Neural network adaptive sliding mode control without overestimation for a maglev system
Mechanical Systems and Signal Processing(2022)
摘要
This study focuses on the high-performance control of a reluctance-motor maglev system (RMMS), while strong uncertainty brings great difficulties. As an effective robust control method, sliding mode control (SMC) suffers from chattering which brings challenges to practical applications. To overcome it, a novel neural network adaptive sliding mode control (NNASMC) method is proposed, which combines a new radial-basis-function-neural-network (RBFNN) compensator with a new adaptive sliding mode controller. Different from the existing results, this method not only compensates for the uncertainty, but also avoid overestimating the switching gain. Moreover, the controller, based on a generalized-form switching gain, needs no prior knowledge of the uncertainty. The stability of NNASMC is analyzed by a Lyapunov function. Through experiment and simulation, NNASMC is compared with SMC and an SMC with a double hidden layer recurrent neural network (SMC-DHLRNN) method. The necessities of compensator and adaptive switch gain are verified by simulation. Experimental results show that NNASMC reduces chattering significantly, achieves high-accuracy control of an RMMS, and has good disturbance-rejection ability.
更多查看译文
关键词
Maglev system,Reluctance motor,Radial basis function neural network,Adaptive sliding mode control
AI 理解论文
溯源树
样例
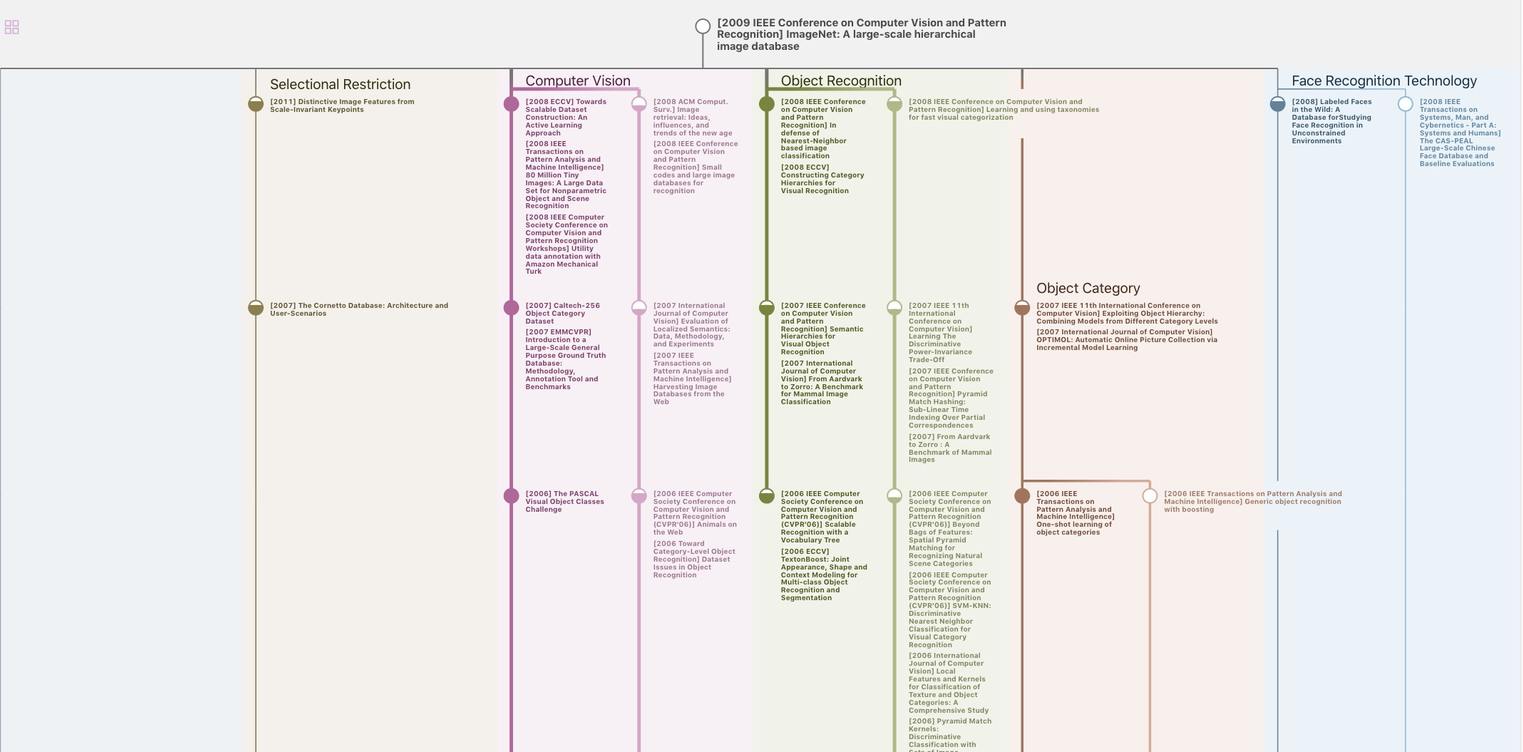
生成溯源树,研究论文发展脉络
Chat Paper
正在生成论文摘要