Feature selection algorithm based on density and distance for fault diagnosis applied to a roll-to-roll manufacturing system
JOURNAL OF COMPUTATIONAL DESIGN AND ENGINEERING(2022)
摘要
Roll-to-roll systems that include rotary components such as driven rolls and idle rollers have significant potential for application in fabrication of flexible functional devices. They are inexpensive, mass producible, and environmentally friendly; however, even minor defects in their component bearings can render them susceptible to severe damage, which necessitates accurate diagnoses of bearing quality. The main steps in machine learning for fault diagnosis include feature extraction and selection. In the case of high-dimensional feature data, critical study is required to identify the best feature combination for proper diagnosis. Thus, this study aims to develop a method that extracts fault characteristics of a bearing from the measured signal and qualify the bearing according to the Mahalanobis distances and differences in density between normal and faulty data groups. Features extracted from vibration data collected from industry-scale roll-to-roll systems and CWRU data were trained with principal component analysis, other modern feature selection techniques, and the proposed algorithm-based eight classifiers. Compared with the existing algorithm, the accuracy increased by up to 9.24%, the training time decreased by up to 34.46%, and the number of features to obtain the maximum accuracy decreased by up to 59.92%. Thus, the proposed algorithm provides an effective and time-efficient approach to improve the accuracy of fault diagnosis of rotary components.
更多查看译文
关键词
roll-to-roll systems, fault diagnosis, feature selection, rotary machines, bearings
AI 理解论文
溯源树
样例
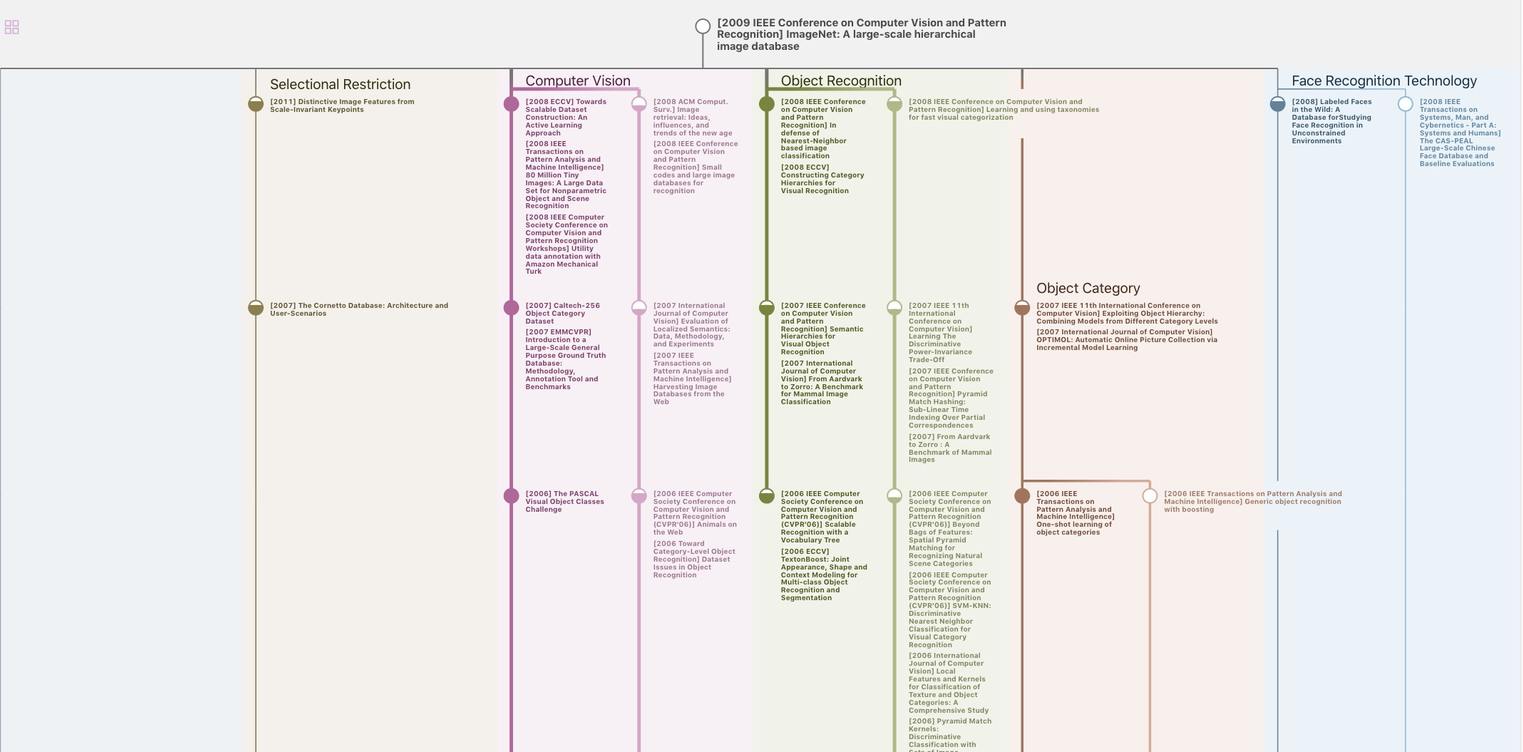
生成溯源树,研究论文发展脉络
Chat Paper
正在生成论文摘要