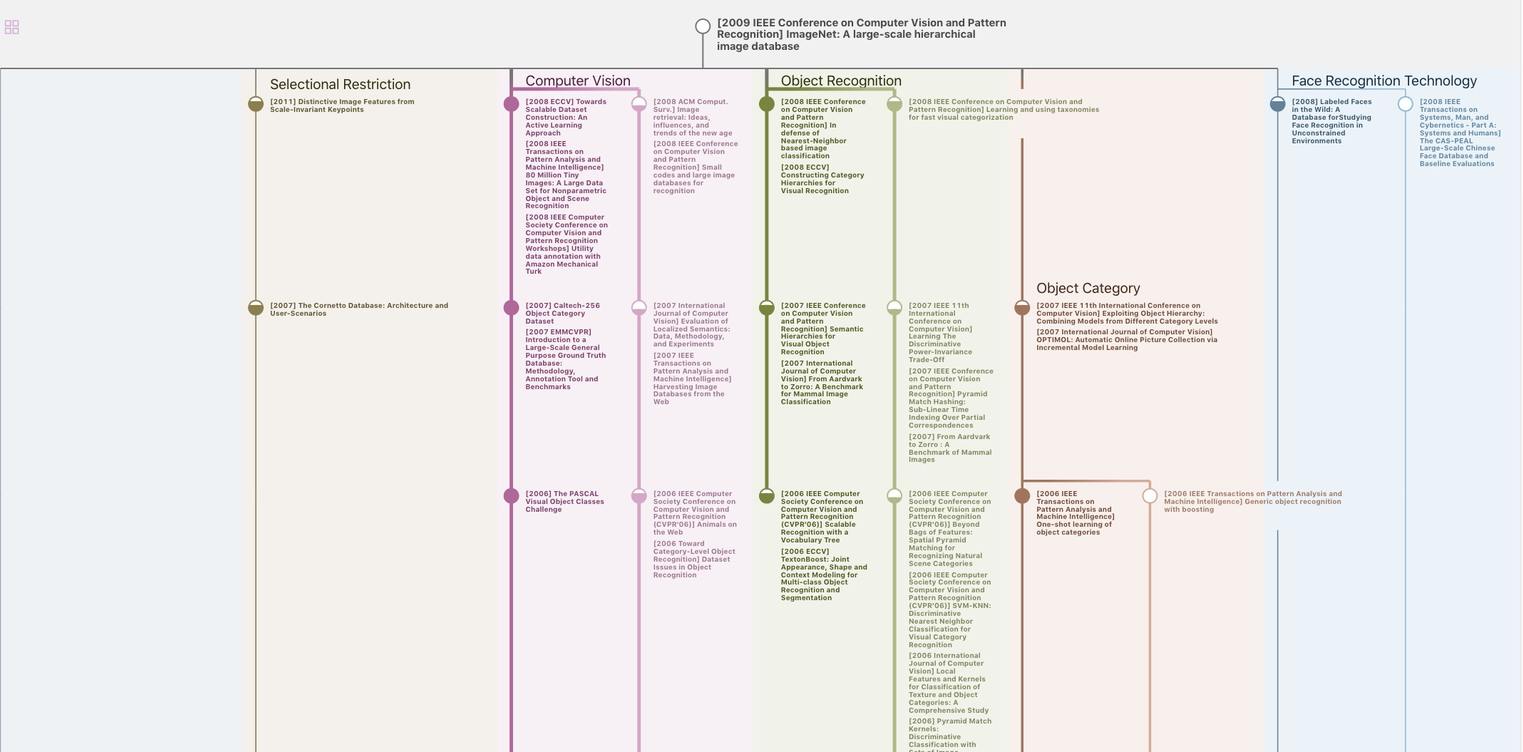
生成溯源树,研究论文发展脉络
Chat Paper
正在生成论文摘要