Audio-based expansion learning for aerial target recognition
Applied Acoustics(2022)
摘要
Data has become the fundamental element for most of audio-based recognition tasks, especially for aerial target recognition schemes. Actually, the lack of aerial target data has been one of great barriers that restrict improvement of recognition performance. In this paper, an expansion learning method was proposed to effectively expend the data set including environmental information of aerial targets. WaveNet is used as a generator for aerial target audio. Multi-layer feature space including three common features and three specific features was proposed to give an intuitive verification to the expansion learning method. Expansion learning can be evaluated by adding the generated samples to dataset. The expansion learning is proved to significantly improve the recognition performance. When mixing the raw audio and generated audio at a ratio of four to one, we achieve a performance with accuracy up to 97.00% and 99.50% respectively on the test sets composed of the raw data and the generated data.
更多查看译文
关键词
Aerial target recognition,Expansion learning,Multi-layer feature space,Convolutional neural networks
AI 理解论文
溯源树
样例
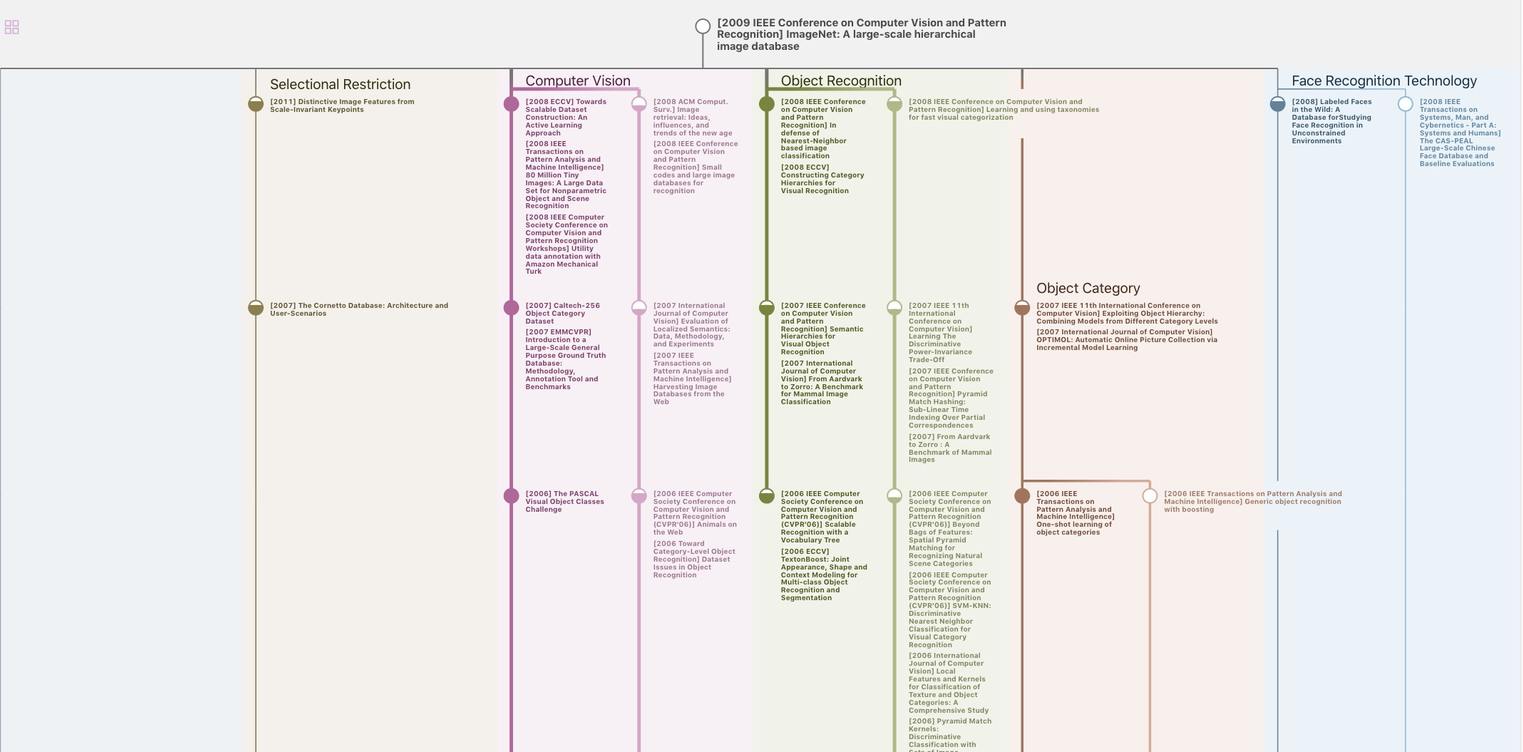
生成溯源树,研究论文发展脉络
Chat Paper
正在生成论文摘要