Autonomous navigation at unsignalized intersections: A coupled reinforcement learning and model predictive control approach
Transportation Research Part C: Emerging Technologies(2022)
摘要
This paper develops an integrated safety-enhanced reinforcement learning (RL) and model predictive control (MPC) framework for autonomous vehicles (AVs) to navigate unsignalized intersections. Researchers have extensively studied how AVs drive along highways. Nonetheless, how AVs navigate intersections in urban environments remains a challenging task due to the constant presence of moving road users, including turning vehicles, crossing or jaywalking pedestrians, and cyclists. AVs are thus required to learn and adapt to a dynamically evolving urban traffic environment. This paper proposes a design benchmark that allows AVs to sense the real-time traffic environment and perform path planning. The agent dynamically generates curves for feasible paths. The ego vehicle attempts to follow these paths under specific constraints. RL and MPC navigation algorithms run in parallel and are suitably selected to enhance ego vehicle safety. The ego AV is modeled with lateral and longitudinal dynamics and trained in a T-intersection using the Twin Delayed Deep Deterministic Policy Gradient (TD3) algorithm under various traffic scenarios. It is then tested on a straight road and a single or multi-lane intersections. All these experiments achieve desirable outcomes in terms of crash avoidance, driving efficiency, comfort, and tracking accuracy. The developed AV navigation system provides a design benchmark for an adaptive AV that can navigate unsignalized intersections.
更多查看译文
关键词
Urban navigation,Path planning,Reinforcement learning,Model predictive control
AI 理解论文
溯源树
样例
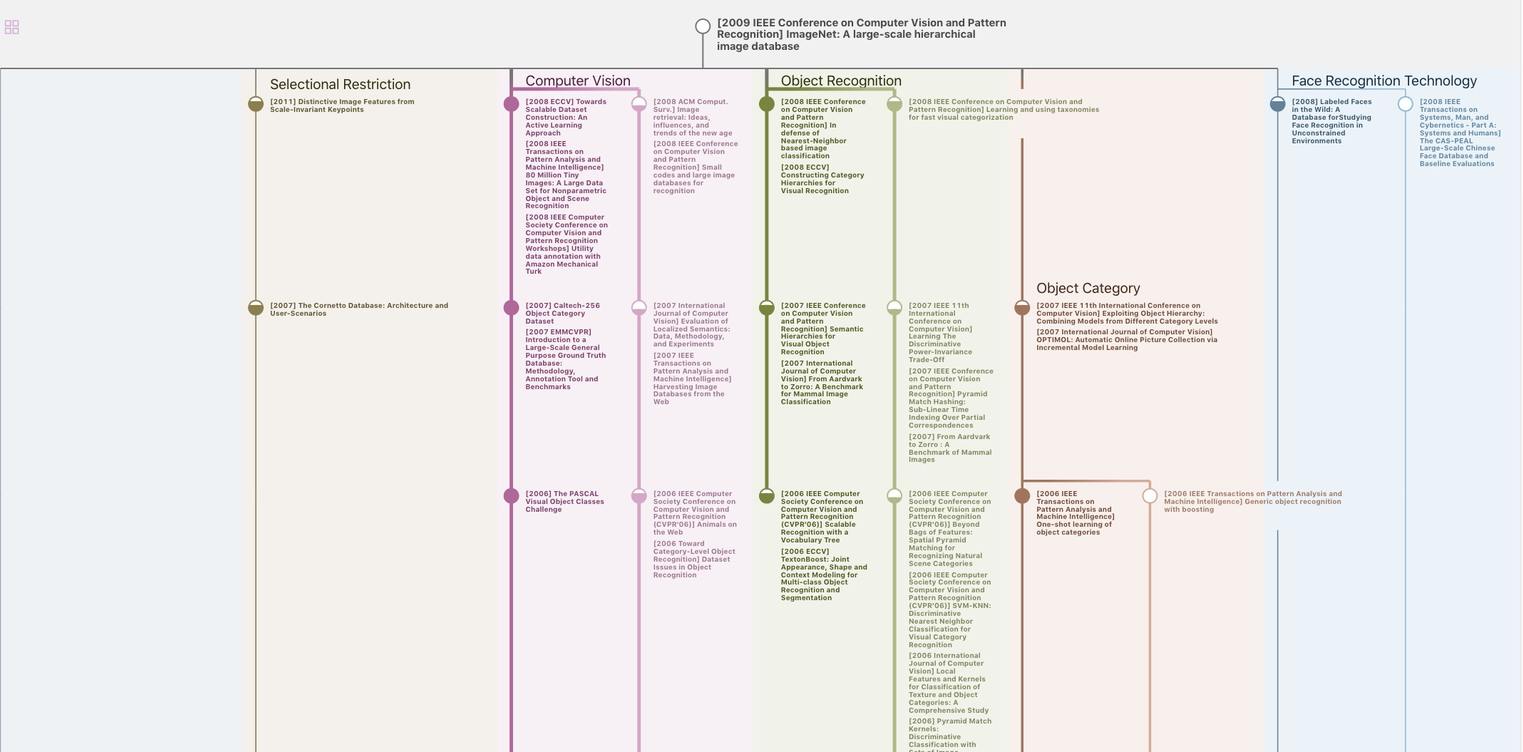
生成溯源树,研究论文发展脉络
Chat Paper
正在生成论文摘要